Learning Disentangled Prompts for Compositional Image Synthesis
CoRR(2023)
摘要
We study domain-adaptive image synthesis, the problem of teaching pretrained image generative models a new style or concept from as few as one image to synthesize novel images, to better understand the compositional image synthesis. We present a framework that leverages a pretrained class-conditional generation model and visual prompt tuning. Specifically, we propose a novel source class distilled visual prompt that learns disentangled prompts of semantic (e.g., class) and domain (e.g., style) from a few images. Learned domain prompt is then used to synthesize images of any classes in the style of target domain. We conduct studies on various target domains with the number of images ranging from one to a few to many, and show qualitative results which show the compositional generalization of our method. Moreover, we show that our method can help improve zero-shot domain adaptation classification accuracy.
更多查看译文
关键词
compositional image synthesis,image synthesis,learning,prompts
AI 理解论文
溯源树
样例
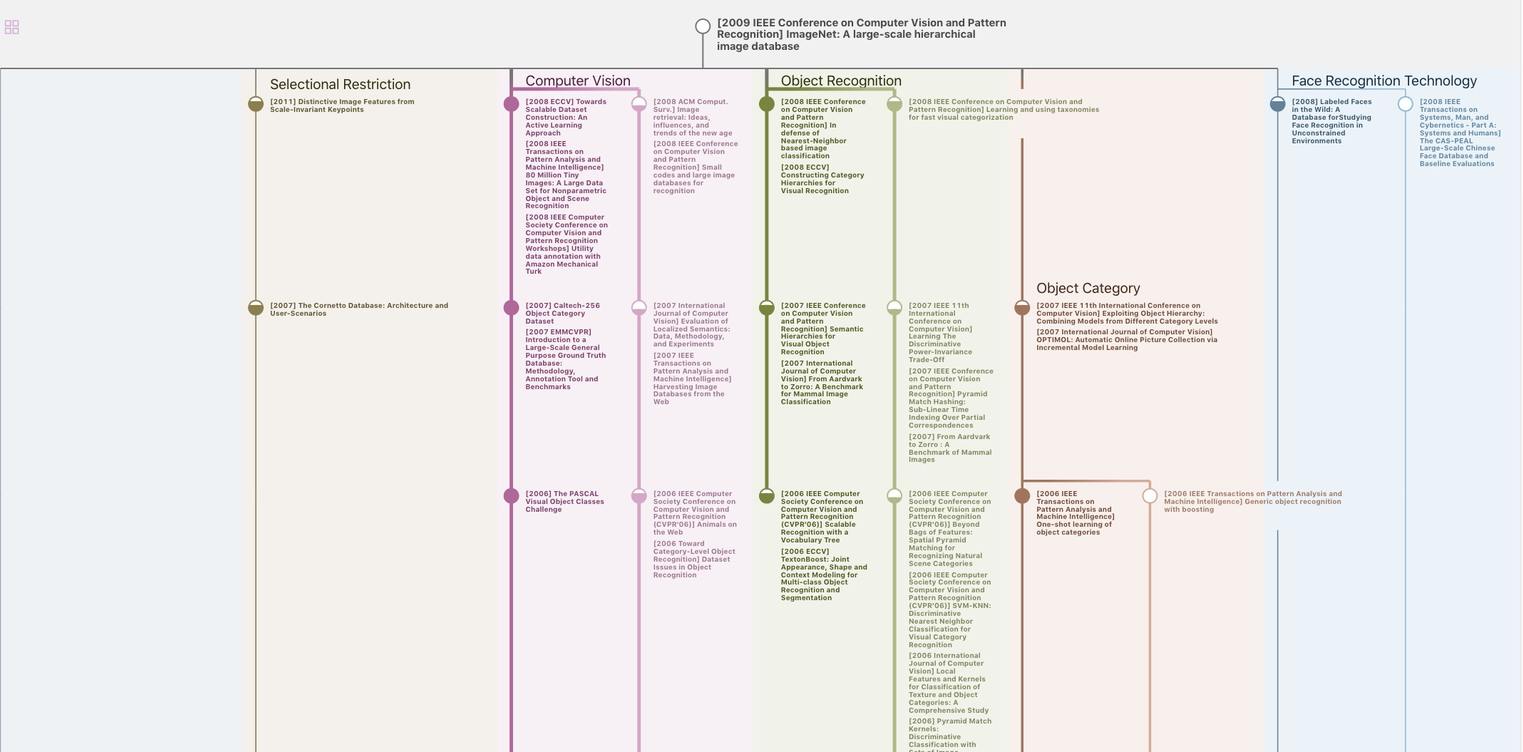
生成溯源树,研究论文发展脉络
Chat Paper
正在生成论文摘要