Provably Efficient Offline RL with Options
AAMAS '23: Proceedings of the 2023 International Conference on Autonomous Agents and Multiagent Systems(2023)
摘要
Temporal abstraction helps to reduce the sample complexity in long-horizon planning in reinforcement learning (RL). One powerful approach is the options framework, where the agent interacts with the environment using closed-loop policies, i.e., options, instead of primitive actions. Recent works show that in the online setting, where the agent can continuously explore the environment, lower PAC-like sample complexity or regret can be attained by learning with options. However, these results are no longer applicable in scenarios where collecting data in an online manner is impossible, e.g., automated driving and healthcare. In this paper, we provide the first analysis of the sample complexity for offline RL with options, where a dataset is provided and no further interaction with the environment is allowed. Two procedures of the data collecting process are considered, which adapt to different scenes of applications and are of great importance to study. Inspired by previous works on offline RL, we propose PEssimistic Value Iteration for Learning with Options (PEVIO) and derive suboptimality bounds for both datasets, which are near-optimal according to a novel information-theoretic lower bound for offline RL with options. Further, the suboptimality bound shows that learning with options can be more sample-efficient than learning with primitive actions in the offline setting.
更多查看译文
AI 理解论文
溯源树
样例
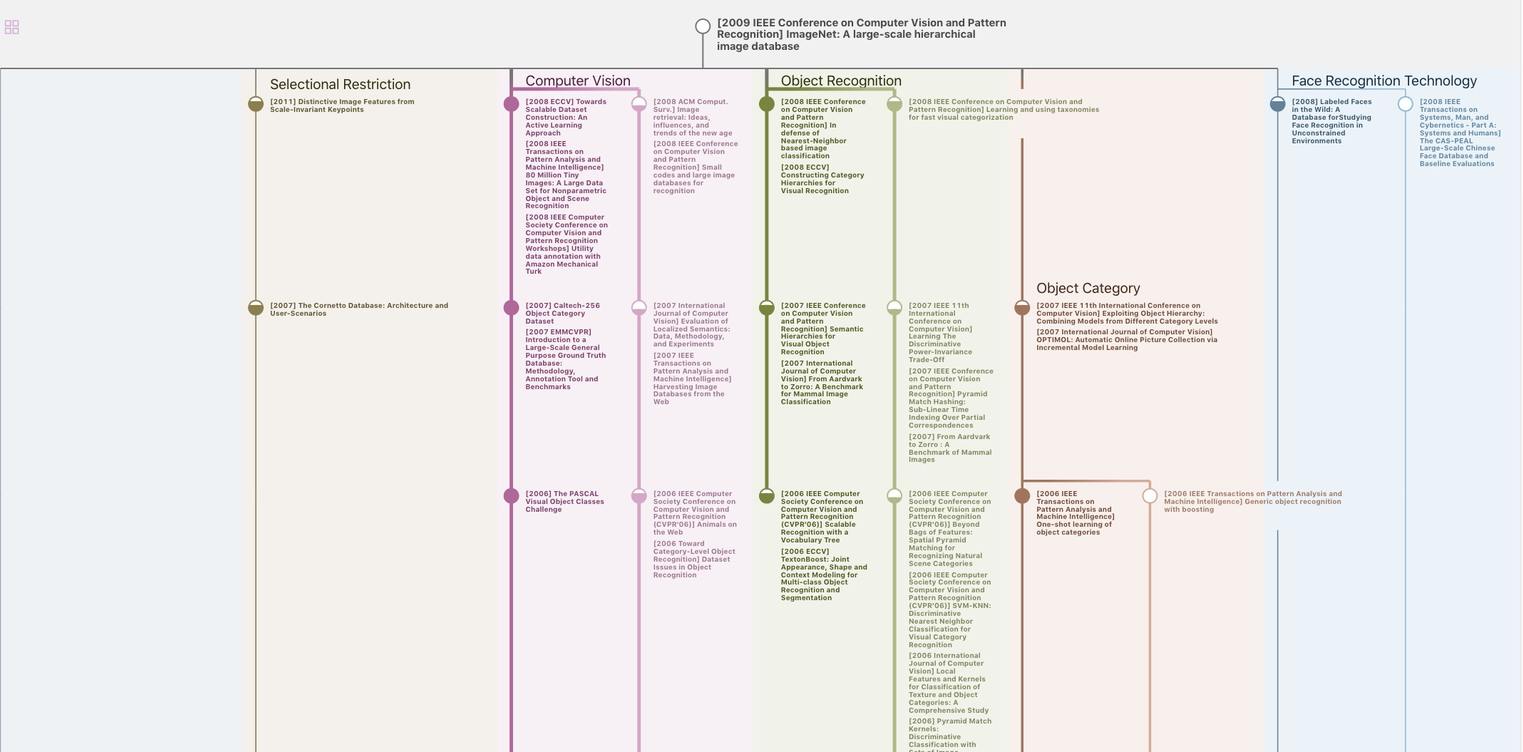
生成溯源树,研究论文发展脉络
Chat Paper
正在生成论文摘要