Graph Exploration Matters: Improving both Individual-Level and System-Level Diversity in WeChat Feed Recommendation
PROCEEDINGS OF THE 32ND ACM INTERNATIONAL CONFERENCE ON INFORMATION AND KNOWLEDGE MANAGEMENT, CIKM 2023(2023)
摘要
There are roughly three stages in real industrial recommendation systems, candidates generation (retrieval), ranking and reranking. Both individual-level diversity and system-level diversity are important in this framework. The former focus on each single user's experience, while the latter focus on the difference among users. Graph-based retrieval strategies are inevitably hijacked by heavy users and popular items, leading to similar results for each user and the convergence among users. In the reranking phase, Determinantal Point Process (DPP) is widely deployed to increase individual-level diversity. Heavily relying on the semantic information of items, DPP suffers from clickbait and inaccurate categories. Besides, most studies only focus on one of the two levels of diversity, and ignore the mutual influence among different stages in real recommender systems. We argue that individual-level diversity and system-level diversity should be viewed as an integrated problem, and we provide an efficient and deployable solution for web-scale recommenders. Generally, we propose to employ the retrieval graph information in diversity-based reranking, by which to weaken the hidden similarity of items exposed to users, and consequently gain more graph explorations to improve the system-level diversity. Besides, we argue that users' propensity for diversity changes over time in content feed recommendation. Therefore, with the explored graph, we also propose to capture the user's real-time personalized propensity to the diversity. We implement and deploy the combined system in WeChat App's Top Stories used by hundreds of millions of users. Comparative and long-term online A/B tests show our solution can effectively improve both user engagement and system revenue.
更多查看译文
关键词
Diversity,Recommendation System,Retrieval,Reranking,GNN,Determinantal Point Process
AI 理解论文
溯源树
样例
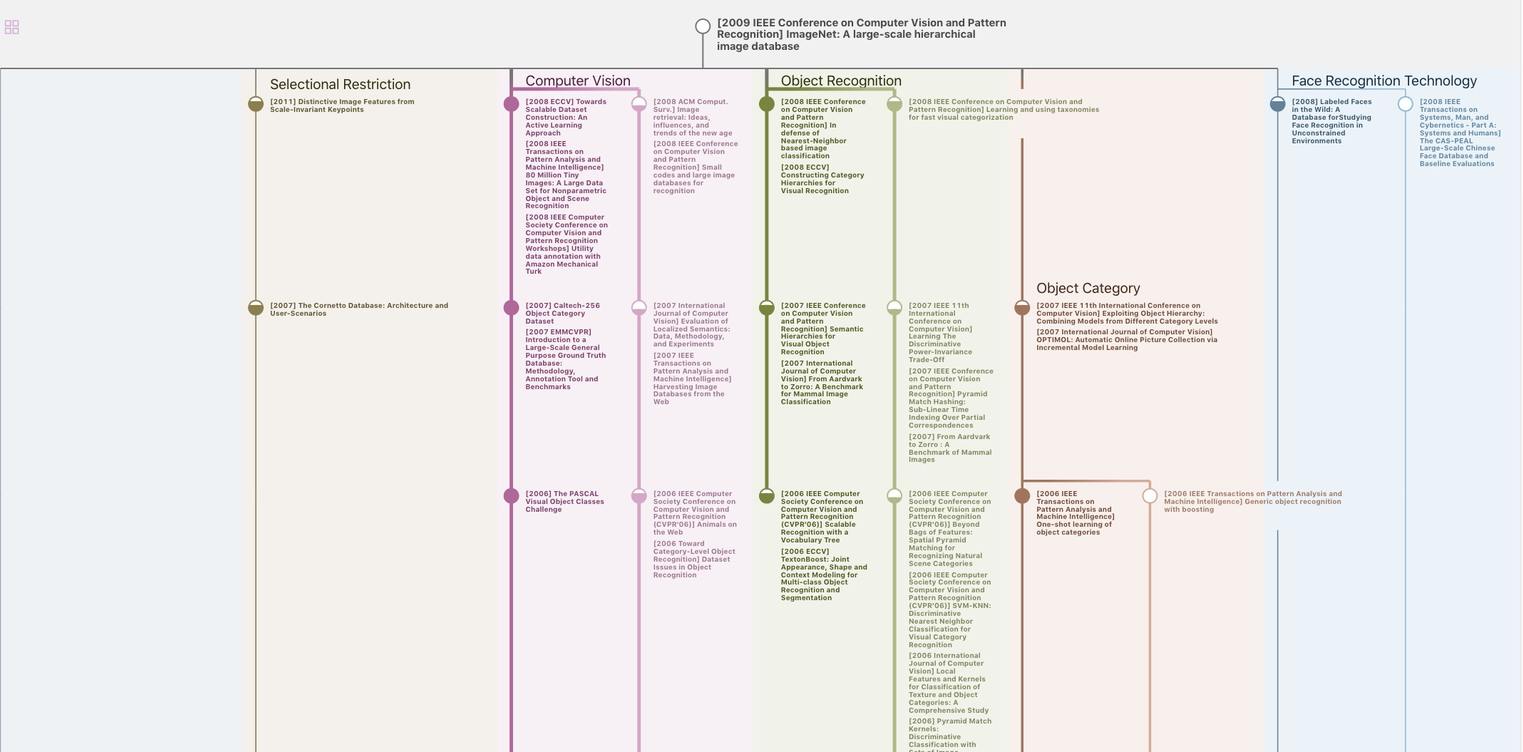
生成溯源树,研究论文发展脉络
Chat Paper
正在生成论文摘要