Feature-Learning Networks Are Consistent Across Widths At Realistic Scales
NeurIPS(2023)
摘要
We study the effect of width on the dynamics of feature-learning neural networks across a variety of architectures and datasets. Early in training, wide neural networks trained on online data have not only identical loss curves but also agree in their point-wise test predictions throughout training. For simple tasks such as CIFAR-5m this holds throughout training for networks of realistic widths. We also show that structural properties of the models, including internal representations, preactivation distributions, edge of stability phenomena, and large learning rate effects are consistent across large widths. This motivates the hypothesis that phenomena seen in realistic models can be captured by infinite-width, feature-learning limits. For harder tasks (such as ImageNet and language modeling), and later training times, finite-width deviations grow systematically. Two distinct effects cause these deviations across widths. First, the network output has initialization-dependent variance scaling inversely with width, which can be removed by ensembling networks. We observe, however, that ensembles of narrower networks perform worse than a single wide network. We call this the bias of narrower width. We conclude with a spectral perspective on the origin of this finite-width bias.
更多查看译文
关键词
consistent across widths,networks,feature-learning
AI 理解论文
溯源树
样例
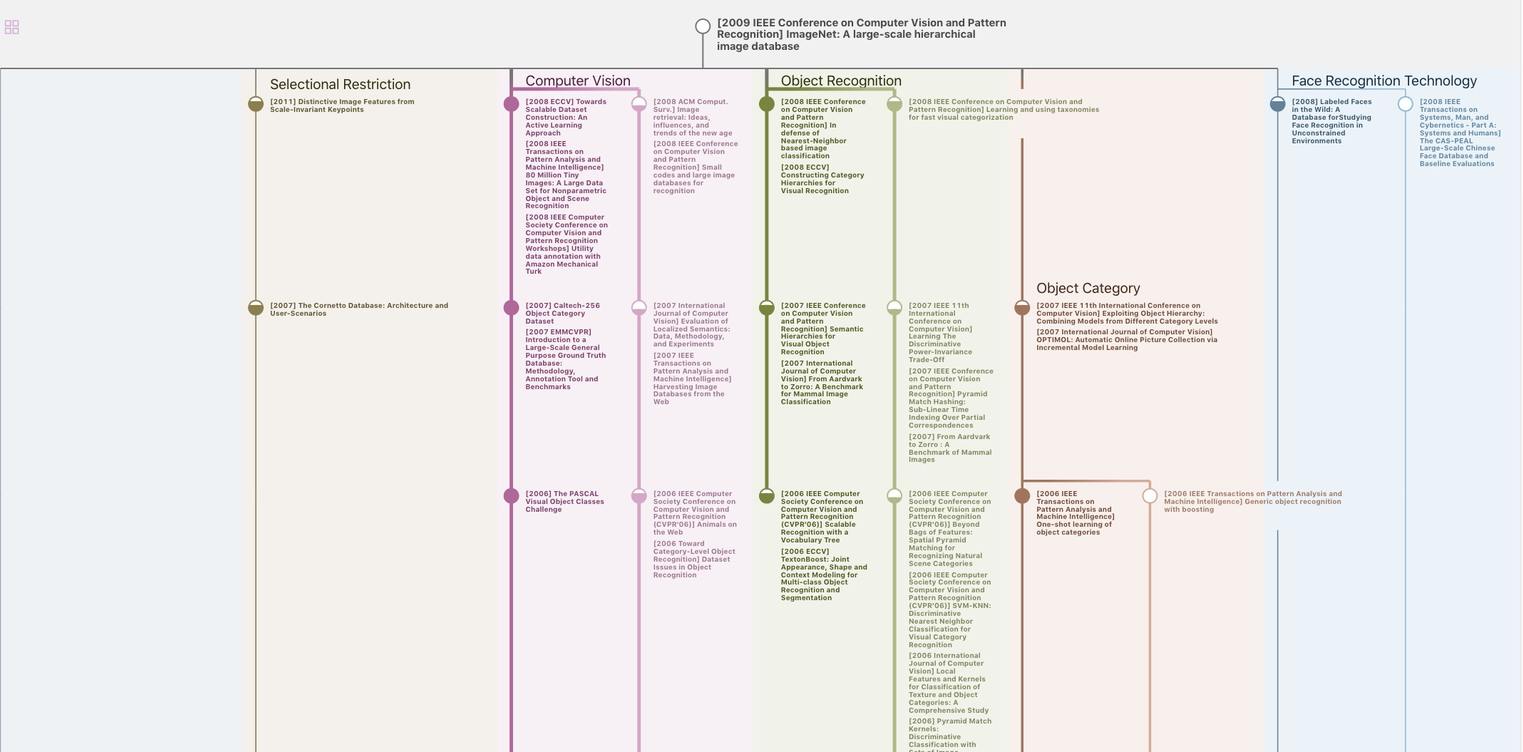
生成溯源树,研究论文发展脉络
Chat Paper
正在生成论文摘要