Quantitative Assessment Framework for Non-Structural Bird's Nest Risk Information of Transmission Tower in High-Resolution UAV Images.
IEEE Trans. Instrum. Meas.(2023)
Abstract
As the main cause of foreign body intrusion, bird's nests pose a significant potential risk to high-voltage transmission towers. Currently, most research uses convolutional neural networks (CNNs) to detect partial images of towers captured by manually operated unmanned aerial vehicles (UAVs). However, these methods have difficulties in learning the locations and potential risk information of bird's nests and generating structured data results. To address these challenges, this article presents a quantitative assessment framework for non-structural bird's nest risk information of transmission towers using high-resolution UAV panoramic images. The integrity of the tower's structure information is maintained by using panoramic UAV images. The proposed method involves estimating the tower's pose and determining whether each key point of the tower has a bird's nest, transforming the non-structural bird's nest locations and risk information into structured data. A risk score is used to classify the level of potential risk caused by bird's nests. The experiments demonstrate that the proposed framework can accurately detect the key points of five types of towers, with an $F1$ -score of 83.09% for bird's nest detection and an accuracy of 91.59% for risk assessment.
MoreTranslated text
Key words
Bird's nest detection,convolutional neural network (CNN),high-resolution net (HRNet),risk assessment,simple baseline,tower pose estimation,unmanned aerial vehicles (UAVs)
AI Read Science
Must-Reading Tree
Example
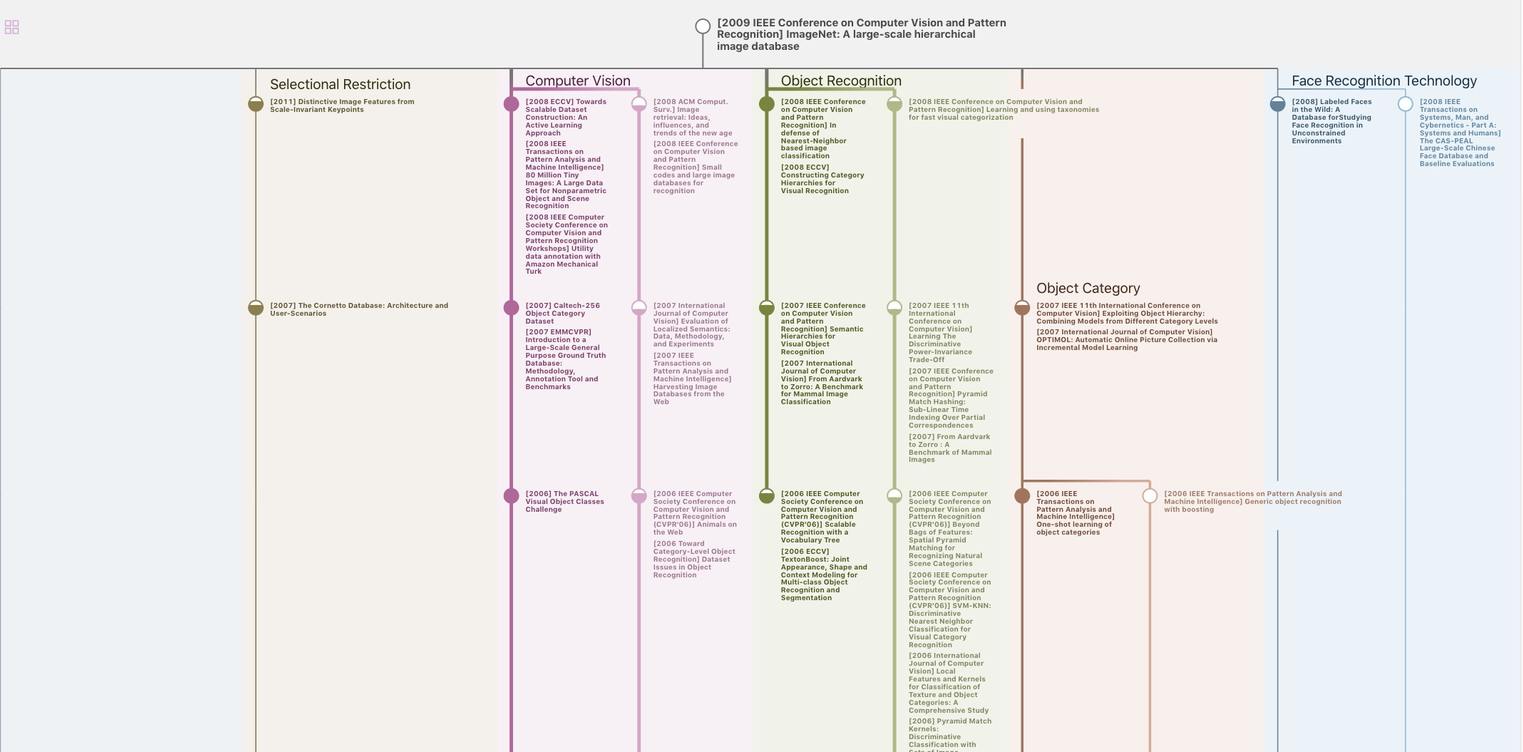
Generate MRT to find the research sequence of this paper
Chat Paper
Summary is being generated by the instructions you defined