Sharp high-probability sample complexities for policy evaluation with linear function approximation
CoRR(2023)
摘要
This paper is concerned with the problem of policy evaluation with linear function approximation in discounted infinite horizon Markov decision processes. We investigate the sample complexities required to guarantee a predefined estimation error of the best linear coefficients for two widely-used policy evaluation algorithms: the temporal difference (TD) learning algorithm and the two-timescale linear TD with gradient correction (TDC) algorithm. In both the on-policy setting, where observations are generated from the target policy, and the off-policy setting, where samples are drawn from a behavior policy potentially different from the target policy, we establish the first sample complexity bound with high-probability convergence guarantee that attains the optimal dependence on the tolerance level. We also exhihit an explicit dependence on problem-related quantities, and show in the on-policy setting that our upper bound matches the minimax lower bound on crucial problem parameters, including the choice of the feature maps and the problem dimension.
更多查看译文
关键词
policy evaluation,linear function approximation,high-probability
AI 理解论文
溯源树
样例
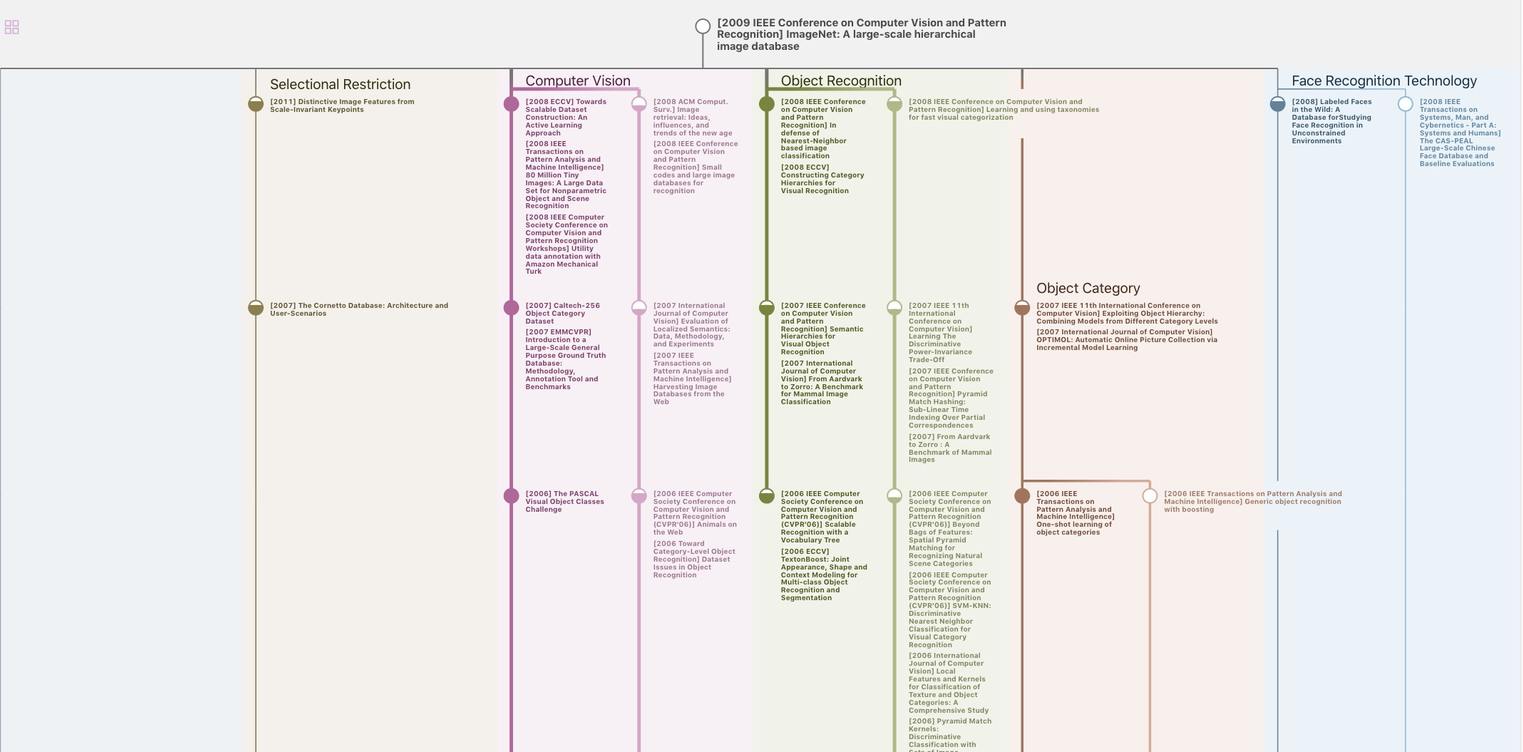
生成溯源树,研究论文发展脉络
Chat Paper
正在生成论文摘要