Defensive Collaborative Learning: Protecting Objective Privacy in Data Sharing
AAMAS '23: Proceedings of the 2023 International Conference on Autonomous Agents and Multiagent Systems(2023)
摘要
In collaborative machine learning, protecting proprietary information and safeguarding competitive advantages are crucial for participating organizations. This necessitates the development of algorithms that target a general notion of privacy defined by the data owner: objective privacy. In this paper, we formalize the idea of objective privacy as the protection of private value propositions characterized by predictive functions. We propose Defensive Collaborative Learning (DCL), where participants share data collaboratively while safeguarding their objective privacy. Formulating a min-max optimization problem that trades off utility and privacy protection, we propose algorithms that leverage mutual information backpropagation in both decentralized and centralized settings. Empirical studies show that the proposed algorithms protect objective privacy while enabling data sharing.
更多查看译文
AI 理解论文
溯源树
样例
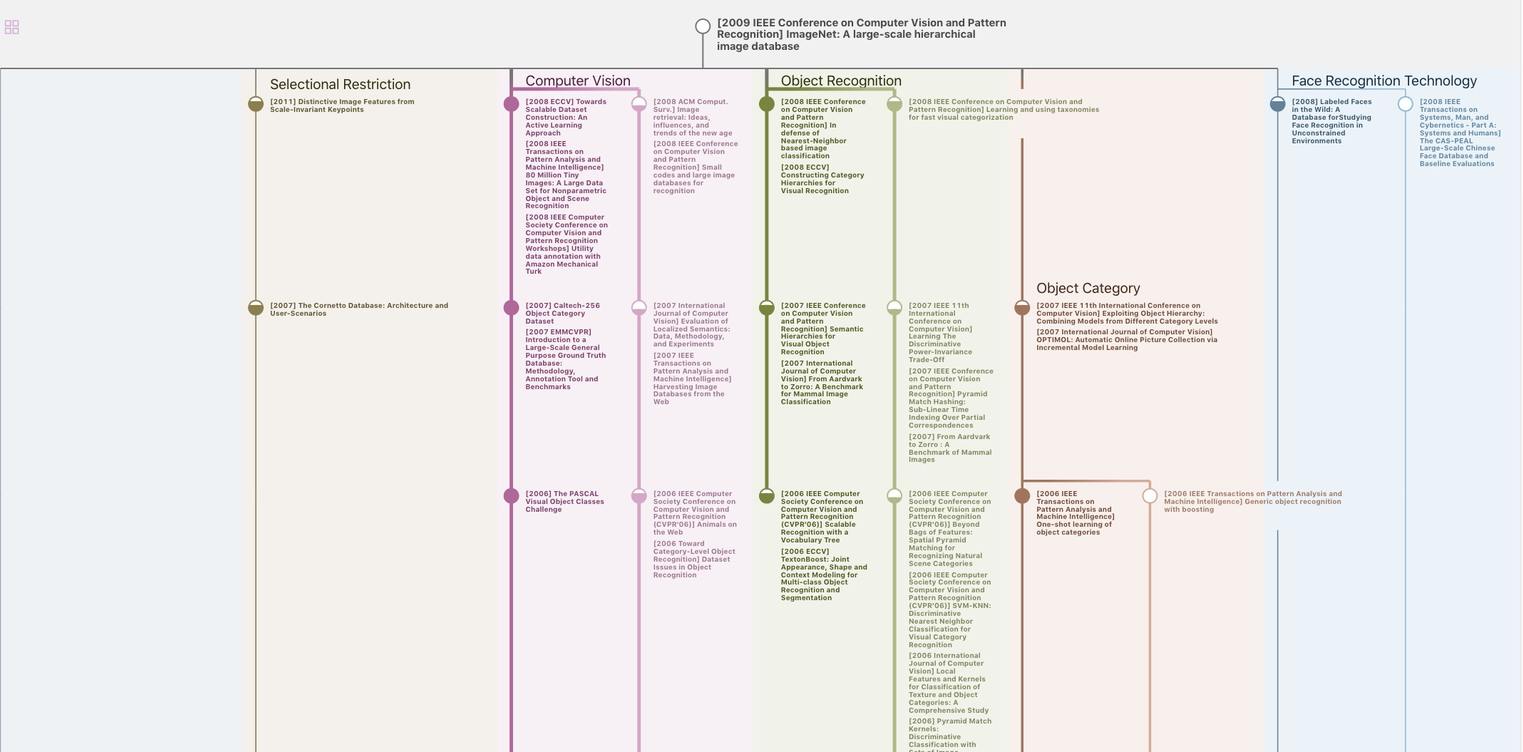
生成溯源树,研究论文发展脉络
Chat Paper
正在生成论文摘要