A time sequence coding based node-structure feature model oriented to node classification.
Expert Syst. Appl.(2023)
摘要
Graph data mining is an important method for managing complex systems in artificial intelligence (AI). As an important branch of graph data mining, node classification is widely applied in paper classification in citation networks, user classification in social networks, etc. At present, many graph neural networks (GNNs) have been proposed to realize node classification by obtaining node embeddings encoded by aggregating neighborhood features. Most GNNs use one-hot encoding as the initial node feature when there is no node attribute in the graph. However, one-hot encoding is a global encoding technique that cannot reflect the environmental context of specific nodes. To this end, we proposed a graph structure sequential coding (GSSC) model, including a learnable node-structure feature X, sampler, encoder and classifier, to obtain the structural embeddings which can better reflect the topological structure of non-attribute graphs. For non-attribute graphs, we utilized the learnable feature X as the initial node-structure feature, which could be simultaneously trained with the GSSC model. In addition, we used a decoupling scheme to separate the sampling and encoding processes of GSSC. Therefore, we could use different samplers for sparse and dense graphs. In addition, different sampling orders (i.e., temporality) in node sequences could reflect different semantic associations between the nodes. Therefore, we innovatively employed time sequence models (TSMs) as encoders to encode node sequences with temporality. Based on these TSMs, semantic information of the head node in the node sequence can be captured. The experimental results on five datasets confirmed that our GSSC model performs better than other representative methods in node classification for graphs without node attributes.
更多查看译文
关键词
classification,time sequence,feature,node-structure
AI 理解论文
溯源树
样例
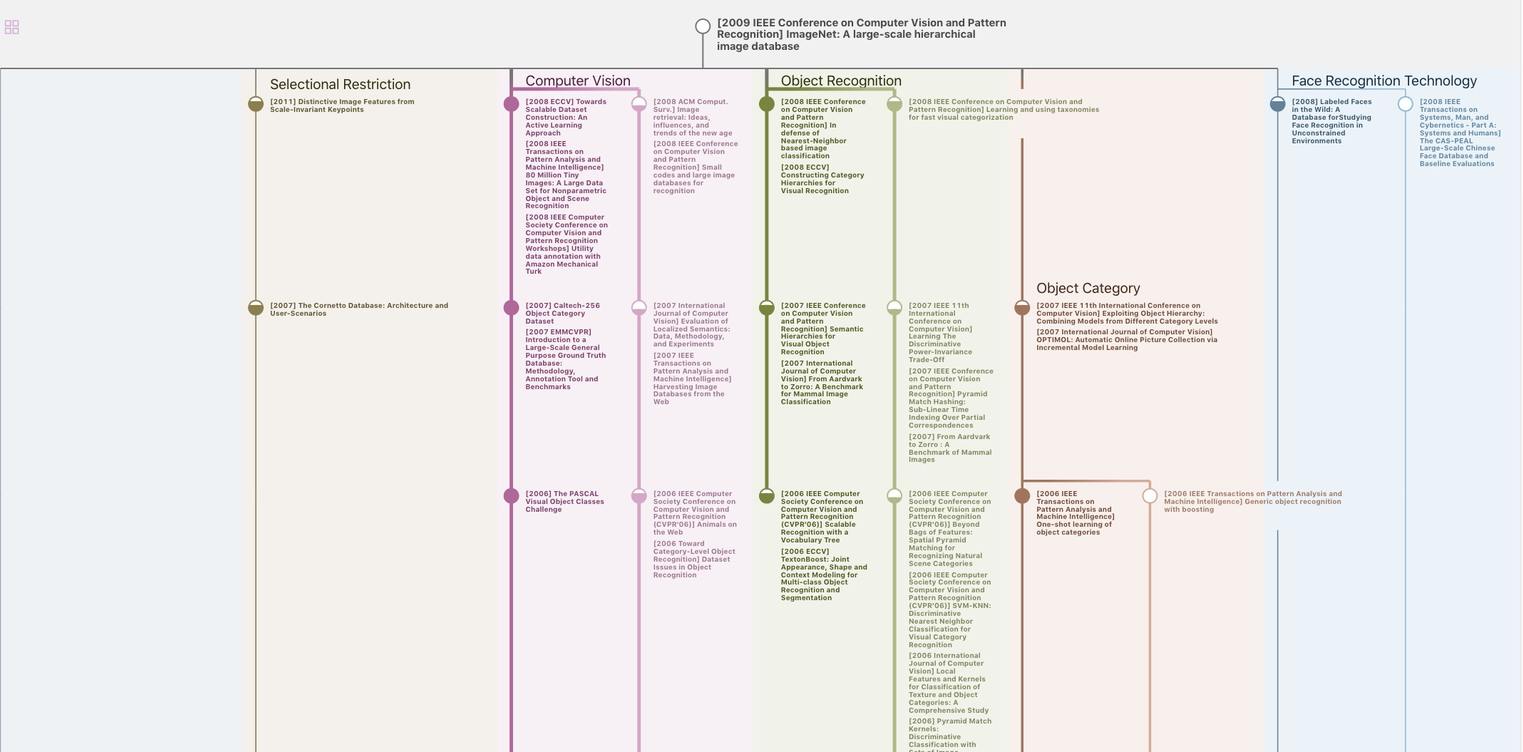
生成溯源树,研究论文发展脉络
Chat Paper
正在生成论文摘要