Time-Series-Based Feature Selection and Clustering for Equine Activity Recognition Using Accelerometers
IEEE Sensors Journal(2023)
摘要
With over 16 million horses worldwide and nearly 60000 sport horses registered to the International Federation for Equestrian Sports database, tracking the activities and performance of these equines is becoming an important aspect in horse management. To perform this activity recognition, inertial measurement units (IMUs) are often used in combination with machine learning algorithms. These often require large labeled datasets to be trained. To this end, a data-efficient algorithm is proposed that requires only 3 min of labeled calibration data. This is achieved by combining supervised feature selection, using the tsfresh time-series feature calculation library and the Kendall rank correlation coefficient, with a distance-based clustering algorithm. The generalizability performance of the algorithm is tested by evaluating on a dataset captured with leg-mounted IMUs and on a dataset captured using a neck-mounted IMU. On both datasets, the algorithm achieved the accuracies of 95%, comparable to state-of-the-art deep learning approaches, when calibrating and evaluating using the same horse. When the algorithm was calibrated on data from multiple horses and evaluated on horses that were not in the calibration dataset, a
${15}\%$
drop in classification accuracy is observed. The proposed algorithm is compared with fully supervised algorithms, such as convolutional neutral network, support vector machine, and random forest, in terms of accuracy achieved with respect to the size of the labeled data using calibration. Our approach achieved accuracies that were similar to these classical algorithms while only using 10%–
${5}\%$
the amount of labeled data.
更多查看译文
关键词
equine activity recognition,accelerometers,clustering,time-series
AI 理解论文
溯源树
样例
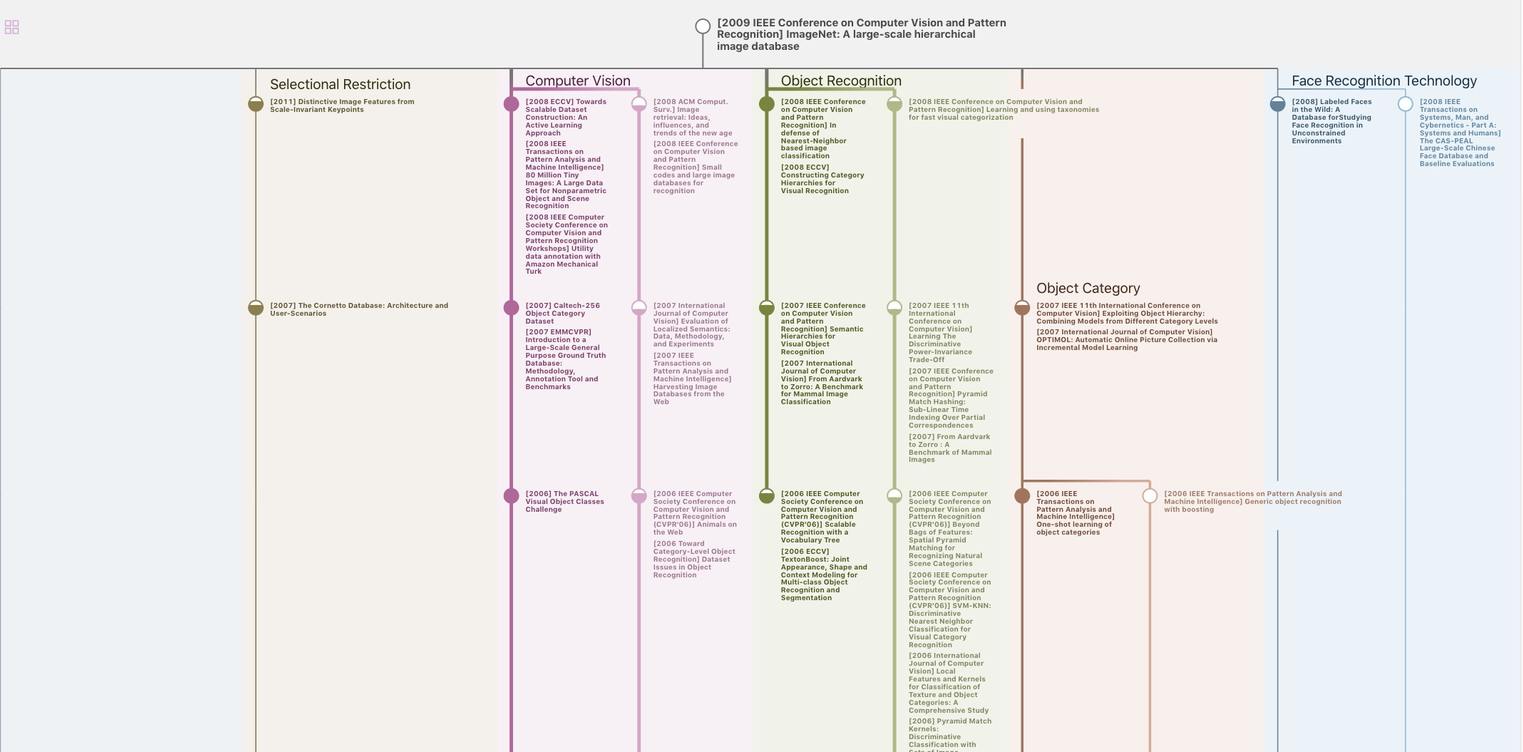
生成溯源树,研究论文发展脉络
Chat Paper
正在生成论文摘要