A Physics-Assisted Online Learning Method for Tool Wear Prediction.
IEEE Trans. Instrum. Meas.(2023)
摘要
Tool wear prediction is critical in machining because worn tools lead to poor precision and quality in production. Conventional tool wear prediction methods include physics-driven methods, which are typically independent of real-time working conditions, and data-driven methods, which are limited by the amount of training data. To address these issues, this article proposes a physics-assisted online learning method for tool wear prediction. The novelties of this method are that it not only utilizes knowledge from data and physical domain but also adjusts the offline basic model with online data. In this method, features extracted by the stacked sparsity autoencoder (SSAE) are combined with measured and physics-generated labels as the training set. A multilayer perceptron (MLP) is trained to get physical model coefficients via working parameters to get pseudo labels under testing conditions. Then, partial and global MLPs are integrated as the basic prediction model. Finally, pseudo labels and real-time signals are treated as the online updating set for basic model calibration. It is found that the proposed method has the lowest mean square error (MSE) 42.0069 and time complexity. The repeated experiment is done to verify this method further. These results show the good accuracy and generalization of this method.
更多查看译文
关键词
online learning method,online learning,tool,prediction,physics-assisted
AI 理解论文
溯源树
样例
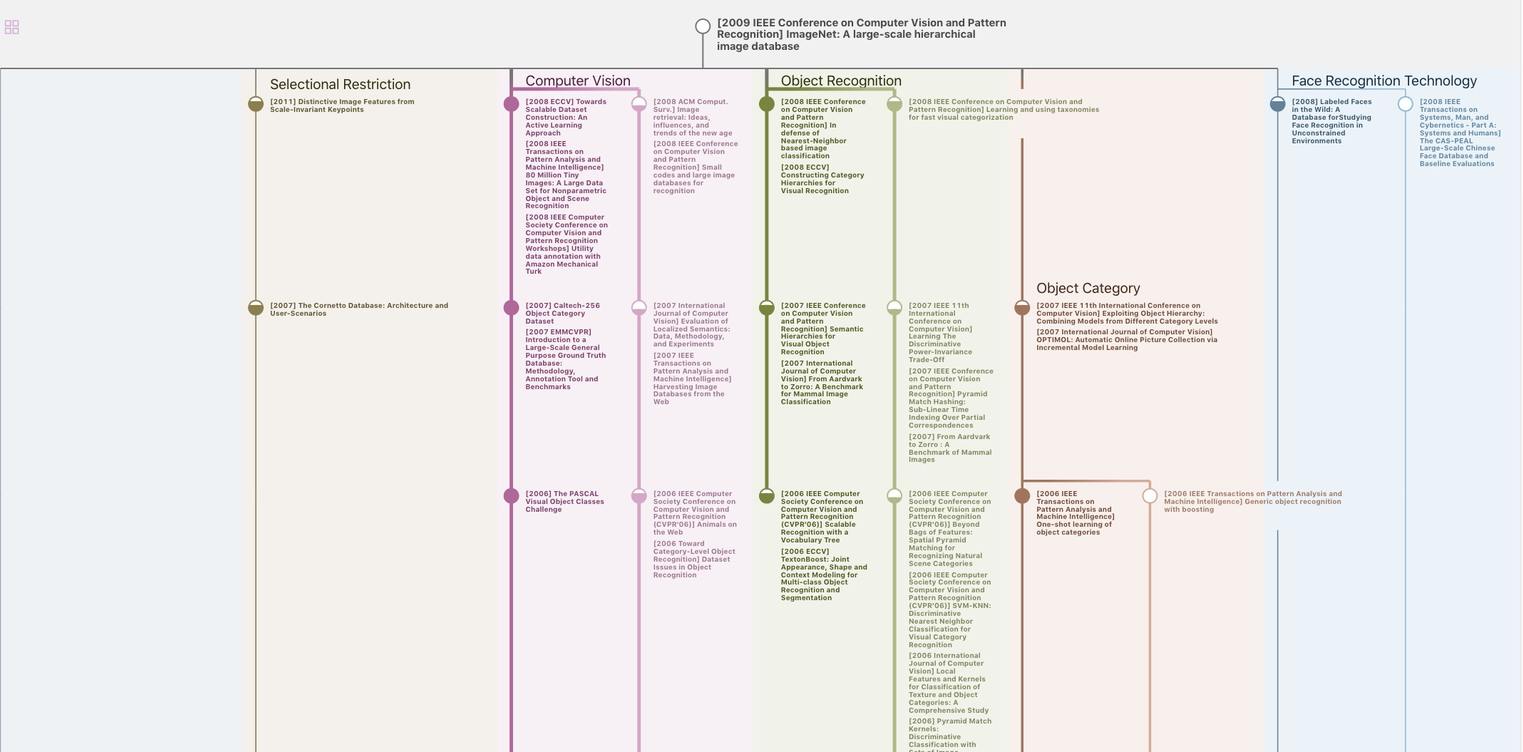
生成溯源树,研究论文发展脉络
Chat Paper
正在生成论文摘要