TPDM: Selectively Removing Positional Information for Zero-shot Translation via Token-Level Position Disentangle Module
CoRR(2023)
摘要
Due to Multilingual Neural Machine Translation's (MNMT) capability of zero-shot translation, many works have been carried out to fully exploit the potential of MNMT in zero-shot translation. It is often hypothesized that positional information may hinder the MNMT from outputting a robust encoded representation for decoding. However, previous approaches treat all the positional information equally and thus are unable to selectively remove certain positional information. In sharp contrast, this paper investigates how to learn to selectively preserve useful positional information. We describe the specific mechanism of positional information influencing MNMT from the perspective of linguistics at the token level. We design a token-level position disentangle module (TPDM) framework to disentangle positional information at the token level based on the explanation. Our experiments demonstrate that our framework improves zero-shot translation by a large margin while reducing the performance loss in the supervised direction compared to previous works.
更多查看译文
关键词
positional information,translation,zero-shot,token-level
AI 理解论文
溯源树
样例
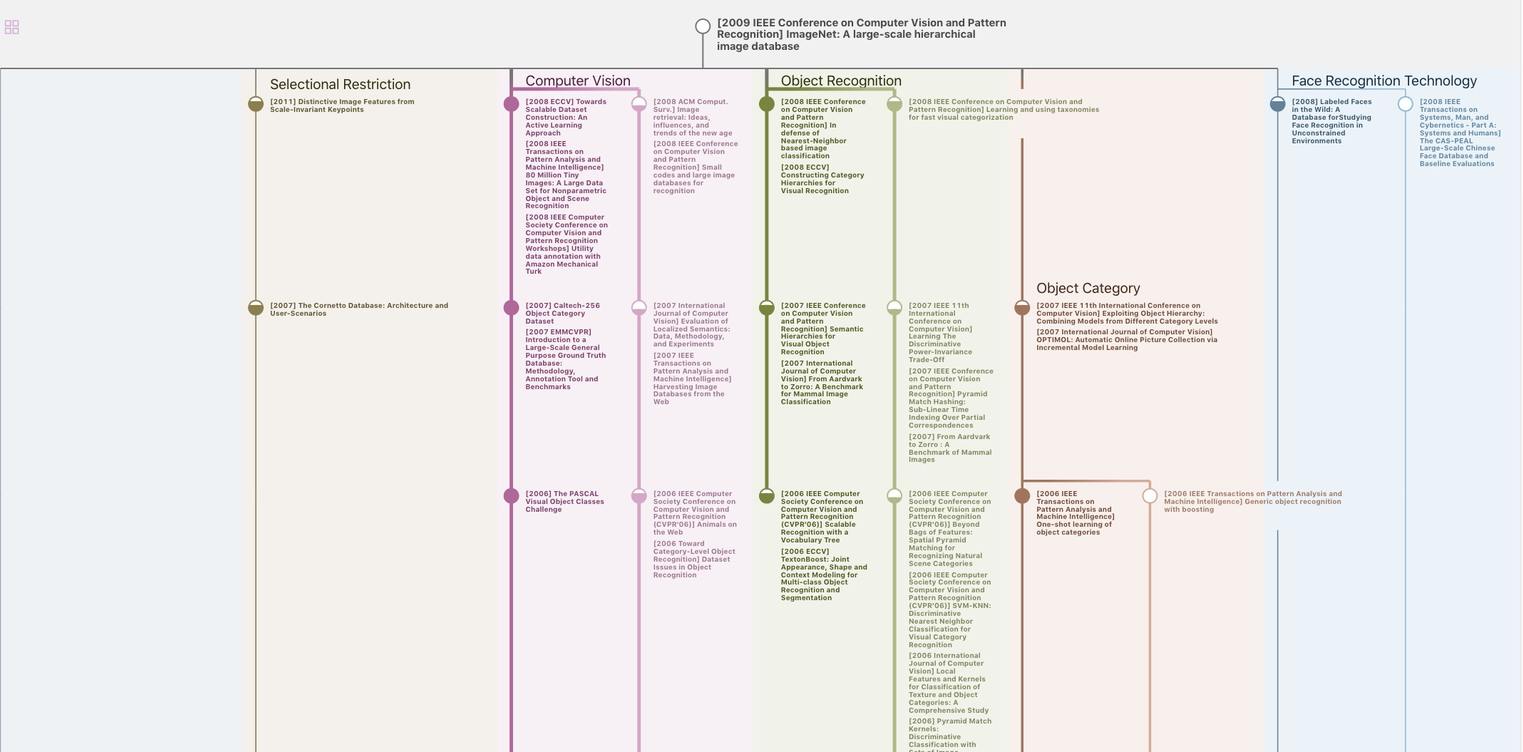
生成溯源树,研究论文发展脉络
Chat Paper
正在生成论文摘要