A Closer Look at In-Context Learning under Distribution Shifts
CoRR(2023)
摘要
In-context learning, a capability that enables a model to learn from input examples on the fly without necessitating weight updates, is a defining characteristic of large language models. In this work, we follow the setting proposed in (Garg et al., 2022) to better understand the generality and limitations of in-context learning from the lens of the simple yet fundamental task of linear regression. The key question we aim to address is: Are transformers more adept than some natural and simpler architectures at performing in-context learning under varying distribution shifts? To compare transformers, we propose to use a simple architecture based on set-based Multi-Layer Perceptrons (MLPs). We find that both transformers and set-based MLPs exhibit in-context learning under in-distribution evaluations, but transformers more closely emulate the performance of ordinary least squares (OLS). Transformers also display better resilience to mild distribution shifts, where set-based MLPs falter. However, under severe distribution shifts, both models' in-context learning abilities diminish.
更多查看译文
关键词
learning,distribution,in-context
AI 理解论文
溯源树
样例
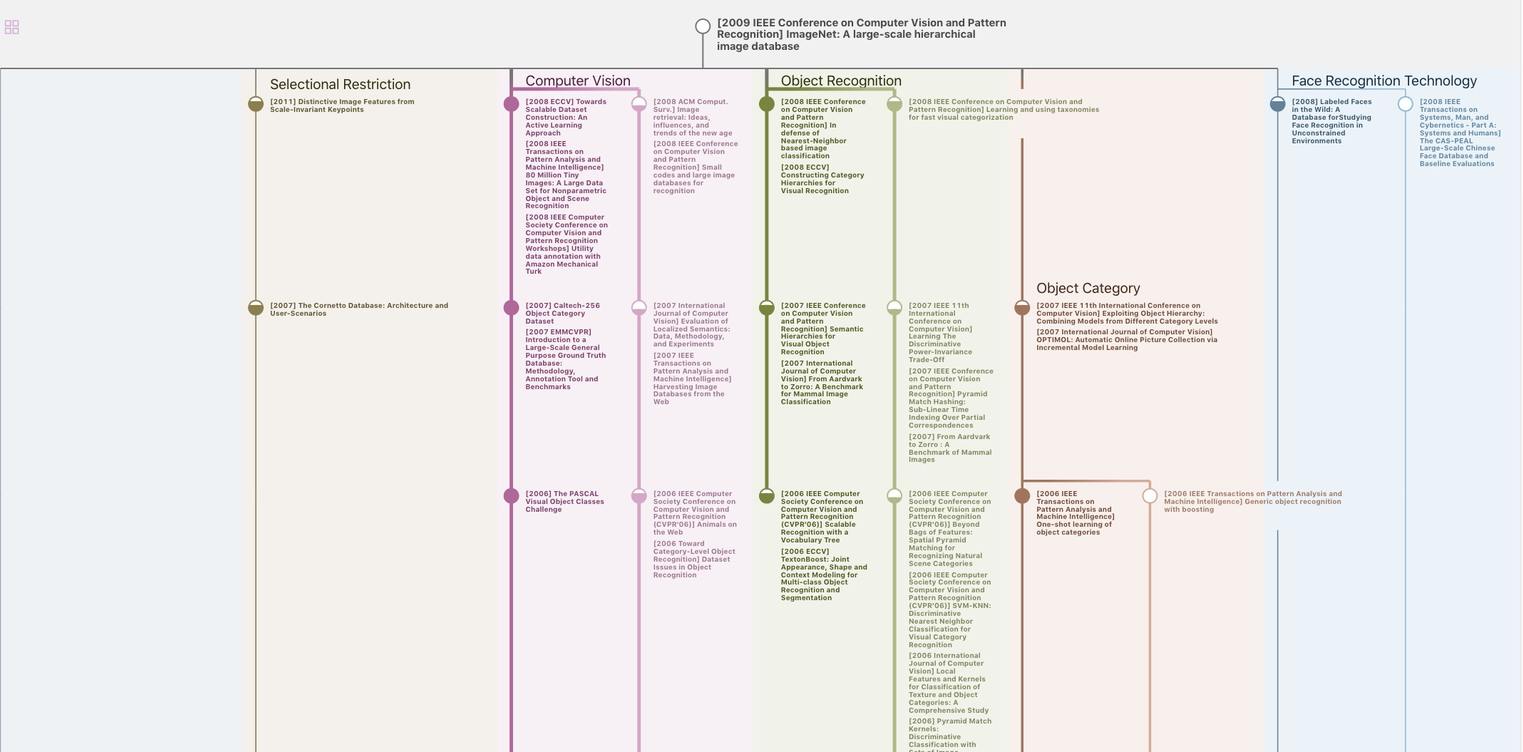
生成溯源树,研究论文发展脉络
Chat Paper
正在生成论文摘要