GMSF: Global Matching Scene Flow
NeurIPS(2023)
摘要
We tackle the task of scene flow estimation from point clouds. Given a source and a target point cloud, the objective is to estimate a translation from each point in the source point cloud to the target, resulting in a 3D motion vector field. Previous dominant scene flow estimation methods require complicated coarse-to-fine or recurrent architectures as a multi-stage refinement. In contrast, we propose a significantly simpler single-scale one-shot global matching to address the problem. Our key finding is that reliable feature similarity between point pairs is essential and sufficient to estimate accurate scene flow. To this end, we propose to decompose the feature extraction step via a hybrid local-global-cross transformer architecture which is crucial to accurate and robust feature representations. Extensive experiments show that GMSF sets a new state-of-the-art on multiple scene flow estimation benchmarks. On FlyingThings3D, with the presence of occlusion points, GMSF reduces the outlier percentage from the previous best performance of 27.4% to 11.7%. On KITTI Scene Flow, without any fine-tuning, our proposed method shows state-of-the-art performance.
更多查看译文
关键词
global matching scene,flow,gmsf
AI 理解论文
溯源树
样例
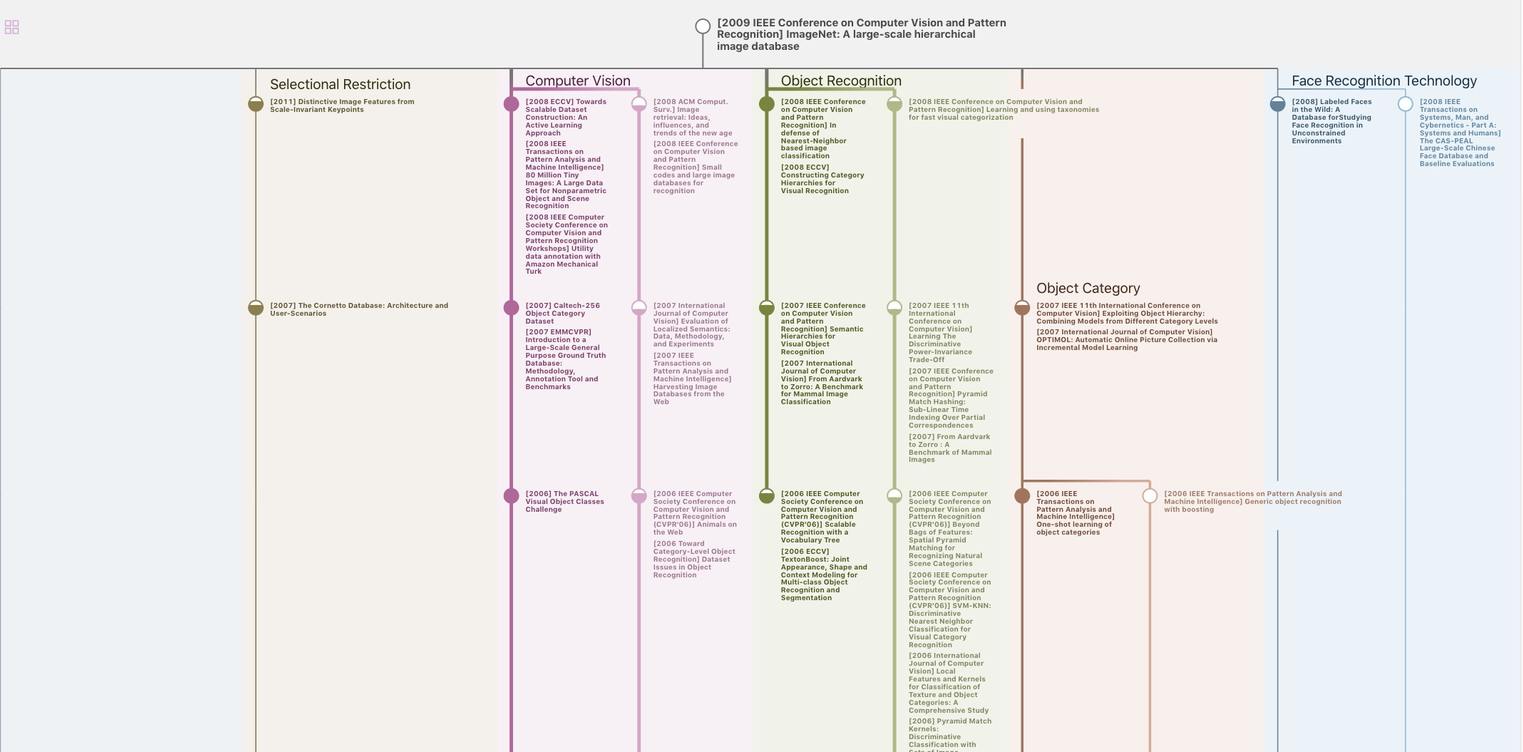
生成溯源树,研究论文发展脉络
Chat Paper
正在生成论文摘要