Toward Real-World Light Field Super-Resolution
CoRR(2023)
摘要
Deep learning has opened up new possibilities for light field super-resolution (SR), but existing methods trained on synthetic datasets with simple degradations (e.g., bicubic downsampling) suffer from poor performance when applied to complex real-world scenarios. To address this problem, we introduce LytroZoom, the first real-world light field SR dataset capturing paired low- and high-resolution light fields of diverse indoor and outdoor scenes using a Lytro ILLUM camera. Additionally, we propose the Omni-Frequency Projection Network (OFPNet), which decomposes the omni-frequency components and iteratively enhances them through frequency projection operations to address spatially variant degradation processes present in all frequency components. Experiments demonstrate that models trained on LytroZoom outperform those trained on synthetic datasets and are generalizable to diverse content and devices. Quantitative and qualitative evaluations verify the superiority of OFPNet. We believe this work will inspire future research in real-world light field SR.
更多查看译文
关键词
bicubic downsampling,deep learning,diverse indoor scenes,frequency projection operations,high-resolution light fields,Lytro ILLUM camera,LytroZoom,omni-frequency components,Omni-Frequency Projection Network,outdoor scenes,real-world light field SR dataset,simple degradations,spatially variant degradation processes,synthetic datasets,toward real-world light field super-resolution
AI 理解论文
溯源树
样例
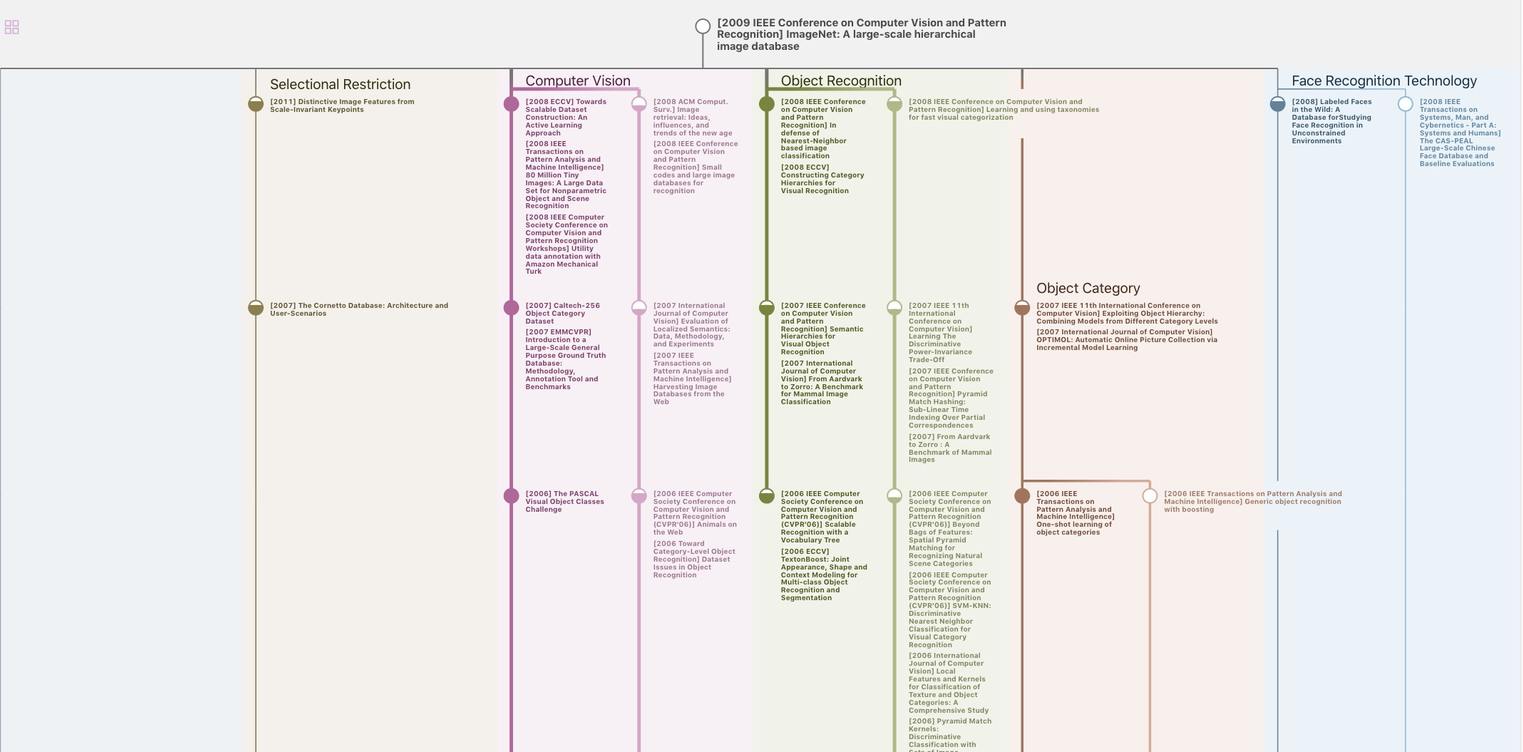
生成溯源树,研究论文发展脉络
Chat Paper
正在生成论文摘要