Analysis of Spatial-Temporal Variability of PM2.5 Concentrations Using Optical Satellite Images and Geographic Information System.
Remote. Sens.(2023)
摘要
Particulate matter less than 2.5 microns in diameter (PM2.5) is an air pollutant that has become a major environmental concern for governments around the world. Management and control require air quality monitoring and prediction. However, previous studies did not fully utilize the spectral information in multispectral satellite images and land use data in geographic datasets. To alleviate these problems, this study proposes the extraction of land use information not only from geographic inventory but also from satellite images with a machine learning-based classification. In this manner, near up-to-date land use data and spectral information from satellite images can be utilized, and the integration of geographic and remote sensing datasets boosts the accuracy of PM2.5 concentration modeling. In the experiments, Landsat-8 imagery with a 30-m spatial resolution was used, and cloud-free image generation was performed prior to the land cover classification. The proposed method, which uses predictors from geographic and multispectral satellite datasets in modeling, was compared with an approach which utilizes geographic and remote sensing datasets, respectively. Quantitative assessments showed that the proposed method and the developed model, with a performance of RMSE = 3.06 mu g/m(3) and R-2 = 0.85 comparatively outperform the models with a performance of RMSE = 3.14 mu g/m(3) and R-2 = 0.68 for remote sensing datasets and a performance of RMSE = 3.47 mu g/m(3) and R-2 = 0.79 for geographic datasets.
更多查看译文
关键词
optical satellite images,spatial–temporal variability,concentrations
AI 理解论文
溯源树
样例
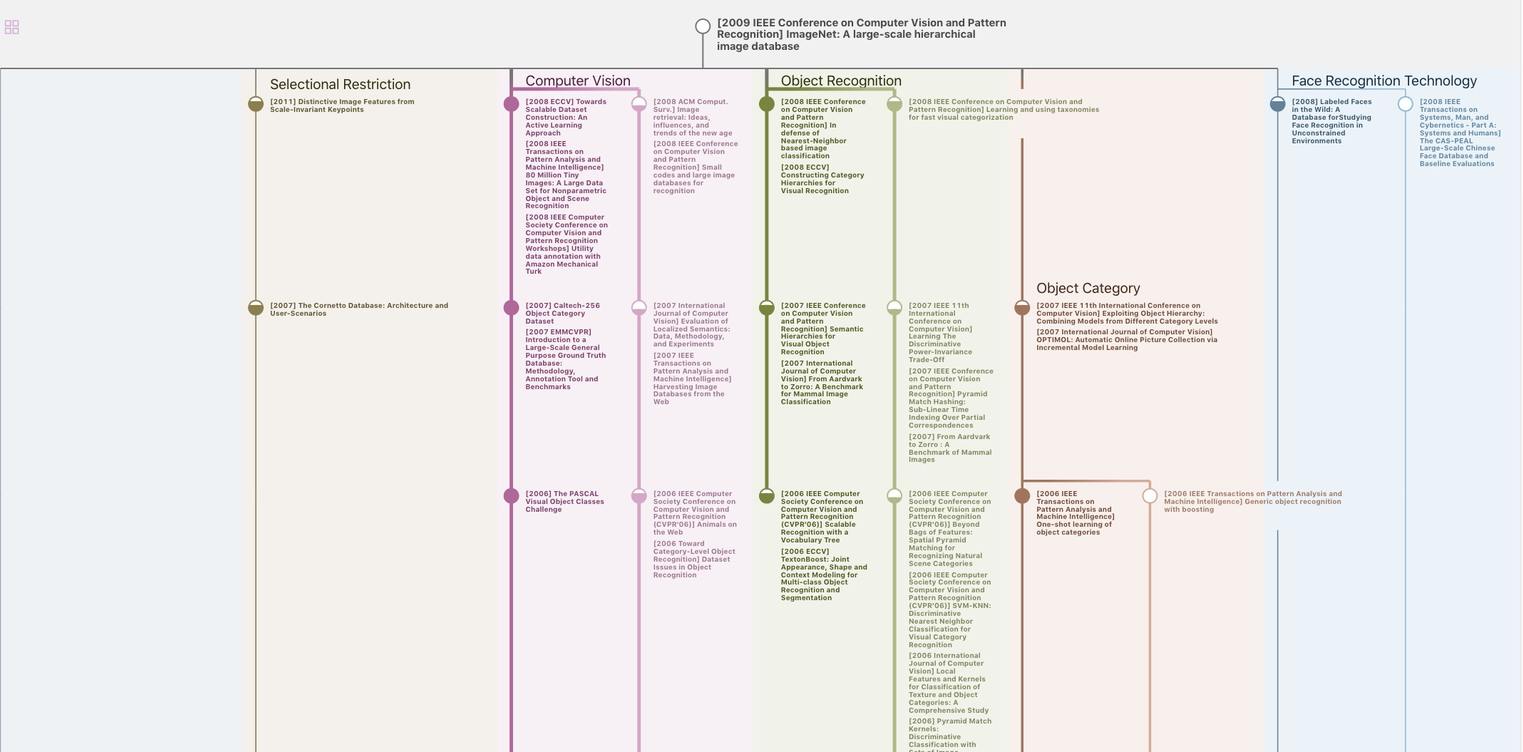
生成溯源树,研究论文发展脉络
Chat Paper
正在生成论文摘要