Understanding Emotion Valence is a Joint Deep Learning Task
CoRR(2023)
摘要
The valence analysis of speakers' utterances or written posts helps to understand the activation and variations of the emotional state throughout the conversation. More recently, the concept of Emotion Carriers (EC) has been introduced to explain the emotion felt by the speaker and its manifestations. In this work, we investigate the natural inter-dependency of valence and ECs via a multi-task learning approach. We experiment with Pre-trained Language Models (PLM) for single-task, two-step, and joint settings for the valence and EC prediction tasks. We compare and evaluate the performance of generative (GPT-2) and discriminative (BERT) architectures in each setting. We observed that providing the ground truth label of one task improves the prediction performance of the models in the other task. We further observed that the discriminative model achieves the best trade-off of valence and EC prediction tasks in the joint prediction setting. As a result, we attain a single model that performs both tasks, thus, saving computation resources at training and inference times.
更多查看译文
关键词
emotion valence,joint deep learning task,deep learning,understanding
AI 理解论文
溯源树
样例
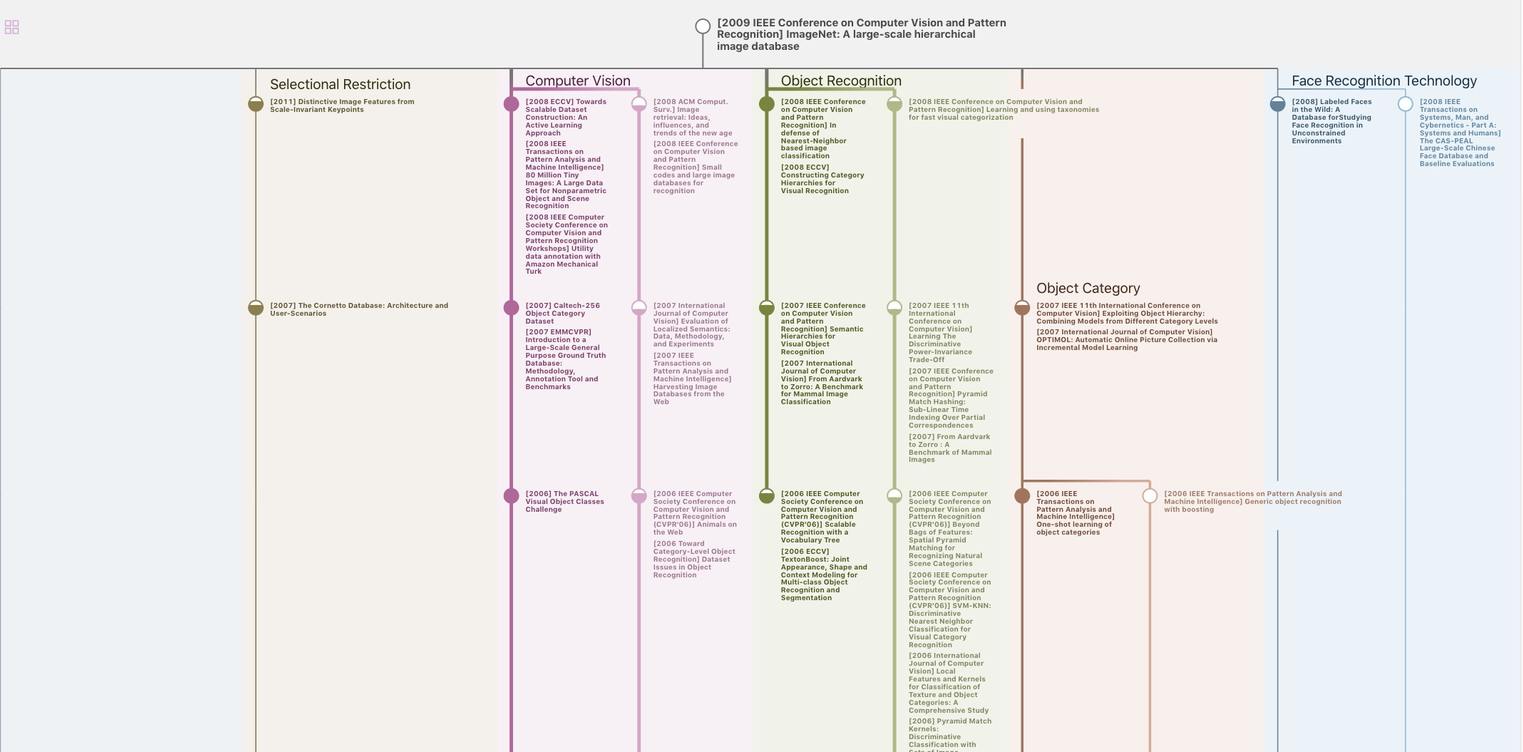
生成溯源树,研究论文发展脉络
Chat Paper
正在生成论文摘要