Entailment as Robust Self-Learner
PROCEEDINGS OF THE 61ST ANNUAL MEETING OF THE ASSOCIATION FOR COMPUTATIONAL LINGUISTICS (ACL 2023): LONG PAPERS, VOL 1(2023)
Abstract
Entailment has been recognized as an important metric for evaluating natural language understanding (NLU) models, and recent studies have found that entailment pretraining benefits weakly supervised fine-tuning. In this work, we design a prompting strategy that formulates a number of different NLU tasks as contextual entailment. This approach improves the zero-shot adaptation of pretrained entailment models. Secondly, we notice that self-training entailment-based models with unlabeled data can significantly improve the adaptation performance on downstream tasks. To achieve more stable improvement, we propose the Simple Pseudo-Label Editing (SimPLE) algorithm for better pseudo-labeling quality in self-training. We also found that both pretrained entailment-based models and the self-trained models are robust against adversarial evaluation data. Experiments on binary and multi-class classification tasks show that SimPLE leads to more robust self-training results, indicating that the self-trained entailment models are more efficient and trustworthy than large language models on language understanding tasks.
MoreTranslated text
Key words
Language Modeling,Topic Modeling,Natural Language Processing,Sequence-to-Sequence Learning,Semantic Simplification
AI Read Science
Must-Reading Tree
Example
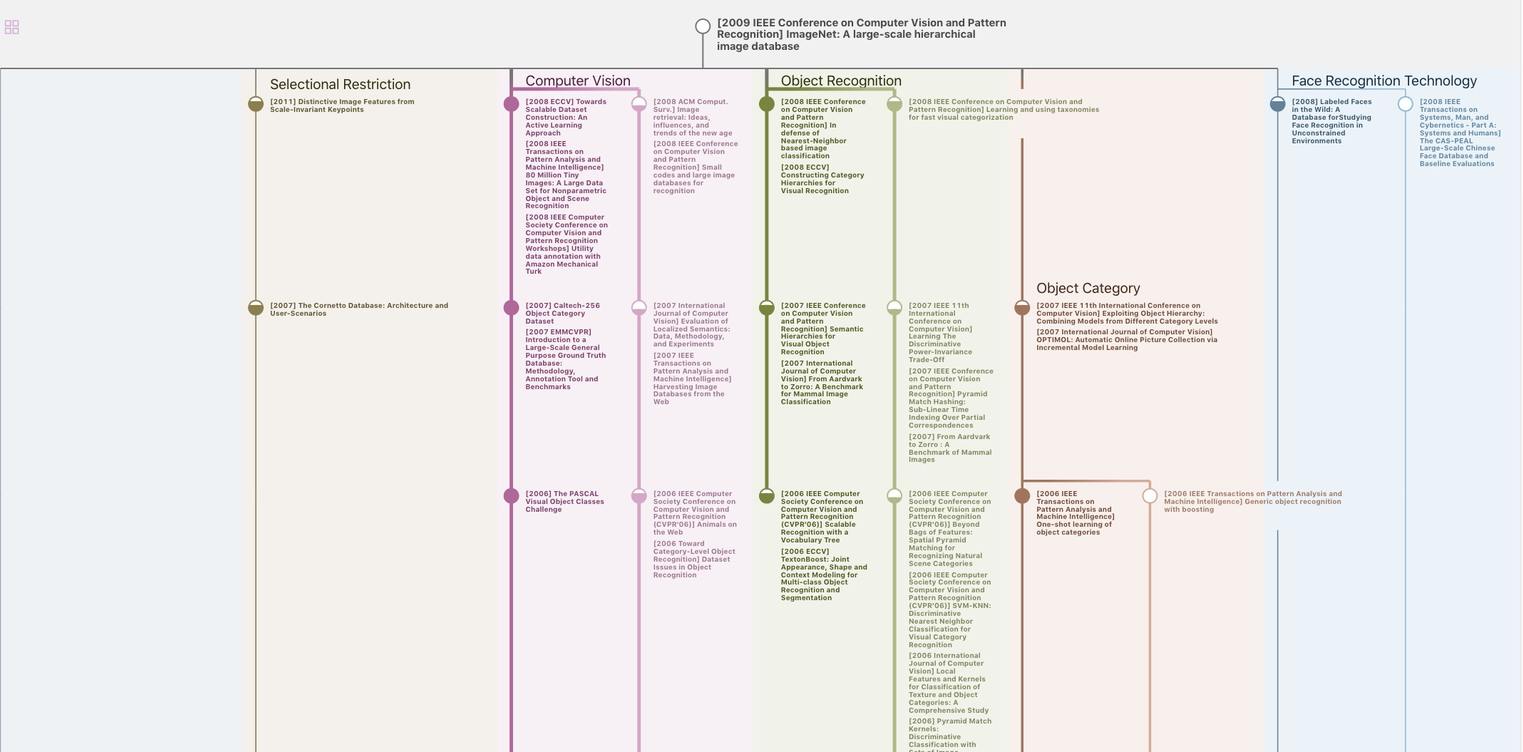
Generate MRT to find the research sequence of this paper
Chat Paper
Summary is being generated by the instructions you defined