Accurate and Structured Pruning for Efficient Automatic Speech Recognition
CoRR(2023)
摘要
Automatic Speech Recognition (ASR) has seen remarkable advancements with deep neural networks, such as Transformer and Conformer. However, these models typically have large model sizes and high inference costs, posing a challenge to deploy on resource-limited devices. In this paper, we propose a novel compression strategy that leverages structured pruning and knowledge distillation to reduce the model size and inference cost of the Conformer model while preserving high recognition performance. Our approach utilizes a set of binary masks to indicate whether to retain or prune each Conformer module, and employs L0 regularization to learn the optimal mask values. To further enhance pruning performance, we use a layerwise distillation strategy to transfer knowledge from unpruned to pruned models. Our method outperforms all pruning baselines on the widely used LibriSpeech benchmark, achieving a 50% reduction in model size and a 28% reduction in inference cost with minimal performance loss.
更多查看译文
关键词
efficient automatic speech
AI 理解论文
溯源树
样例
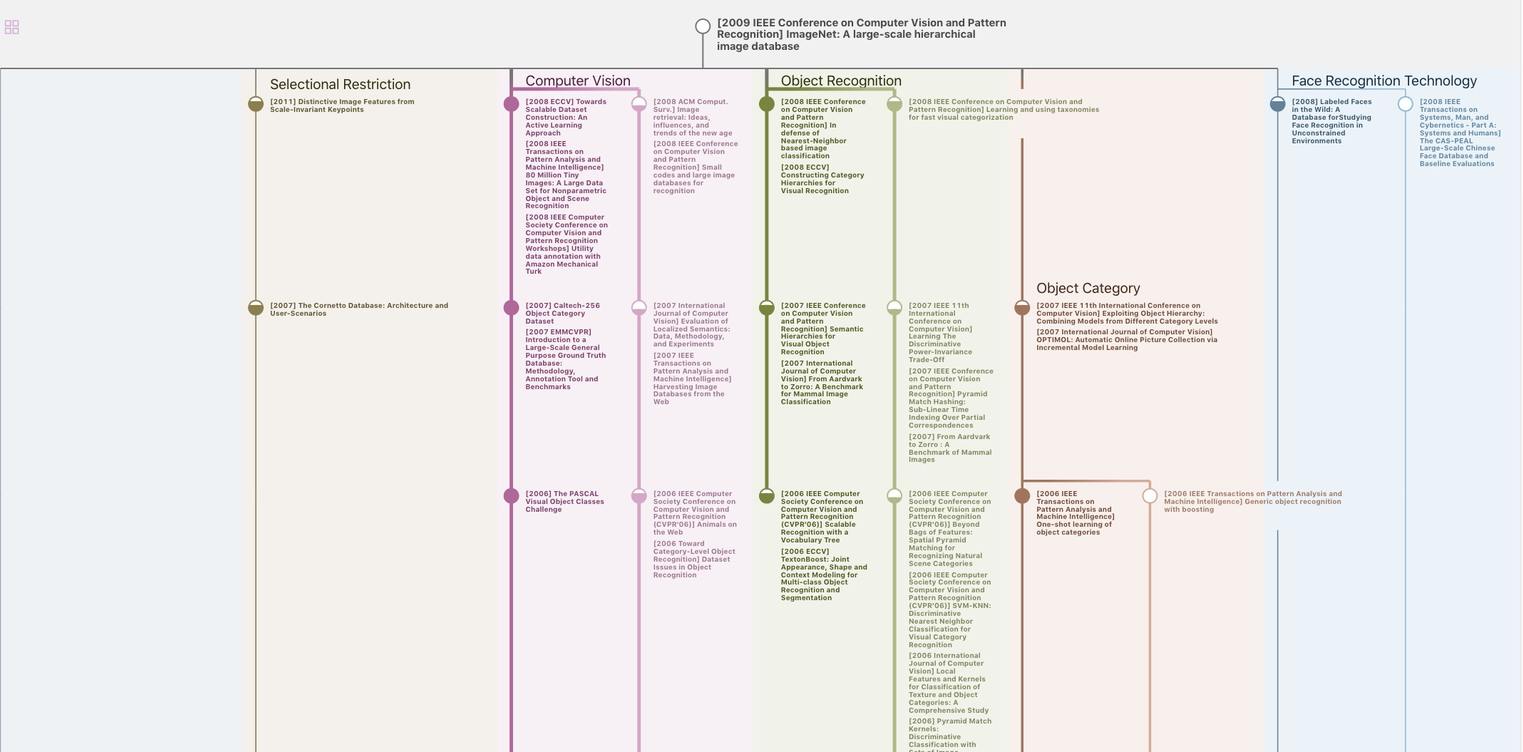
生成溯源树,研究论文发展脉络
Chat Paper
正在生成论文摘要