Fast transport simulations with higher-fidelity surrogate models for ITER
PHYSICS OF PLASMAS(2023)
摘要
A fast and accurate turbulence transport model based on quasilinear gyrokinetics is developed. The model consists of a set of neural networks trained on a bespoke quasilinear GENE dataset, with a saturation rule calibrated to dedicated nonlinear simulations. The resultant neural network is approximately eight orders of magnitude faster than the original GENE quasilinear calculations. ITER predictions with the new model project a fusion gain in line with ITER targets. While the dataset is currently limited to the ITER baseline regime, this approach illustrates a pathway to develop reduced-order turbulence models both faster and more accurate than the current state-of-the-art.
更多查看译文
关键词
fast transport simulations,surrogate models,higher-fidelity
AI 理解论文
溯源树
样例
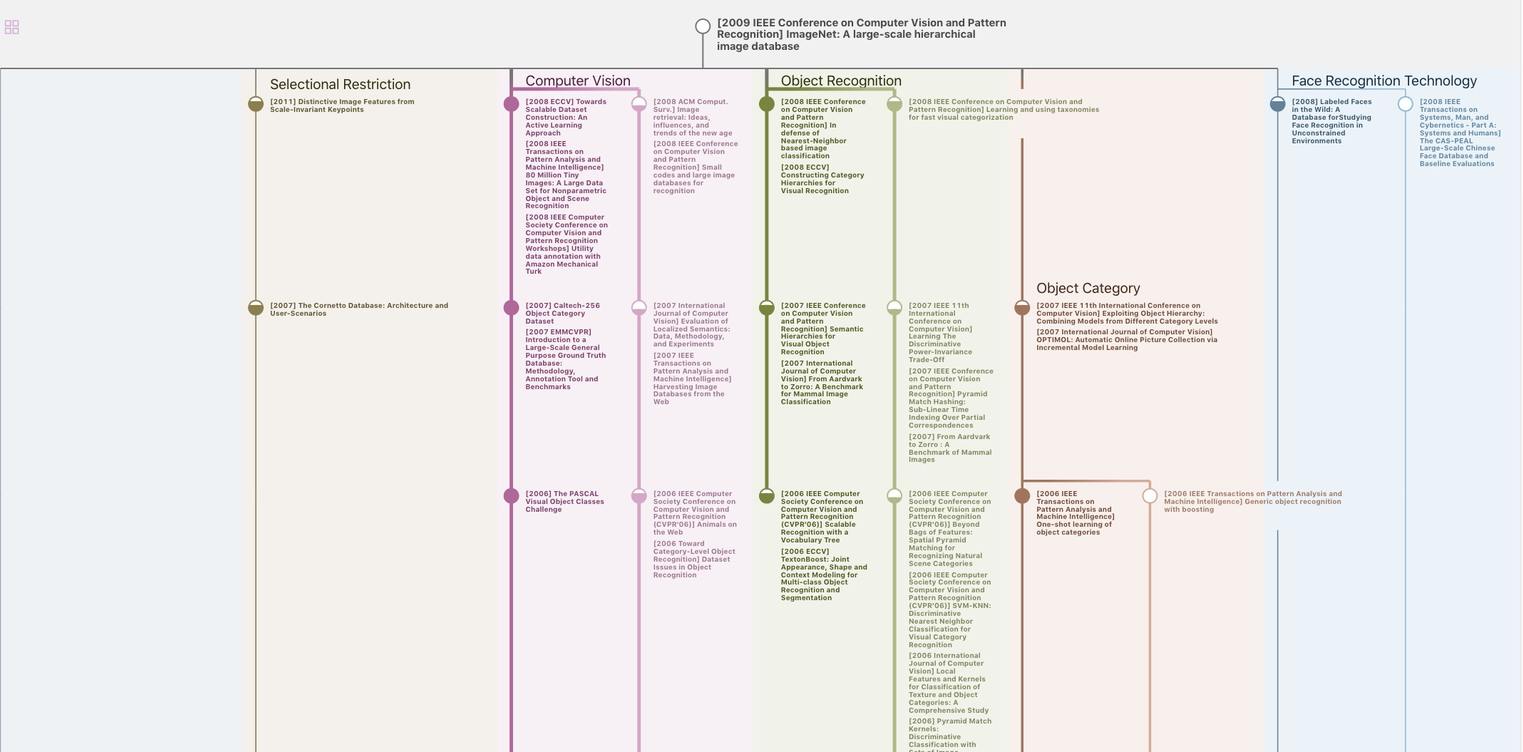
生成溯源树,研究论文发展脉络
Chat Paper
正在生成论文摘要