Universal Test-time Adaptation through Weight Ensembling, Diversity Weighting, and Prior Correction
2024 IEEE/CVF Winter Conference on Applications of Computer Vision (WACV)(2023)
摘要
Since distribution shifts are likely to occur during test-time and can drastically decrease the model's performance, online test-time adaptation (TTA) continues to update the model after deployment, leveraging the current test data. Clearly, a method proposed for online TTA has to perform well for all kinds of environmental conditions. By introducing the variable factors 'domain non-stationarity' and 'temporal correlation', we first unfold all practically relevant settings and define the entity as universal TTA. To tackle the problem of universal TTA, we identify and highlight several challenges a self-training based method has to deal with, including: 1) model bias and the occurrence of trivial solutions when performing entropy minimization on varying sequence lengths with and without multiple domain shifts, 2) loss of generalization which exacerbates the adaptation to future domain shifts and the occurrence of catastrophic forgetting, and 3) performance degradation due to shifts in label prior. To prevent the model from becoming biased, we leverage a dataset and model-agnostic certainty and diversity weighting. In order to maintain generalization and prevent catastrophic forgetting, we propose to continually weight-average the source and adapted model. To compensate for disparities in the label prior during test-time, we propose an adaptive additive prior correction scheme. We evaluate our approach, named ROID, on a wide range of settings, datasets, and models, setting new standards in the field of universal TTA.
更多查看译文
关键词
Algorithms,Machine learning architectures,formulations,and algorithms
AI 理解论文
溯源树
样例
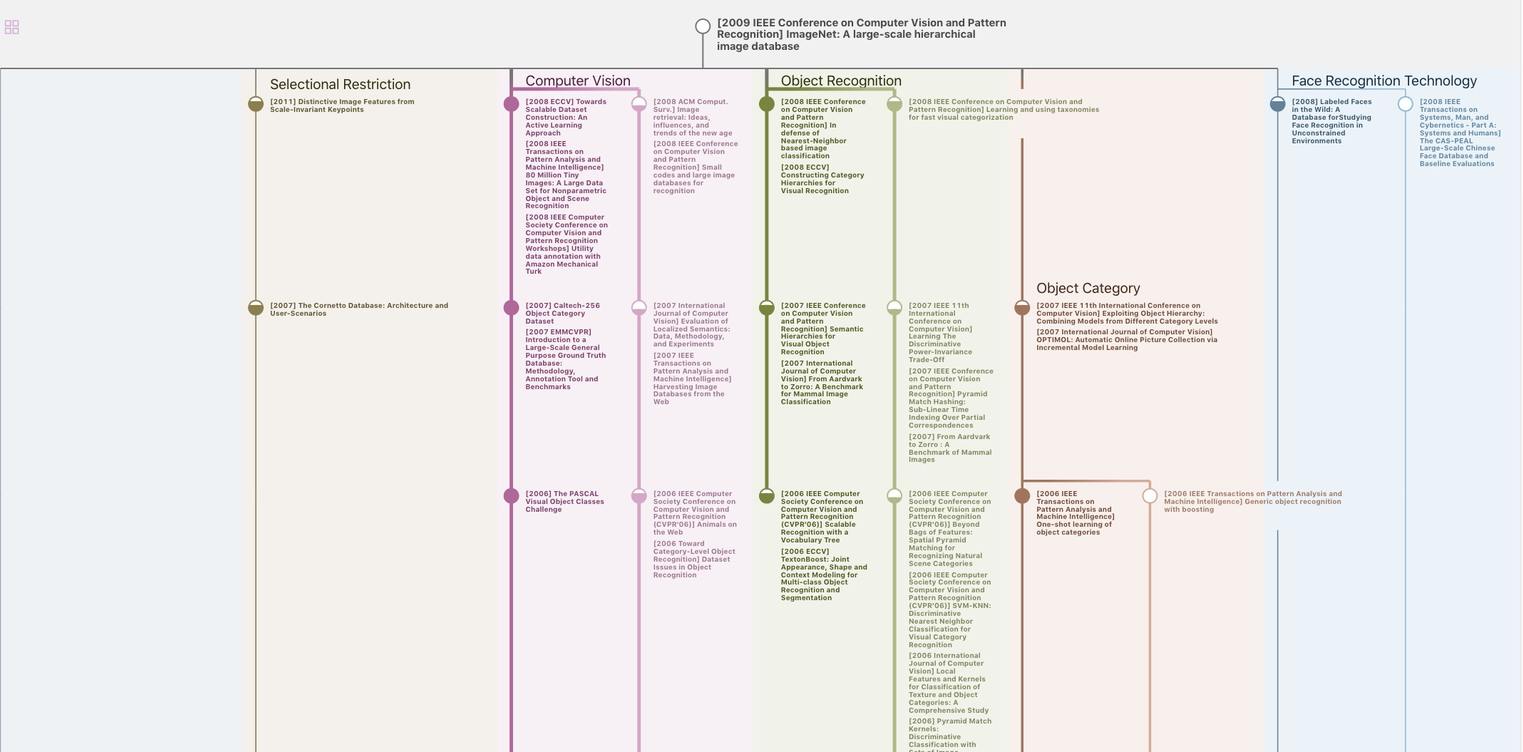
生成溯源树,研究论文发展脉络
Chat Paper
正在生成论文摘要