No-regret Learning Dynamics for Sequential Correlated Equilibria
AAMAS '23: Proceedings of the 2023 International Conference on Autonomous Agents and Multiagent Systems(2023)
摘要
While no-regret learning procedures that converge to correlated equilibria have long been known to exist for normal form games, their analogue in sequential games remains less clear. We propose the sequential correlated equilibrium, a solution concept that extends the correlated equilibrium to sequential games while also guaranteeing sequential rationality even for mediator recommendations off the path of play. Additionally, we show that any internal regret minimization procedure designed for normal-form games can be efficiently extended to sequential games and use this to design no-regret learning dynamics that converge to the set of sequential correlated equilibria.
更多查看译文
AI 理解论文
溯源树
样例
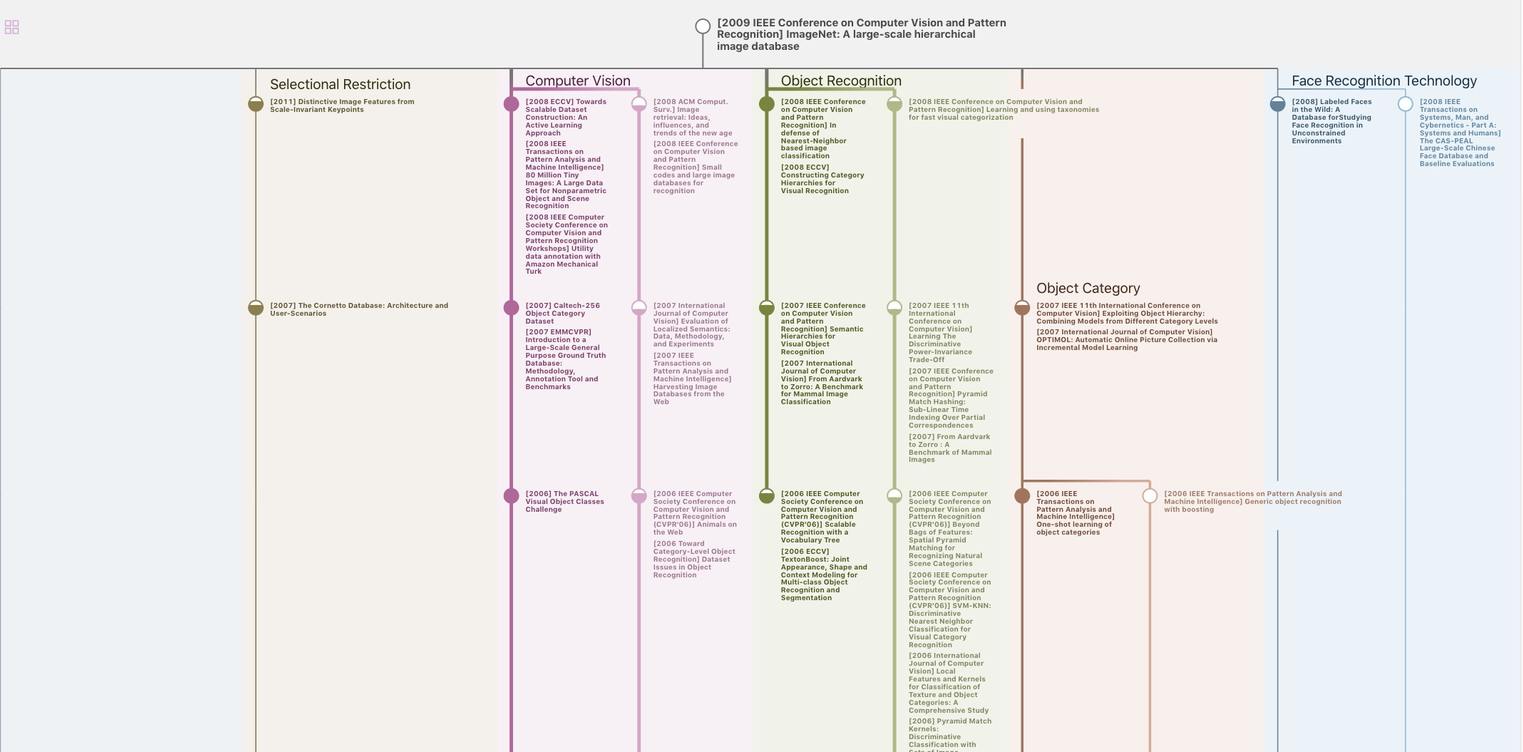
生成溯源树,研究论文发展脉络
Chat Paper
正在生成论文摘要