A novel deep policy gradient action quantization for trusted collaborative computation in intelligent vehicle networks.
Expert Syst. Appl.(2023)
摘要
The openness of the intelligent vehicle network makes it easy for selfish or untrustworthy vehicles to maliciously occupy limited resources in the mobile edge network or spread malicious information. However, most of the existing trust models rely on evaluating vehicles or data at the application level. For selfish or forgery attacks in intelligent vehicle networks, we propose a trusted deep reinforcement learning (DRL) cybersecurity approach for computation offloading to evaluate the safety and reliability performance in IoT edge networks, including our intelligent system model and a Deep Policy Gradient Action Quantization (DPGAQ) scheme. By introducing a reputation record table and designing a highly decisive communication trusted computing mode, we can accurately predict the untrusted selfish attack of vehicle in the task offloading of the Internet of things. Furthermore, in the multi-vehicle scenario, because the trusted offloading decision is a mixed integer programming problem, which leads to the dimension explosion of channel state and space, we propose a joint action-value quantization with attention mechanism to approximate the continuous actions values to a limited number of discrete values. Because it is not only inefficient but also unnecessary to generate high-dimensional decision actions in each time frame, we prune the infeasible action decisions by order preserving pruning to reduce the computational complexity of training and achieve efficient training on the premise of ensuring accuracy. To verify the feasibility and effectiveness of our proposed algorithm, millions of channels of edge vehicle networks are used as the input data. The simulation results show that compared with the benchmark trust model, DPGAQ achieves more than 72% reputation level, and improves 11%, 10% and 11% respectively in precision, recall and F-score.
更多查看译文
关键词
intelligent vehicle networks,collaborative computation
AI 理解论文
溯源树
样例
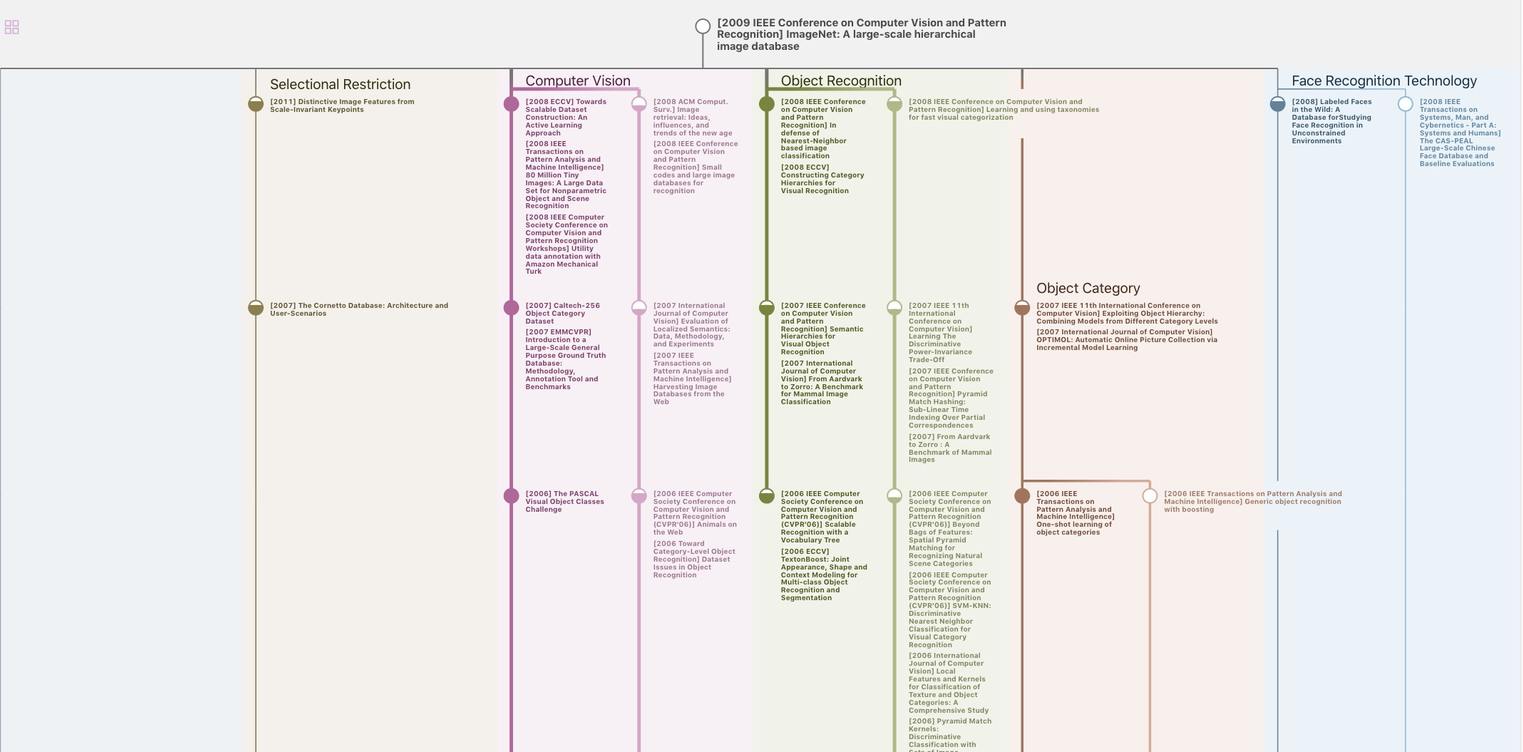
生成溯源树,研究论文发展脉络
Chat Paper
正在生成论文摘要