Algorithm Instance Footprint: Separating Easily Solvable and Challenging Problem Instances
CoRR(2023)
摘要
In black-box optimization, it is essential to understand why an algorithm instance works on a set of problem instances while failing on others and provide explanations of its behavior. We propose a methodology for formulating an algorithm instance footprint that consists of a set of problem instances that are easy to be solved and a set of problem instances that are difficult to be solved, for an algorithm instance. This behavior of the algorithm instance is further linked to the landscape properties of the problem instances to provide explanations of which properties make some problem instances easy or challenging. The proposed methodology uses meta-representations that embed the landscape properties of the problem instances and the performance of the algorithm into the same vector space. These meta-representations are obtained by training a supervised machine learning regression model for algorithm performance prediction and applying model explainability techniques to assess the importance of the landscape features to the performance predictions. Next, deterministic clustering of the meta-representations demonstrates that using them captures algorithm performance across the space and detects regions of poor and good algorithm performance, together with an explanation of which landscape properties are leading to it.
更多查看译文
关键词
algorithm behavior,single-objective optimization,latent representations,supervised machine learning,explainability
AI 理解论文
溯源树
样例
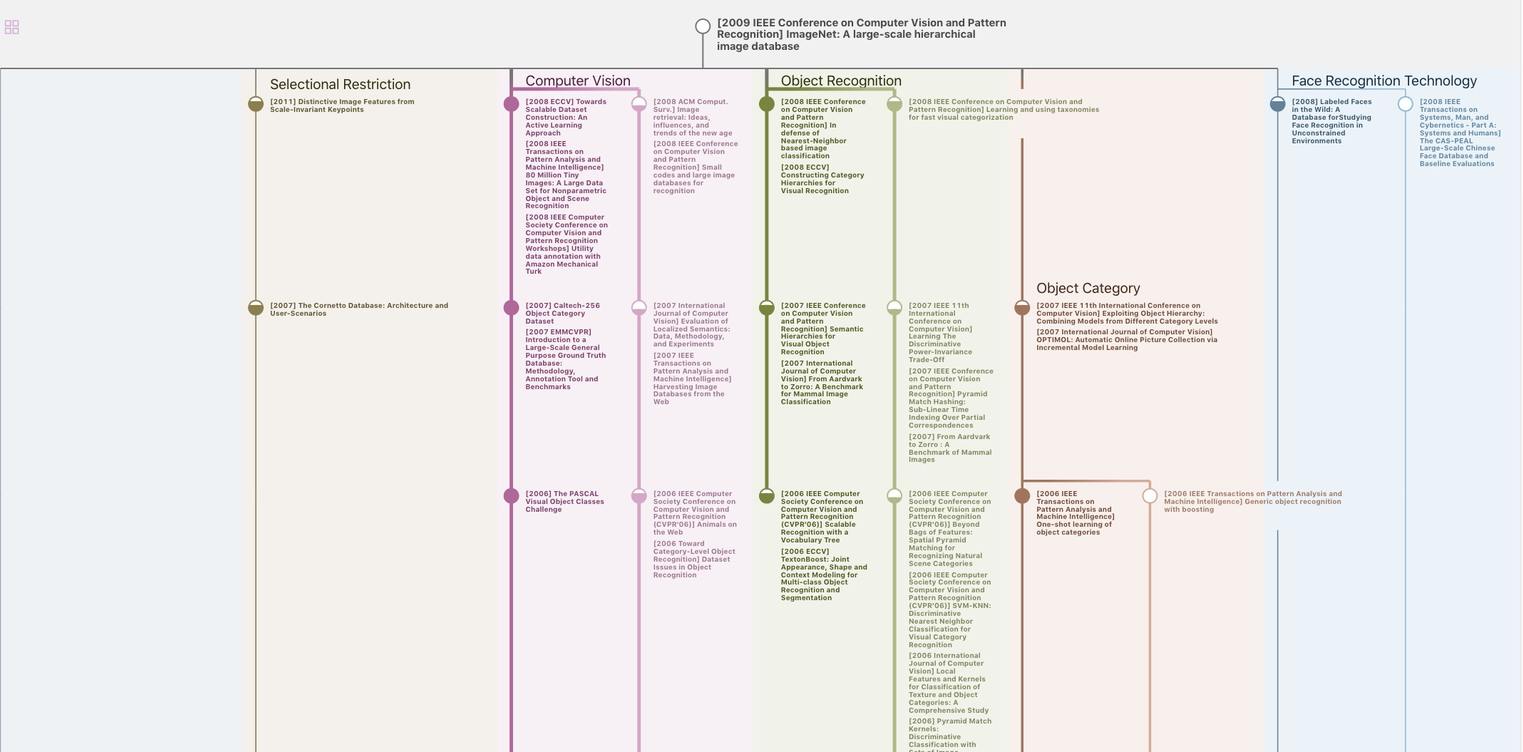
生成溯源树,研究论文发展脉络
Chat Paper
正在生成论文摘要