Convolutional neural network reveals frequency content of medio-lateral COM body sway to be highly predictive of Parkinson's disease
medRxiv (Cold Spring Harbor Laboratory)(2023)
摘要
Postural instability as a symptom of progressing Parkinson's disease (PD) greatly reduces quality of life. Hence, early detection of postural impairments is crucial to facilitate interventions. Our aim was to use a convolutional neural network (CNN) to differentiate people with early to mid-stage PD from healthy age-matched individuals based on spectrogram images obtained from their body movement. We hypothesized the time-frequency content of body sway to be predictive of PD, even when impairments are not yet manifested in day-to-day postural control. We tracked their center of pressure (COP) using a Wii Balance Board and their full-body motion using a Microsoft Kinect, out of which we calculated the trajectory of their center of mass (COM). We used 30 s-snippets of motion data from which we acquired wavelet-based time-frequency spectrograms that were fed into a custom-built CNN as labeled images. We used binary classification to have the network differentiate between individuals with PD and controls (n=15, respectively). Classification performance was best when the medio-lateral motion of the COM was considered. Here, our network reached an average predictive accuracy of 98.45 % with a receiver operating characteristic area under the curve of 1.0. Moreover, an explainable AI approach revealed high frequencies in the postural sway data to be most distinct between both groups. Our findings suggest a CNN classifier based on cost-effective and conveniently obtainable posturographic data to be a promising approach to detect postural impairments in early to mid-stage PD and to gain novel insight into the subtle characteristics of impairments at this stage of the disease.
### Competing Interest Statement
The authors have declared no competing interest.
### Funding Statement
This study was funded by Deutsche Forschungsgemeinschaft: IRTG-1901, CRC/TRR-135 (Project No. 222641018), European Union: PLATYPUS, and Hessisches Ministerium fuer Wissenschaft und Kunst: The Adaptive Mind (TAM). Author A.P. is supported by a Marie Skłodowska-Curie Actions, H2020-MSCA-IF-2018 grant (ID: 832518, Project: MOVES).
### Author Declarations
I confirm all relevant ethical guidelines have been followed, and any necessary IRB and/or ethics committee approvals have been obtained.
Yes
The details of the IRB/oversight body that provided approval or exemption for the research described are given below:
The ethics committee of the Psychology Department, University of Marburg, and the ethics committee of the Faculty of Medicine, University of Marburg (case 77/19), gave ethical approval for this work.
I confirm that all necessary patient/participant consent has been obtained and the appropriate institutional forms have been archived, and that any patient/participant/sample identifiers included were not known to anyone (e.g., hospital staff, patients or participants themselves) outside the research group so cannot be used to identify individuals.
Yes
I understand that all clinical trials and any other prospective interventional studies must be registered with an ICMJE-approved registry, such as ClinicalTrials.gov. I confirm that any such study reported in the manuscript has been registered and the trial registration ID is provided (note: if posting a prospective study registered retrospectively, please provide a statement in the trial ID field explaining why the study was not registered in advance).
Yes
I have followed all appropriate research reporting guidelines, such as any relevant EQUATOR Network research reporting checklist(s) and other pertinent material, if applicable.
Yes
All data and code produced in the present study are available upon request to the authors. They will be made available online soon.
更多查看译文
关键词
parkinsons,convolutional neural network,medio-lateral
AI 理解论文
溯源树
样例
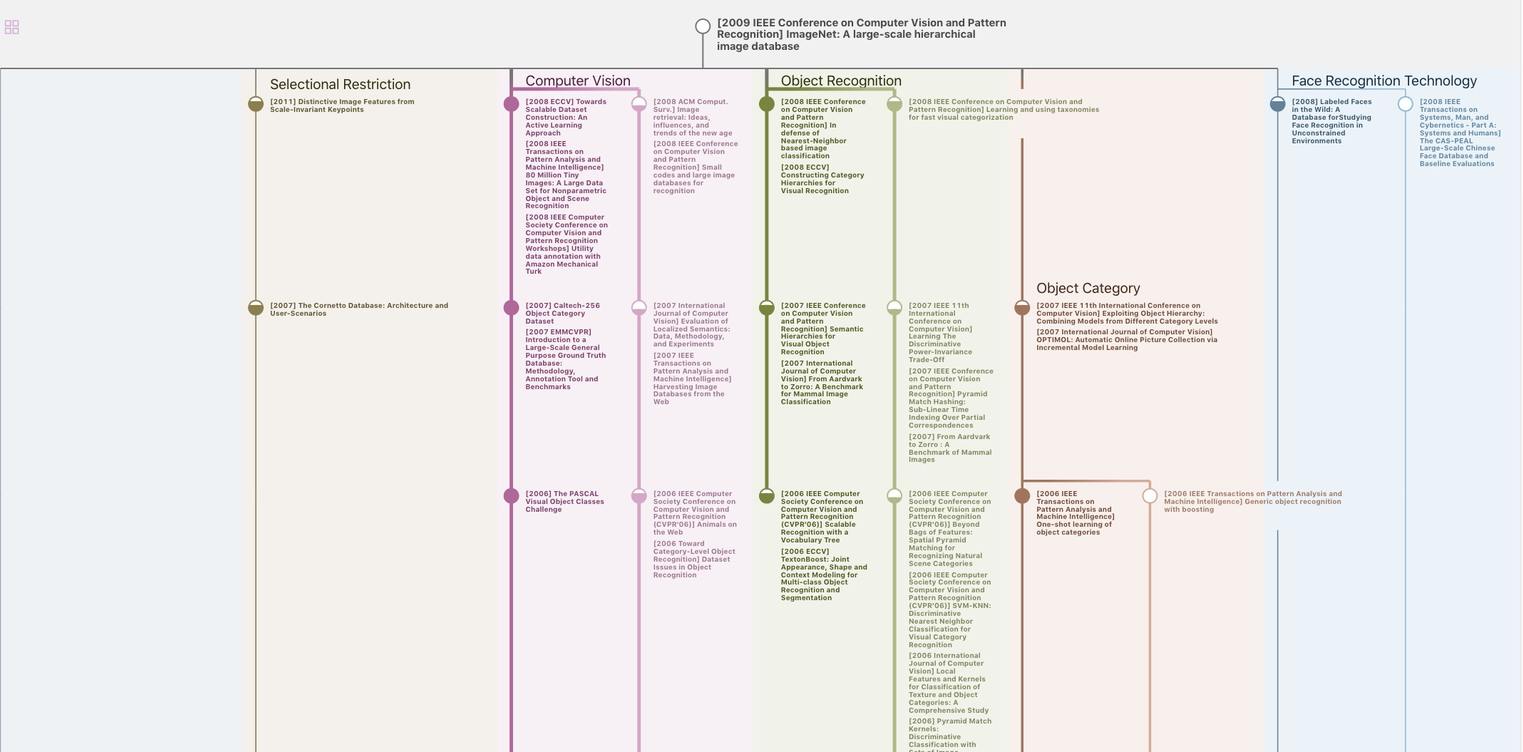
生成溯源树,研究论文发展脉络
Chat Paper
正在生成论文摘要