Super-Resolution of License Plate Images Using Attention Modules and Sub-Pixel Convolution Layers
CoRR(2023)
摘要
Recent years have seen significant developments in the field of License Plate Recognition (LPR) through the integration of deep learning techniques and the increasing availability of training data. Nevertheless, reconstructing license plates (LPs) from low-resolution (LR) surveillance footage remains challenging. To address this issue, we introduce a Single-Image Super-Resolution (SISR) approach that integrates attention and transformer modules to enhance the detection of structural and textural features in LR images. Our approach incorporates sub-pixel convolution layers (also known as PixelShuffle) and a loss function that uses an Optical Character Recognition (OCR) model for feature extraction. We trained the proposed architecture on synthetic images created by applying heavy Gaussian noise to high-resolution LP images from two public datasets, followed by bicubic downsampling. As a result, the generated images have a Structural Similarity Index Measure (SSIM) of less than 0.10. Our results show that our approach for reconstructing these low-resolution synthesized images outperforms existing ones in both quantitative and qualitative measures. Our code is publicly available at https://github.com/valfride/lpr-rsr-ext/
更多查看译文
关键词
license plate images,attention modules,super-resolution,sub-pixel
AI 理解论文
溯源树
样例
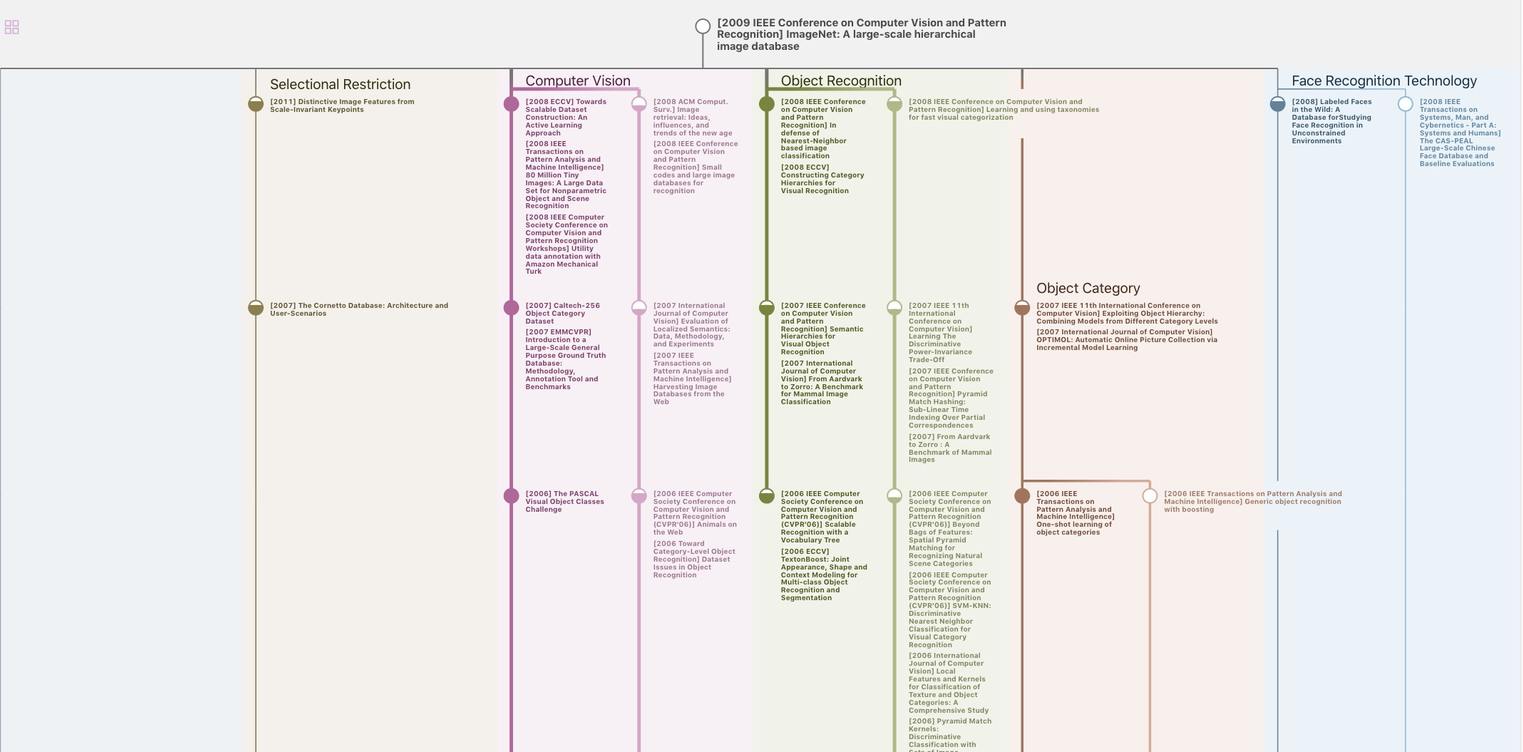
生成溯源树,研究论文发展脉络
Chat Paper
正在生成论文摘要