AMR4NLI: Interpretable and robust NLI measures from semantic graphs
CoRR(2023)
Abstract
The task of natural language inference (NLI) asks whether a given premise (expressed in NL) entails a given NL hypothesis. NLI benchmarks contain human ratings of entailment, but the meaning relationships driving these ratings are not formalized. Can the underlying sentence pair relationships be made more explicit in an interpretable yet robust fashion? We compare semantic structures to represent premise and hypothesis, including sets of contextualized embeddings and semantic graphs (Abstract Meaning Representations), and measure whether the hypothesis is a semantic substructure of the premise, utilizing interpretable metrics. Our evaluation on three English benchmarks finds value in both contextualized embeddings and semantic graphs; moreover, they provide complementary signals, and can be leveraged together in a hybrid model.
MoreTranslated text
Key words
robust amr4nli measures,graphs
AI Read Science
Must-Reading Tree
Example
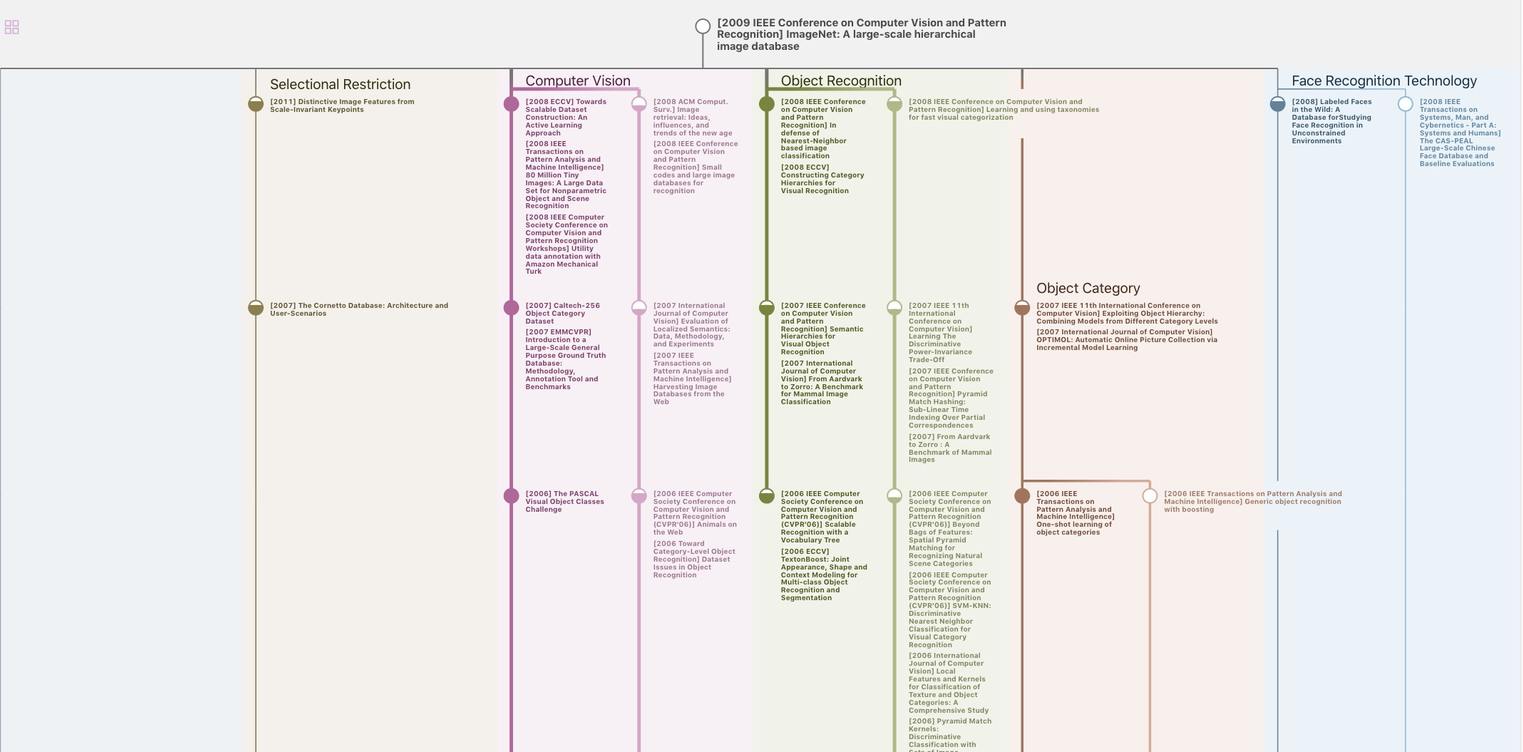
Generate MRT to find the research sequence of this paper
Chat Paper
Summary is being generated by the instructions you defined