Nuclei Segmentation Using Cascaded Bilateral Attention U-Net.
AIPR(2022)
摘要
Medical image segmentation plays an indispensable role in biomedical development, especially in automatic disease diagnosis and treatment. The task of semantic segmentation is to group parts of an image that belong to the same object class together. In neuroscience studies, automated, accurate, and high-throughput nuclear segmentation methods are of high demand to quantify the number of cells. In this paper, we propose a cascaded U-Net network architecture with bilateral attention gate. In order to obtain nuclei edge for followed counting cell, we design the cascaded networks with different segmentation tasks. A U-Net is used as the first layer of the network to roughly segment the nucleus region, and the next layer uses a bilateral attention U-Net model with a gating mechanism to fine-tune the nucleus segmentation (including nuclei, edges, and background). The bilateral gating mechanism can learn importance of the features at different scales. Using the proposed network, experiments are conducted on a dataset of microscopic nuclear images. According to experimental results, the cascaded multi-task model with bilateral attention gate outperforms individual U-Net network and attention U-Net.
更多查看译文
AI 理解论文
溯源树
样例
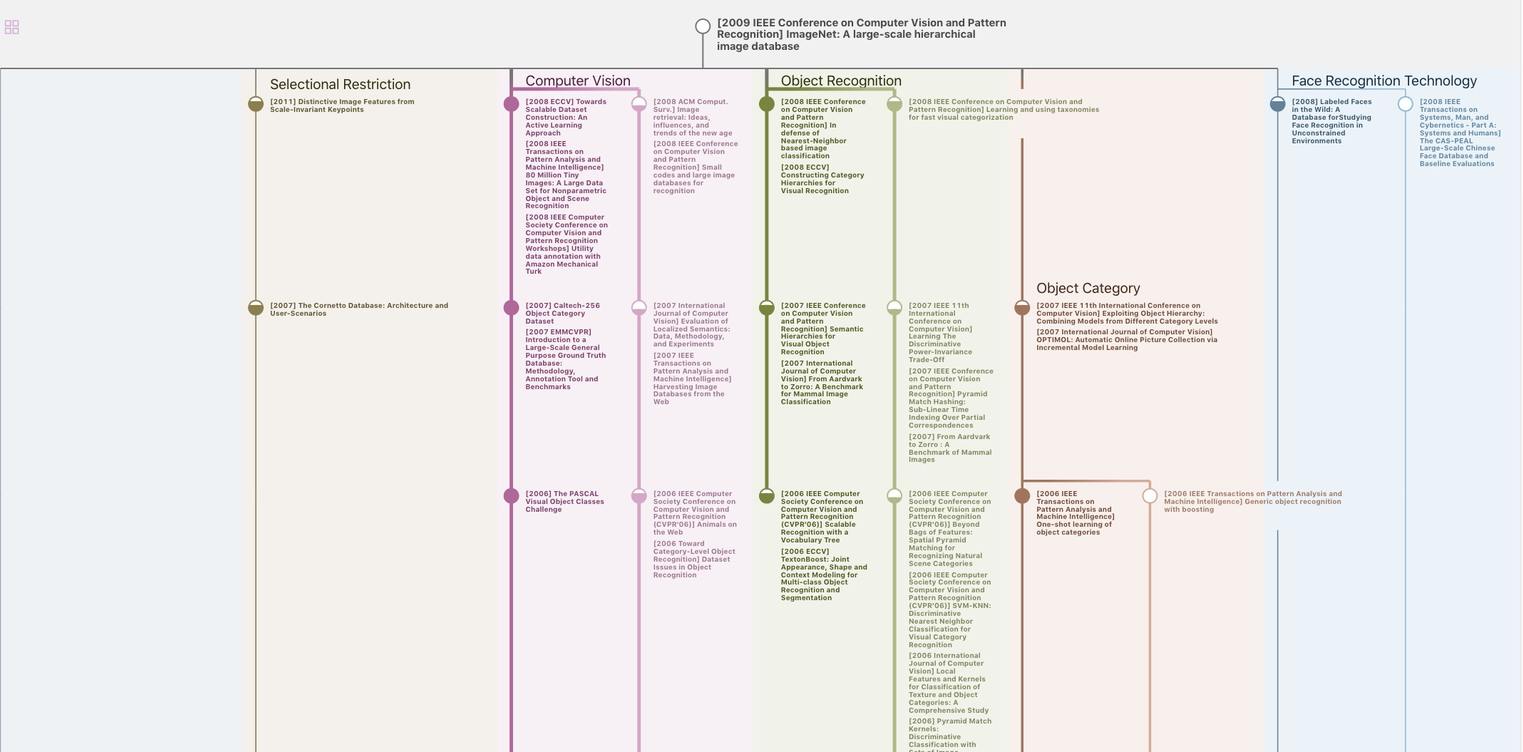
生成溯源树,研究论文发展脉络
Chat Paper
正在生成论文摘要