ViCo: Detail-Preserving Visual Condition for Personalized Text-to-Image Generation
CoRR(2023)
摘要
Personalized text-to-image generation using diffusion models has recently been proposed and attracted lots of attention. Given a handful of images containing a novel concept (e.g., a unique toy), we aim to tune the generative model to capture fine visual details of the novel concept and generate photorealistic images following a text condition. We present a plug-in method, named ViCo, for fast and lightweight personalized generation. Specifically, we propose an image attention module to condition the diffusion process on the patch-wise visual semantics. We introduce an attention-based object mask that comes almost at no cost from the attention module. In addition, we design a simple regularization based on the intrinsic properties of text-image attention maps to alleviate the common overfitting degradation. Unlike many existing models, our method does not finetune any parameters of the original diffusion model. This allows more flexible and transferable model deployment. With only light parameter training (~6% of the diffusion U-Net), our method achieves comparable or even better performance than all state-of-the-art models both qualitatively and quantitatively.
更多查看译文
关键词
visual,generation,detail-preserving,text-to-image
AI 理解论文
溯源树
样例
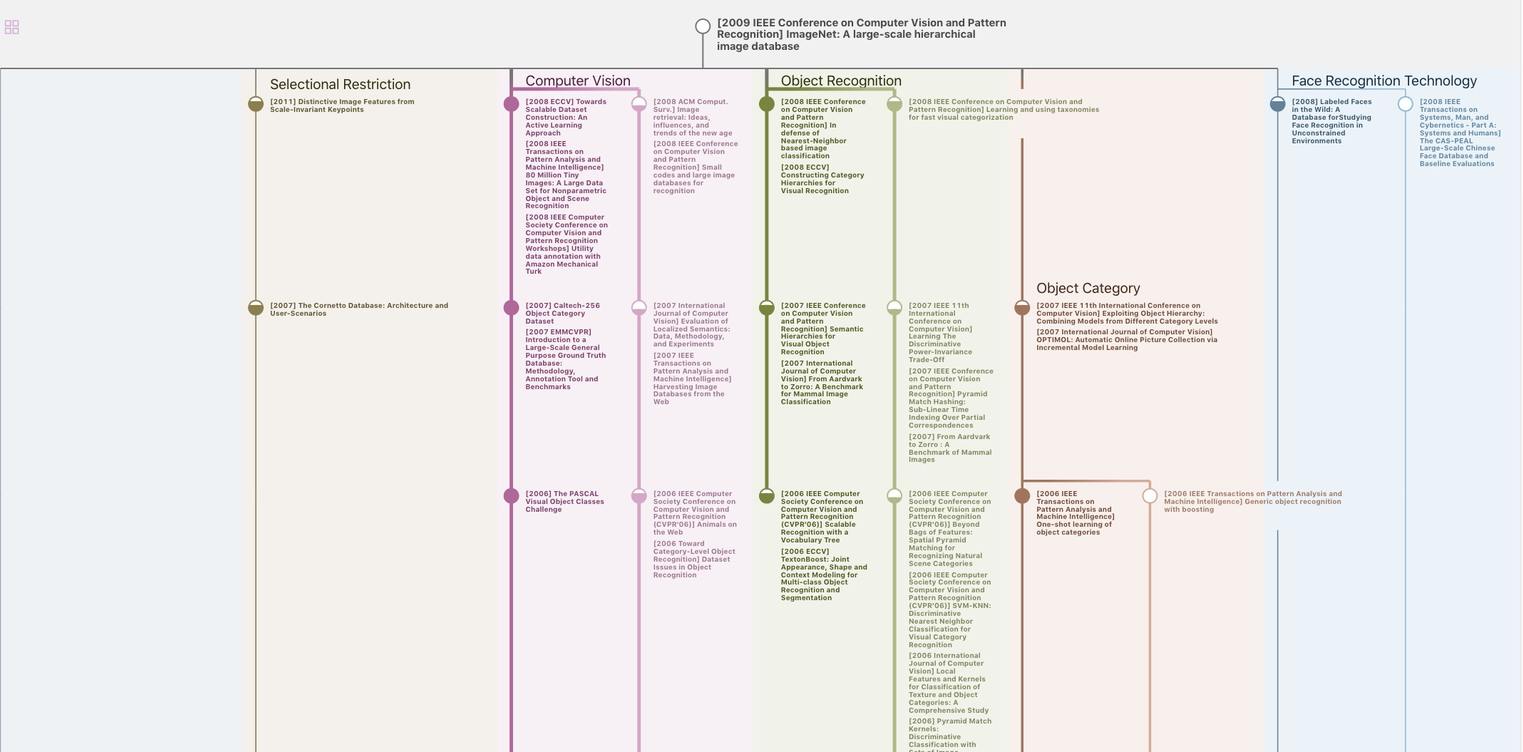
生成溯源树,研究论文发展脉络
Chat Paper
正在生成论文摘要