Deep Reinforcement Learning-based Quantization for Federated Learning.
WCNC(2023)
摘要
Federated learning (FL) is a promising solution to harness the advances of machine learning under the premise of privacy security, whereas the communication overhead of model exchange remains an obstacle to deploying FL in wireless networks. To tackle this challenge, we consider the non-uniform quantization of the global model in this work. By formulating the optimization of quantization intervals as a Markov decision process (MDP), we propose a deep reinforcement learning (DRL)-based approach to improve the performance of the quantizer for FL. Through crafting a compound reward function, the DRL agent is guided to reduce the quantization error and training loss simultaneously. Furthermore, a dual time-scale mechanism between FL and DRL is adopted to ensure that the actor and critic models of DRL converge more steadily. Simulations on various real-world datasets reveal that the proposed method can provide higher accuracy and faster convergence than the existing uniform quantizers, and can retain these benefits when applying the learned policy to a similar learning task.
更多查看译文
关键词
federated learning,quantization,reinforcement learning,model compression
AI 理解论文
溯源树
样例
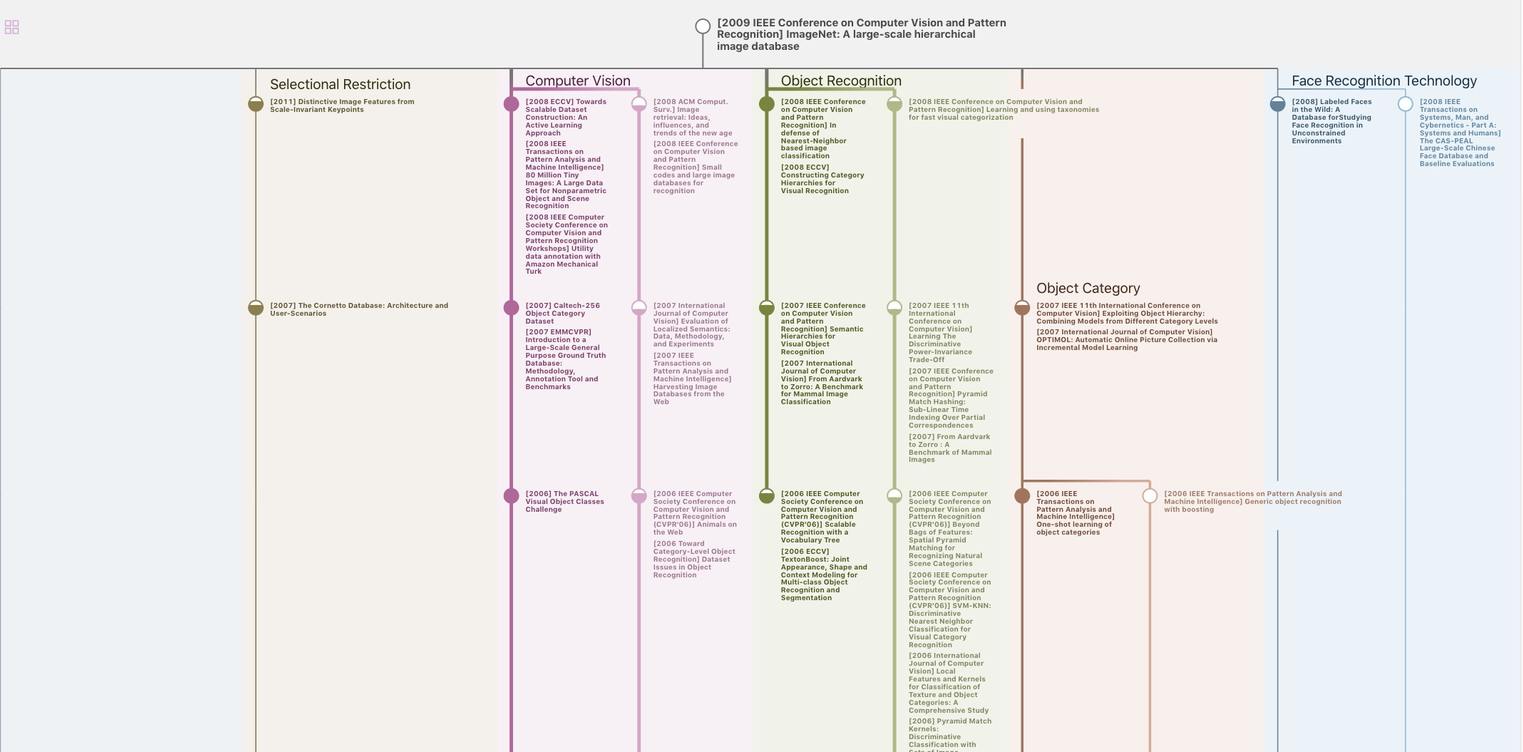
生成溯源树,研究论文发展脉络
Chat Paper
正在生成论文摘要