Off-Policy RL Algorithms Can be Sample-Efficient for Continuous Control via Sample Multiple Reuse
Information Sciences(2024)
摘要
Sample efficiency is one of the most critical issues for online reinforcement learning (RL). Existing methods achieve higher sample efficiency by adopting model-based methods, Q-ensemble, or better exploration mechanisms. We, instead, propose to train an off-policy RL agent via updating on a fixed sampled batch multiple times, thus reusing these samples and better exploiting them within a single optimization loop. We name our method sample multiple reuse (SMR). We theoretically show the properties of Q-learning with SMR, e.g., convergence. Furthermore, we incorporate SMR with off-the-shelf off-policy RL algorithms and conduct experiments on a variety of continuous control benchmarks. Empirical results show that SMR significantly boosts the sample efficiency of the base methods across most of the evaluated tasks without any hyperparameter tuning or additional tricks.
更多查看译文
关键词
continuous control
AI 理解论文
溯源树
样例
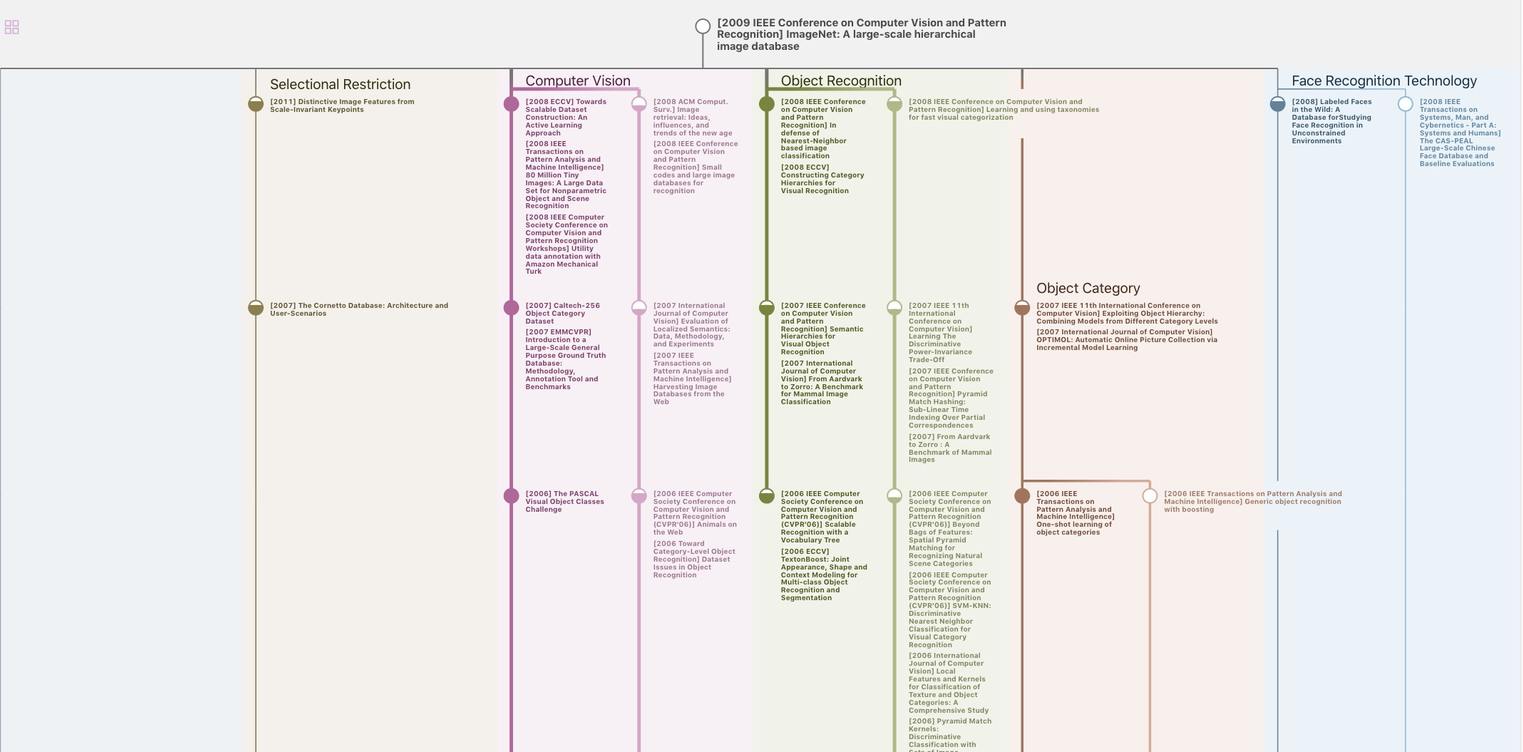
生成溯源树,研究论文发展脉络
Chat Paper
正在生成论文摘要