Test Cost Reduction for 5G and Beyond using Machine Learning
2023 IEEE International Conference on Software Testing, Verification and Validation Workshops (ICSTW)(2023)
摘要
Software testing is essential, but expensive, especially for significant issues, feature-rich systems such as telecommunication systems evolving toward 5G and beyond. There is a need in this domain for effective testing techniques to ensure that a minimal number of test cases assess the most important combinations of system functions with respect to domain-specific criteria.Our approach aims to address this challenge by first automatically mapping existing test cases to the combinations of system capabilities they exercise and visualizing the mappings using decision tree learners. Then, the approach uses a combination of the engineers’ feedback (domain-specific criteria), mapping data, and test execution logs to propose new test cases covering newly-added capabilities or better exercising/verifying existing ones while ensuring the efficacy at fault detection, code coverage, equipment cost, test execution time, redundancy avoidance, among other things.
更多查看译文
关键词
5G Systems, Machine Learning, Software Testing
AI 理解论文
溯源树
样例
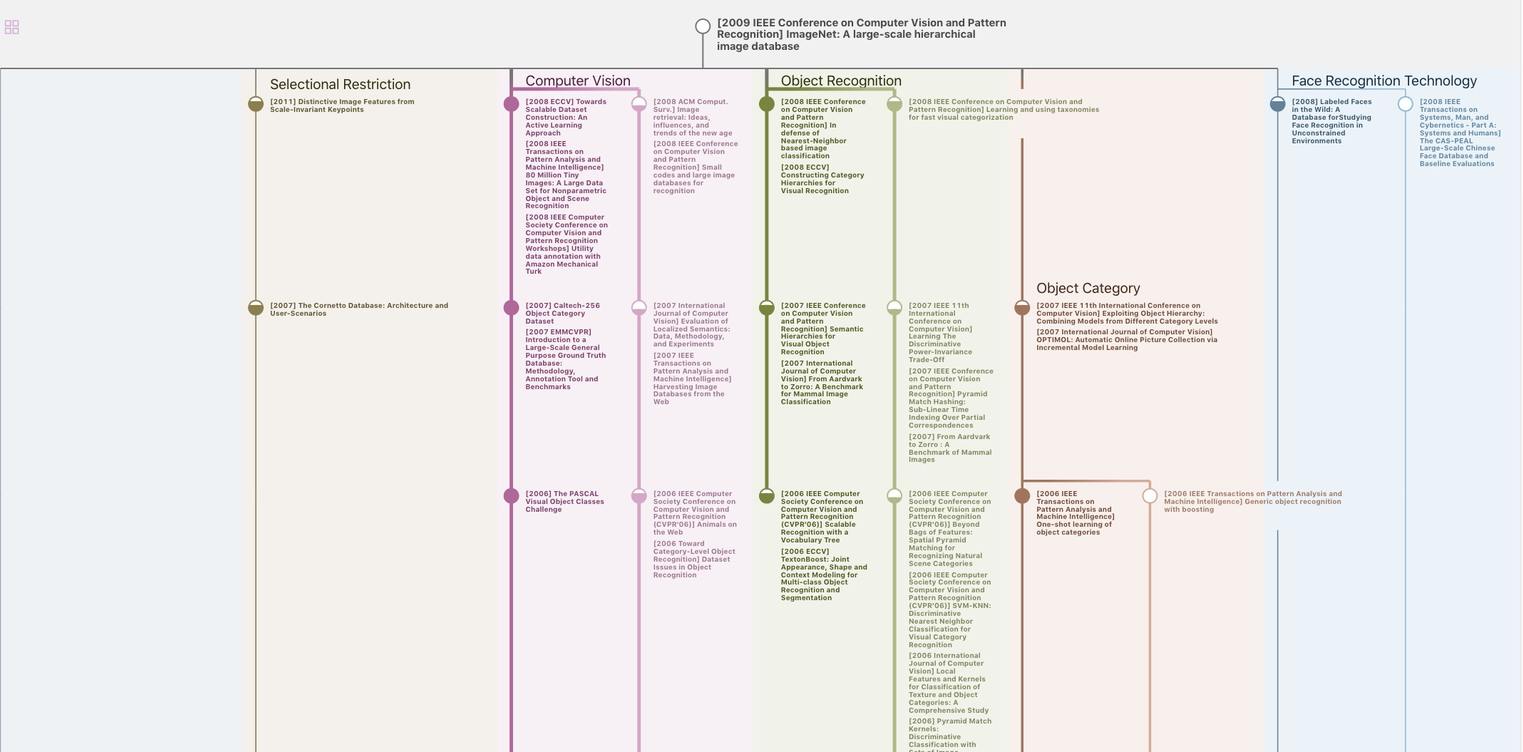
生成溯源树,研究论文发展脉络
Chat Paper
正在生成论文摘要