A Hierarchical Context-aware Modeling Approach for Multi-aspect and Multi-granular Pronunciation Assessment
CoRR(2023)
摘要
Automatic Pronunciation Assessment (APA) plays a vital role in Computer-assisted Pronunciation Training (CAPT) when evaluating a second language (L2) learner's speaking proficiency. However, an apparent downside of most de facto methods is that they parallelize the modeling process throughout different speech granularities without accounting for the hierarchical and local contextual relationships among them. In light of this, a novel hierarchical approach is proposed in this paper for multi-aspect and multi-granular APA. Specifically, we first introduce the notion of sup-phonemes to explore more subtle semantic traits of L2 speakers. Second, a depth-wise separable convolution layer is exploited to better encapsulate the local context cues at the sub-word level. Finally, we use a score-restraint attention pooling mechanism to predict the sentence-level scores and optimize the component models with a multitask learning (MTL) framework. Extensive experiments carried out on a publicly-available benchmark dataset, viz. speechocean762, demonstrate the efficacy of our approach in relation to some cutting-edge baselines.
更多查看译文
关键词
pronunciation,modeling,context-aware,multi-aspect,multi-granular
AI 理解论文
溯源树
样例
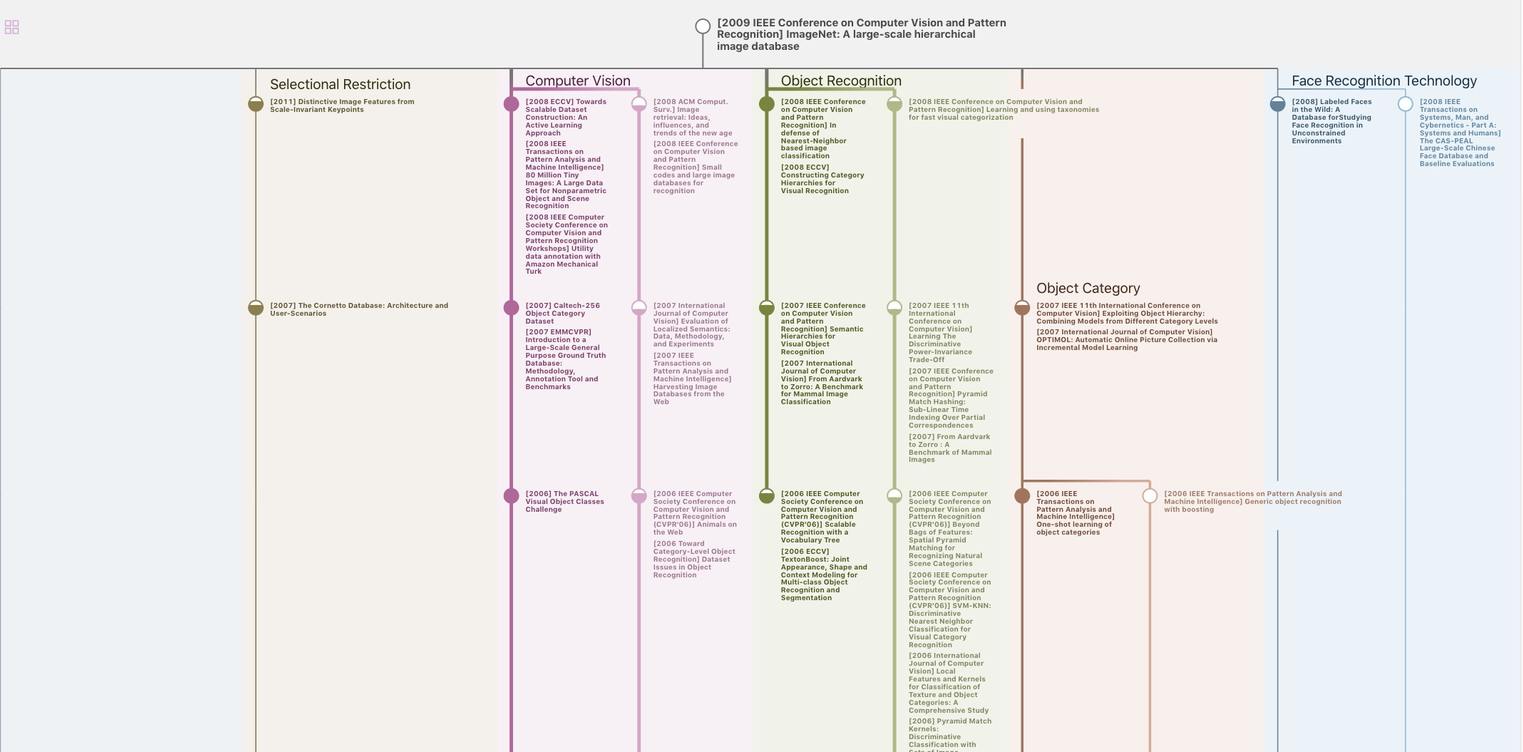
生成溯源树,研究论文发展脉络
Chat Paper
正在生成论文摘要