Optimal Deep Learning Based Intruder Identification in Industrial Internet of Things Environment.
Comput. Syst. Sci. Eng.(2023)
摘要
With the increased advancements of smart industries, cybersecurity has become a vital growth factor in the success of industrial transformation. The Industrial Internet of Things (IIoT) or Industry 4.0 has revolutionized the concepts of manufacturing and production altogether. In industry 4.0, powerful Intrusion Detection Systems (IDS) play a significant role in ensuring network security. Though various intrusion detection techniques have been developed so far, it is challenging to protect the intricate data of networks. This is because conventional Machine Learning (ML) approaches are inadequate and insufficient to address the demands of dynamic IIoT networks. Further, the existing Deep Learning (DL) can be employed to identify anonymous intrusions. Therefore, the current study proposes a Hunger Games Search Optimization with Deep Learning-Driven Intrusion Detection (HGSODL-ID) model for the IIoT environment. The presented HGSODL-ID model exploits the linear normalization approach to transform the input data into a useful format. The HGSO algorithm is employed for Feature Selection (HGSO-FS) to reduce the curse of dimensionality. Moreover, Sparrow Search Optimization (SSO) is utilized with a Graph Convolutional Network (GCN) to classify and identify intrusions in the network. Finally, the SSO technique is exploited to fine-tune the hyper-parameters involved in the GCN model. The proposed HGSODL-ID model was experimentally validated using a benchmark dataset, and the results confirmed the superiority of the proposed HGSODL-ID method over recent approaches.
更多查看译文
关键词
deep learning,industrial internet,things environment,identification
AI 理解论文
溯源树
样例
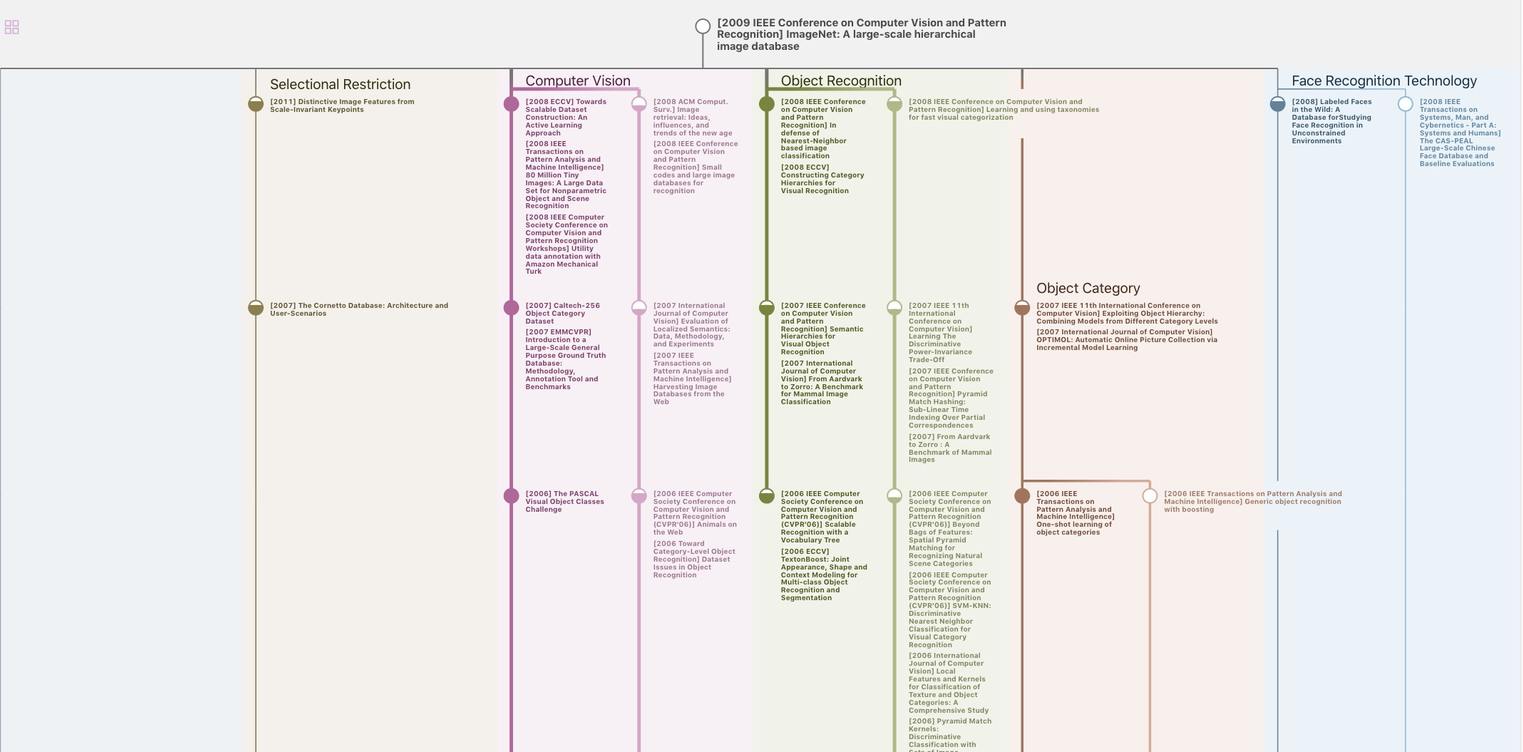
生成溯源树,研究论文发展脉络
Chat Paper
正在生成论文摘要