Efficient Implementation of a Multi-Layer Gradient-Free Online-Trainable Spiking Neural Network on FPGA
CoRR(2023)
摘要
This paper presents an efficient hardware implementation of the recently proposed Optimized Deep Event-driven Spiking Neural Network Architecture (ODESA). ODESA is the first network to have end-to-end multi-layer online local supervised training without using gradients and has the combined adaptation of weights and thresholds in an efficient hierarchical structure. This research shows that the network architecture and the online training of weights and thresholds can be implemented efficiently on a large scale in hardware. The implementation consists of a multi-layer Spiking Neural Network (SNN) and individual training modules for each layer that enable online self-learning without using back-propagation. By using simple local adaptive selection thresholds, a Winner-Takes-All (WTA) constraint on each layer, and a modified weight update rule that is more amenable to hardware, the trainer module allocates neuronal resources optimally at each layer without having to pass high-precision error measurements across layers. All elements in the system, including the training module, interact using event-based binary spikes. The hardware-optimized implementation is shown to preserve the performance of the original algorithm across multiple spatial-temporal classification problems with significantly reduced hardware requirements.
更多查看译文
关键词
spiking neural network,fpga,multi-layer,gradient-free,online-trainable
AI 理解论文
溯源树
样例
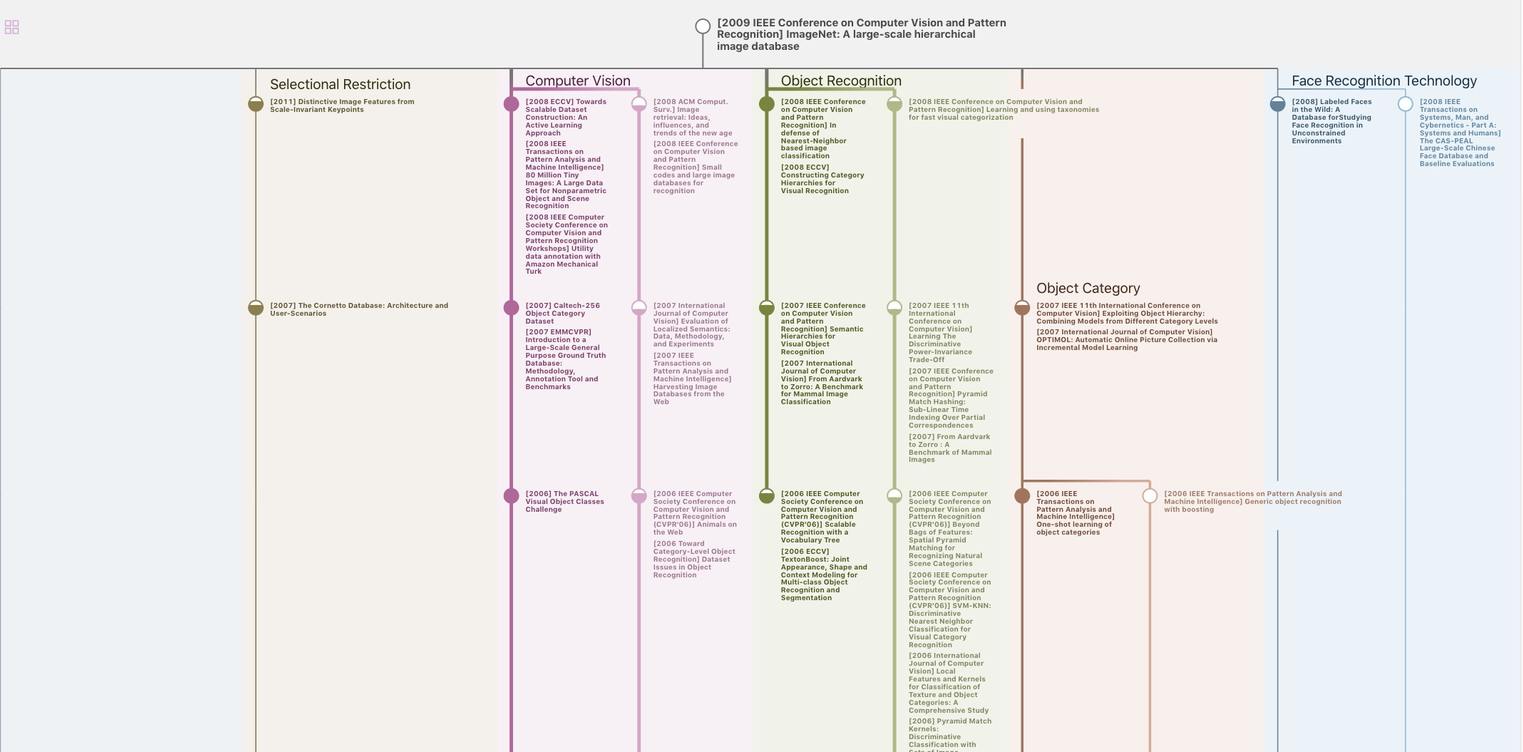
生成溯源树,研究论文发展脉络
Chat Paper
正在生成论文摘要