Signal Property Information-Based Target Detection with Dual-Output Neural Network in Complex Environments.
Sensors(2023)
摘要
The performance of traditional model-based constant false-alarm ratio (CFAR) detection algorithms can suffer in complex environments, particularly in scenarios involving multiple targets (MT) and clutter edges (CE) due to an imprecise estimation of background noise power level. Furthermore, the fixed threshold mechanism that is commonly used in the single-input single-output neural network can result in performance degradation due to changes in the scene. To overcome these challenges and limitations, this paper proposes a novel approach, a single-input dual-output network detector (SIDOND) using data-driven deep neural networks (DNN). One output is used for signal property information (SPI)-based estimation of the detection sufficient statistic, while the other is utilized to establish a dynamic-intelligent threshold mechanism based on the threshold impact factor (TIF), where the TIF is a simplified description of the target and background environment information. Experimental results demonstrate that SIDOND is more robust and performs better than model-based and single-output network detectors. Moreover, the visual explanation technique is employed to explain the working of SIDOND.
更多查看译文
关键词
radar signal processing,target detection,signal property information,dual-output network,dynamic-intelligent threshold
AI 理解论文
溯源树
样例
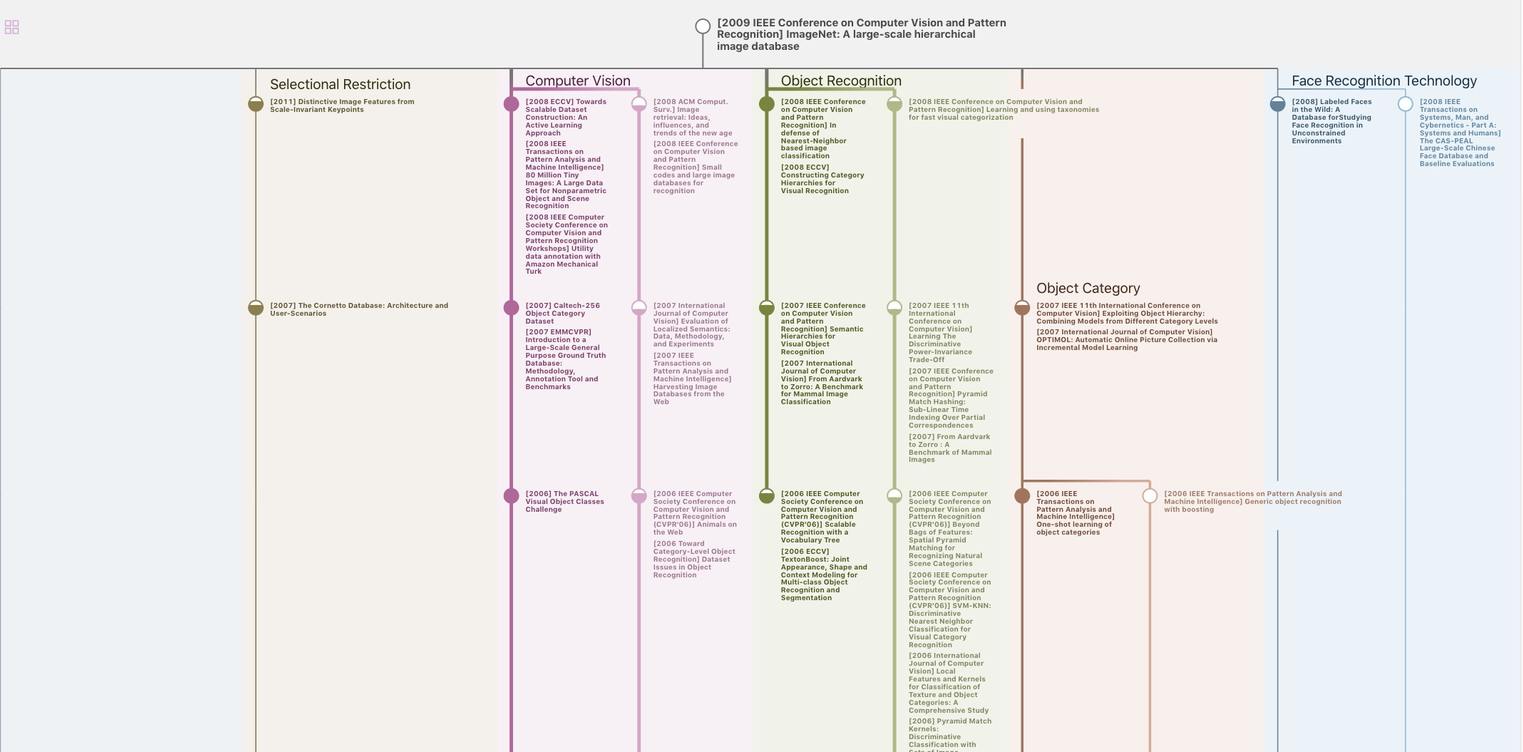
生成溯源树,研究论文发展脉络
Chat Paper
正在生成论文摘要