Augmentation-Adapted Retriever Improves Generalization of Language Models as Generic Plug-In
conf_acl(2023)
摘要
Retrieval augmentation can aid language models (LMs) in knowledge-intensive tasks by supplying them with external information. Prior works on retrieval augmentation usually jointly fine-tune the retriever and the LM, making them closely coupled. In this paper, we explore the scheme of generic retrieval plug-in: the retriever is to assist target LMs that may not be known beforehand or are unable to be fine-tuned together. To retrieve useful documents for unseen target LMs, we propose augmentation-adapted retriever (AAR), which learns LM's preferences obtained from a known source LM. Experiments on the MMLU and PopQA datasets demonstrate that our AAR trained with a small source LM is able to significantly improve the zero-shot generalization of larger target LMs ranging from 250M Flan-T5 to 175B InstructGPT. Further analysis indicates that the preferences of different LMs overlap, enabling AAR trained with a single source LM to serve as a generic plug-in for various target LMs. Our code is open-sourced at https://github.com/OpenMatch/Augmentation-Adapted-Retriever.
更多查看译文
关键词
language
models,generalization,augmentation-adapted
AI 理解论文
溯源树
样例
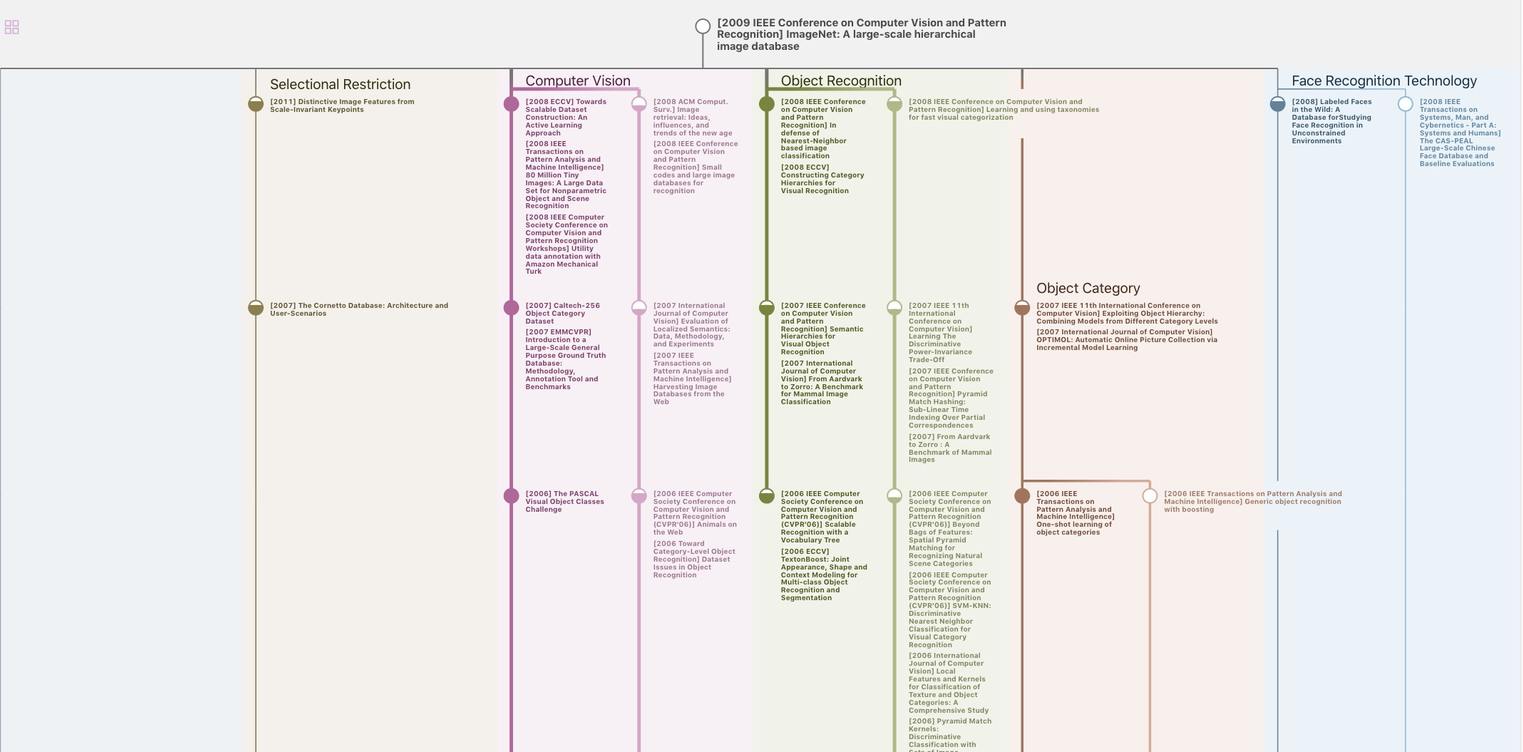
生成溯源树,研究论文发展脉络
Chat Paper
正在生成论文摘要