Online-to-PAC Conversions: Generalization Bounds via Regret Analysis
CoRR(2023)
摘要
We present a new framework for deriving bounds on the generalization bound of statistical learning algorithms from the perspective of online learning. Specifically, we construct an online learning game called the "generalization game", where an online learner is trying to compete with a fixed statistical learning algorithm in predicting the sequence of generalization gaps on a training set of i.i.d. data points. We establish a connection between the online and statistical learning setting by showing that the existence of an online learning algorithm with bounded regret in this game implies a bound on the generalization error of the statistical learning algorithm, up to a martingale concentration term that is independent of the complexity of the statistical learning method. This technique allows us to recover several standard generalization bounds including a range of PAC-Bayesian and information-theoretic guarantees, as well as generalizations thereof.
更多查看译文
关键词
regret analysis,generalization bounds,online-to-pac
AI 理解论文
溯源树
样例
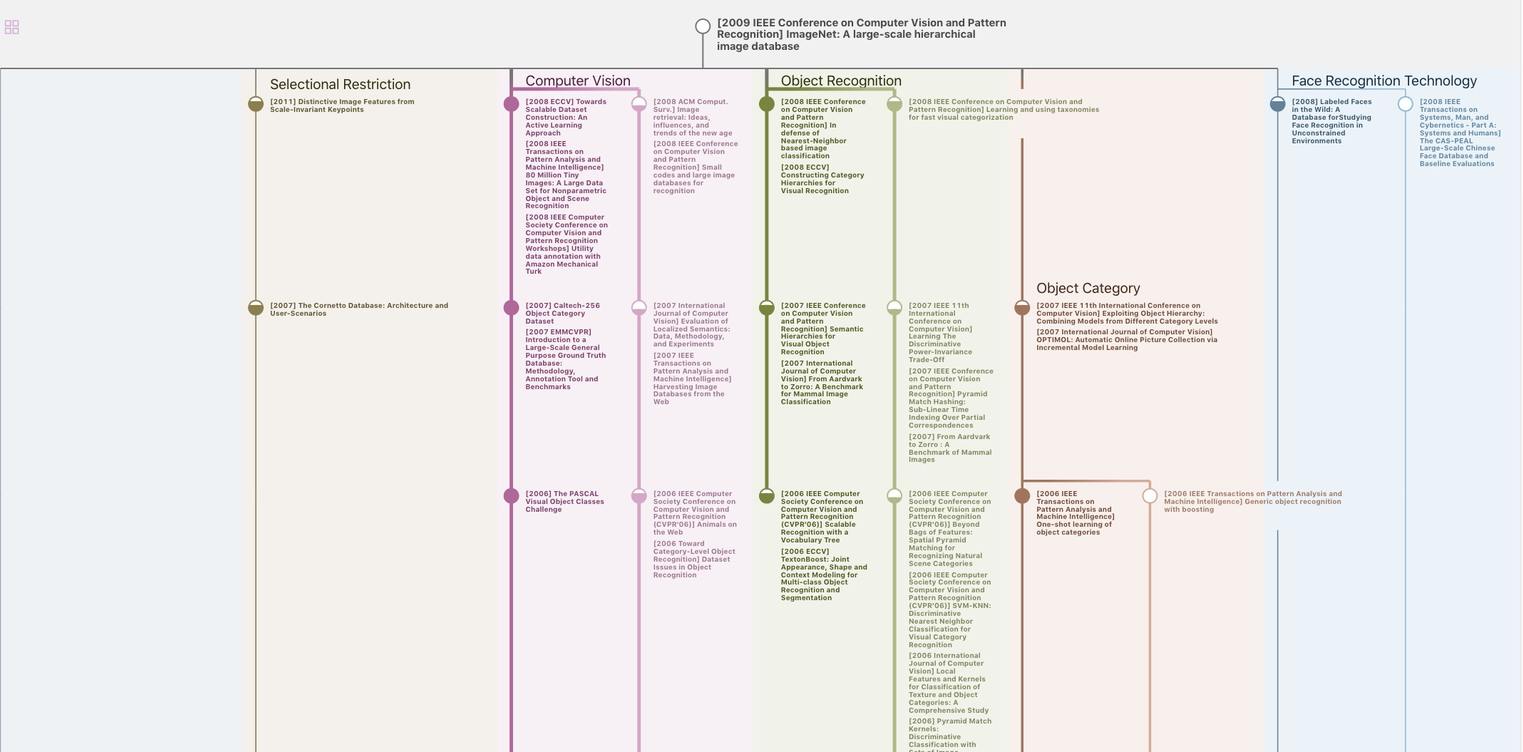
生成溯源树,研究论文发展脉络
Chat Paper
正在生成论文摘要