GVdoc: Graph-based Visual Document Classification
conf_acl(2023)
摘要
The robustness of a model for real-world deployment is decided by how well it performs on unseen data and distinguishes between in-domain and out-of-domain samples. Visual document classifiers have shown impressive performance on in-distribution test sets. However, they tend to have a hard time correctly classifying and differentiating out-of-distribution examples. Image-based classifiers lack the text component, whereas multi-modality transformer-based models face the token serialization problem in visual documents due to their diverse layouts. They also require a lot of computing power during inference, making them impractical for many real-world applications. We propose, GVdoc, a graph-based document classification model that addresses both of these challenges. Our approach generates a document graph based on its layout, and then trains a graph neural network to learn node and graph embeddings. Through experiments, we show that our model, even with fewer parameters, outperforms state-of-the-art models on out-of-distribution data while retaining comparable performance on the in-distribution test set.
更多查看译文
关键词
visual document classification,graph-based
AI 理解论文
溯源树
样例
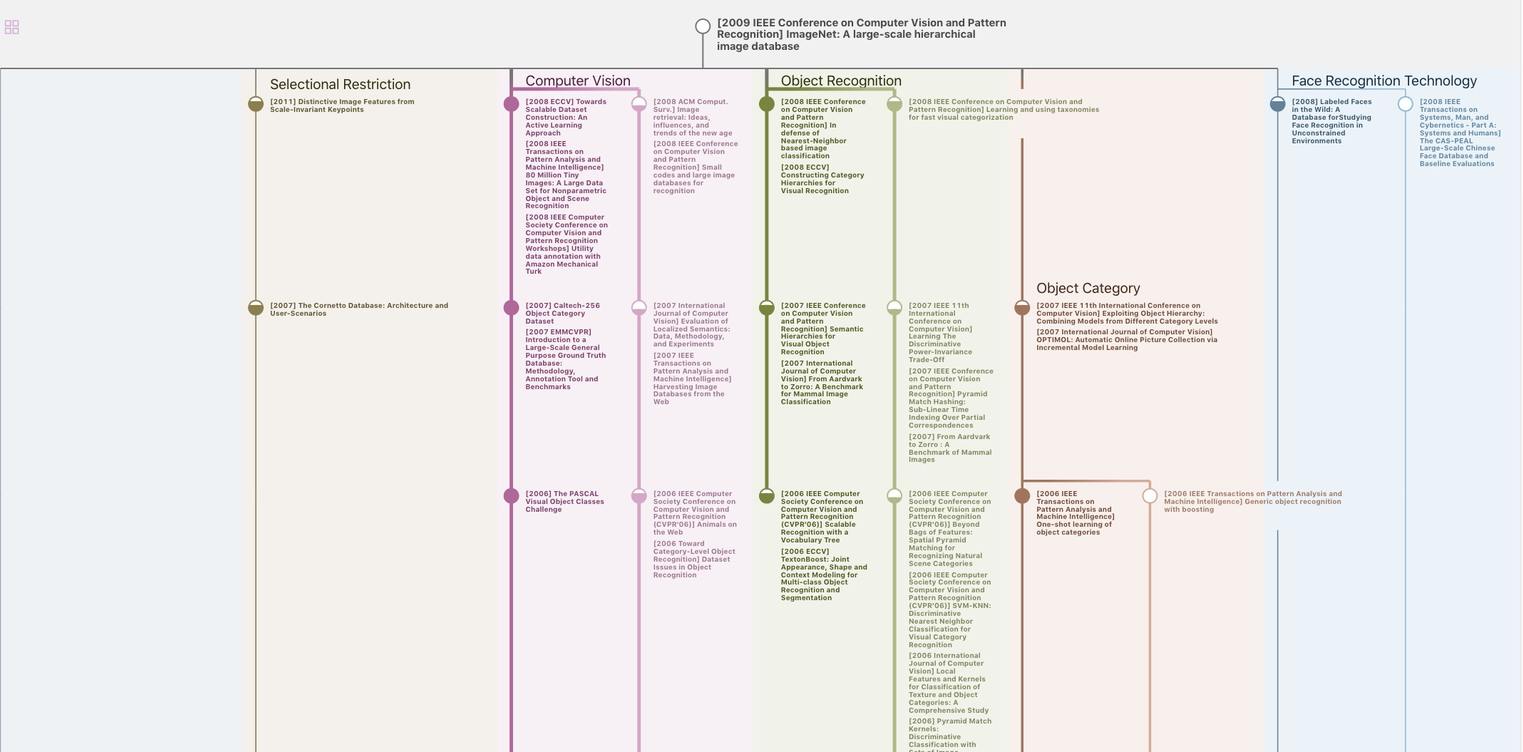
生成溯源树,研究论文发展脉络
Chat Paper
正在生成论文摘要