System model of neuromorphic sequence learning on a memristive crossbar array.
Neuromorph. Comput. Eng.(2023)
摘要
Machine learning models for sequence learning and processing often suffer from high energy consumption and require large amounts of training data. The brain presents more efficient solutions to how these types of tasks can be solved. While this has inspired the conception of novel brain-inspired algorithms, their realizations remain constrained to conventional von-Neumann machines. Therefore, the potential power efficiency of the algorithm cannot be exploited due to the inherent memory bottleneck of the computing architecture. Therefore, we present in this paper a dedicated hardware implementation of a biologically plausible version of the Temporal Memory component of the Hierarchical Temporal Memory concept. Our implementation is built on a memristive crossbar array and is the result of a hardware-algorithm co-design process. Rather than using the memristive devices solely for data storage, our approach leverages their specific switching dynamics to propose a formulation of the peripheral circuitry, resulting in a more efficient design. By combining a brain-like algorithm with emerging non-volatile memristive device technology we strive for maximum energy efficiency. We present simulation results on the training of complex high-order sequences and discuss how the system is able to predict in a context-dependent manner. Finally, we investigate the energy consumption during the training and conclude with a discussion of scaling prospects.
更多查看译文
关键词
neuromorphic sequence
AI 理解论文
溯源树
样例
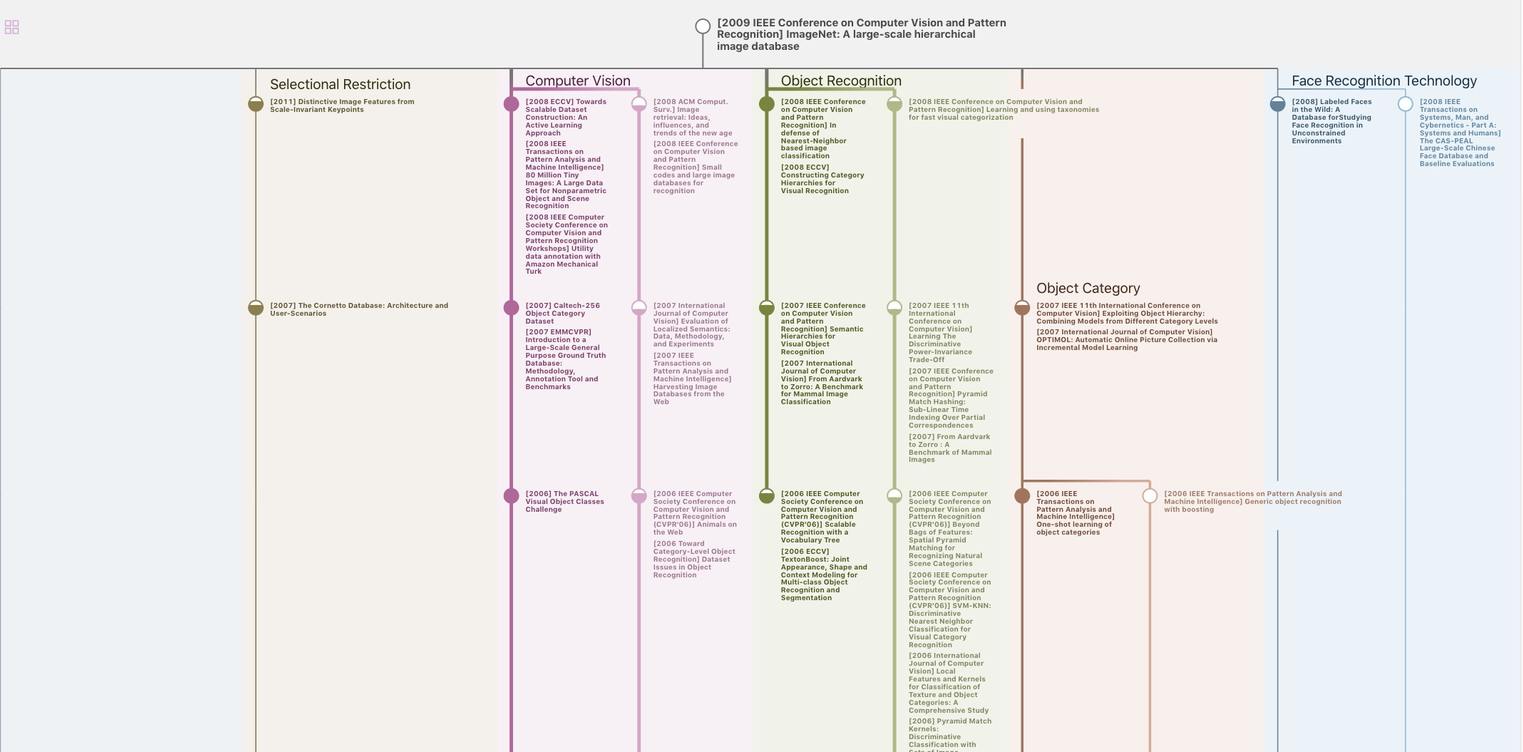
生成溯源树,研究论文发展脉络
Chat Paper
正在生成论文摘要