Representation Of Lexical Stylistic Features In Language Models' Embedding Space
CoRR(2023)
摘要
The representation space built by pretrained Language Models (LMs) encodes rich information about words and their relationships (e.g., similarity, hypernymy/hyponymy, polysemy) as well as abstract semantic notions (e.g., intensity). In this paper, we demonstrate that lexical stylistic notions such as complexity, formality, and figurativeness, can also be identified in this space. We show that it is possible to derive a vector representation for each of these stylistic notions, from only a small number of seed text pairs. Using these vectors, we can characterize new texts in terms of these dimensions using simple calculations in the corresponding embedding space. We perform experiments on five datasets and find that static embeddings encode these features more accurately at the level of words and phrases, whereas contextualized LMs perform better on longer texts. The lower performance of contextualized representations at the word level is partially attributable to the anisotropy of their vector space, which can be corrected through techniques like standardization to further improve performance.
更多查看译文
关键词
lexical stylistic features,language models,embedding
AI 理解论文
溯源树
样例
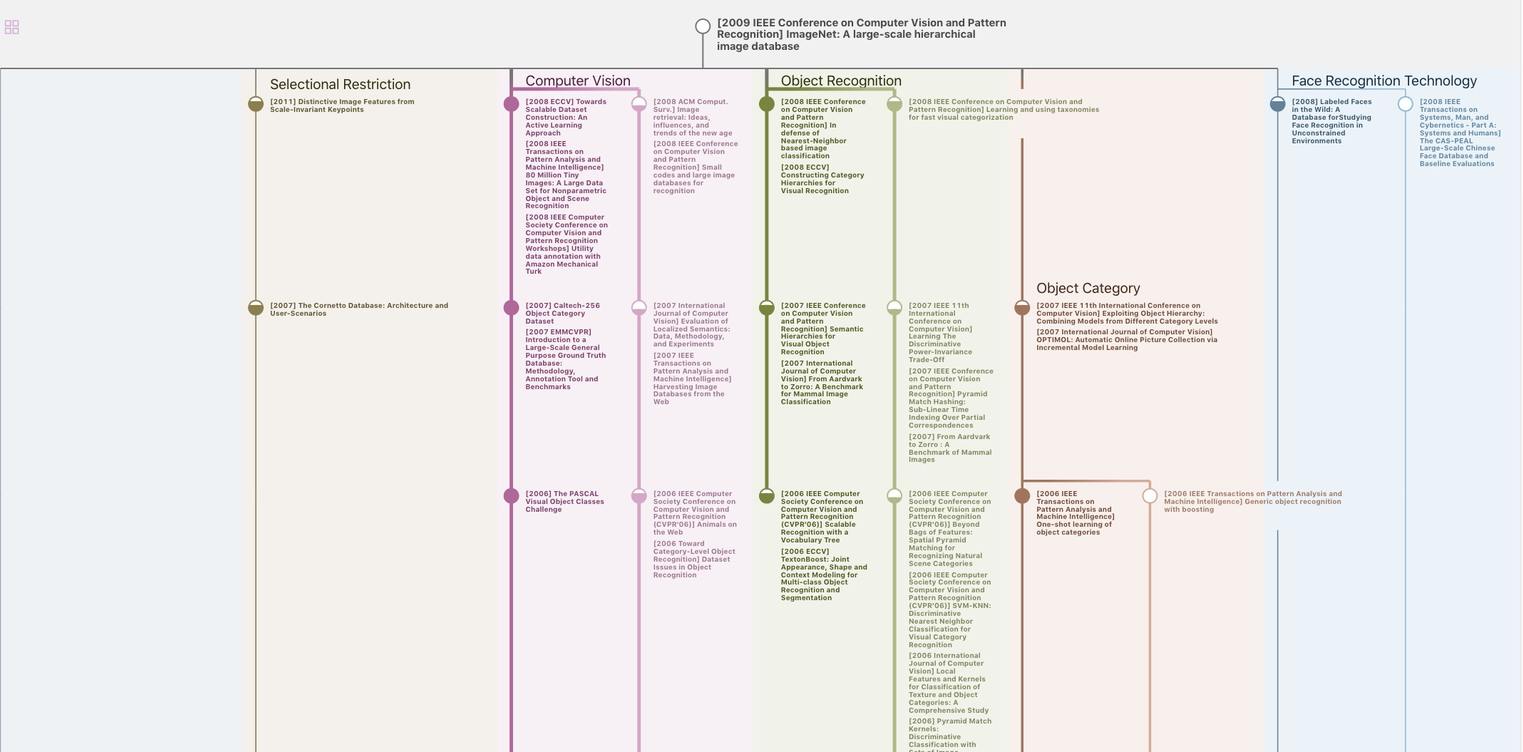
生成溯源树,研究论文发展脉络
Chat Paper
正在生成论文摘要