CCFL: Communication-Efficient Cross-Cluster Blockchain-Based Federated Learning.
WCNC(2023)
摘要
Federated Learning (FL) is a distributed learning framework that enables data sharing among multiple devices to protect data privacy. Blockchain is a decentralized ledger that can record data securely and reliably. The blockchainbased FL (BFL) framework has been used to share data and computing resources in multiple clusters. However, for the BFL framework among multi-institutional clusters, data sparsity in a cluster is a key issue. Most of the relevant works assume that the data in one cluster is rich enough to build a suitable model, which is not always satisfied in all scenarios. One method to address the problem is that enlarging the size of a BFL cluster that covers as many nodes as possible is one way. However, this method will increase communication overheads and reduce transaction throughput of the blockchain. To solve the above issues, we propose a communication-efficient blockchainbased FL framework called CCFL, which connects multiple BFL clusters to solve the data sparsity issue. We also design a pearson correlation coefficient-based dynamic model filtering mechanism that filters unnecessary models to reduce communication costs and exclude malicious models. Moreover, we illustrate a reliable contribution-based interactive validation reputation mechanism to prevent malicious nodes from participating in the training. We carry out some experiments to show the feasibility and efficiency of the proposed framework.
更多查看译文
关键词
Federated Learning,Blockchain,Model filtering,Cross-cluster,Reputation
AI 理解论文
溯源树
样例
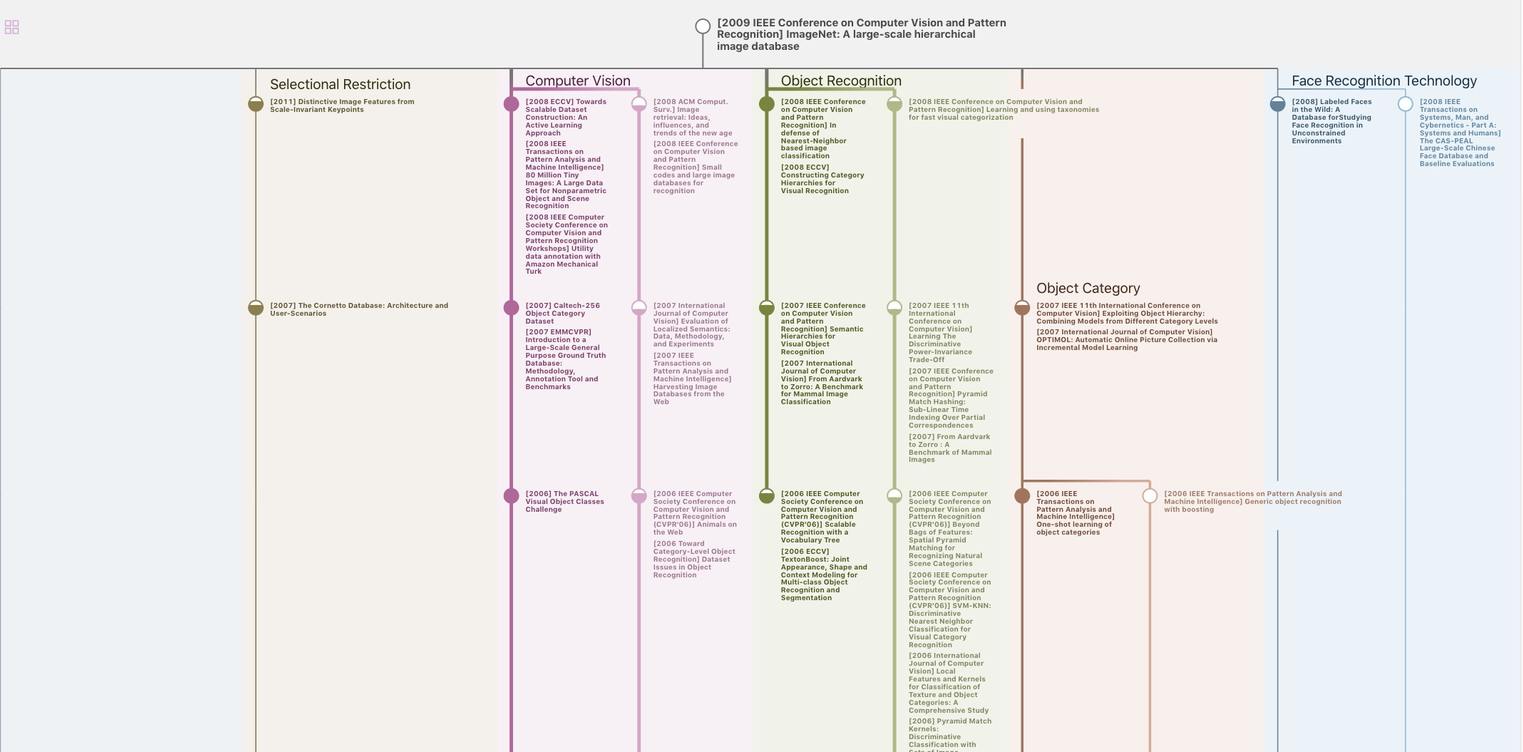
生成溯源树,研究论文发展脉络
Chat Paper
正在生成论文摘要