DKAF: KB Arbitration for Learning Task-Oriented Dialog Systems with Dialog-KB Inconsistencies
conf_acl(2023)
摘要
Task-oriented dialog (TOD) agents often ground their responses on external knowledge bases (KBs). These KBs can be dynamic and may be updated frequently. Existing approaches for learning TOD agents assume the KB snapshot contemporary to each individual dialog is available during training. However, in real-world scenarios, only the latest KB snapshot is available during training and as a result, the train dialogs may contain facts conflicting with the latest KB. These dialog-KB inconsistencies in the training data may potentially confuse the TOD agent learning algorithm. In this work, we define the novel problem of learning a TOD agent with dialog-KB inconsistencies in the training data. We propose a Dialog-KB Arbitration Framework (DKAF) which reduces the dialog-KB inconsistencies by predicting the contemporary KB snapshot for each train dialog. These predicted KB snapshots are then used for training downstream TOD agents. As there are no existing datasets with dialog-KB inconsistencies, we systematically introduce inconsistencies in two publicly available dialog datasets. We show that TOD agents trained with DKAF perform better than existing baselines on both these datasets
更多查看译文
关键词
dialog systems,kb arbitration,task-oriented
AI 理解论文
溯源树
样例
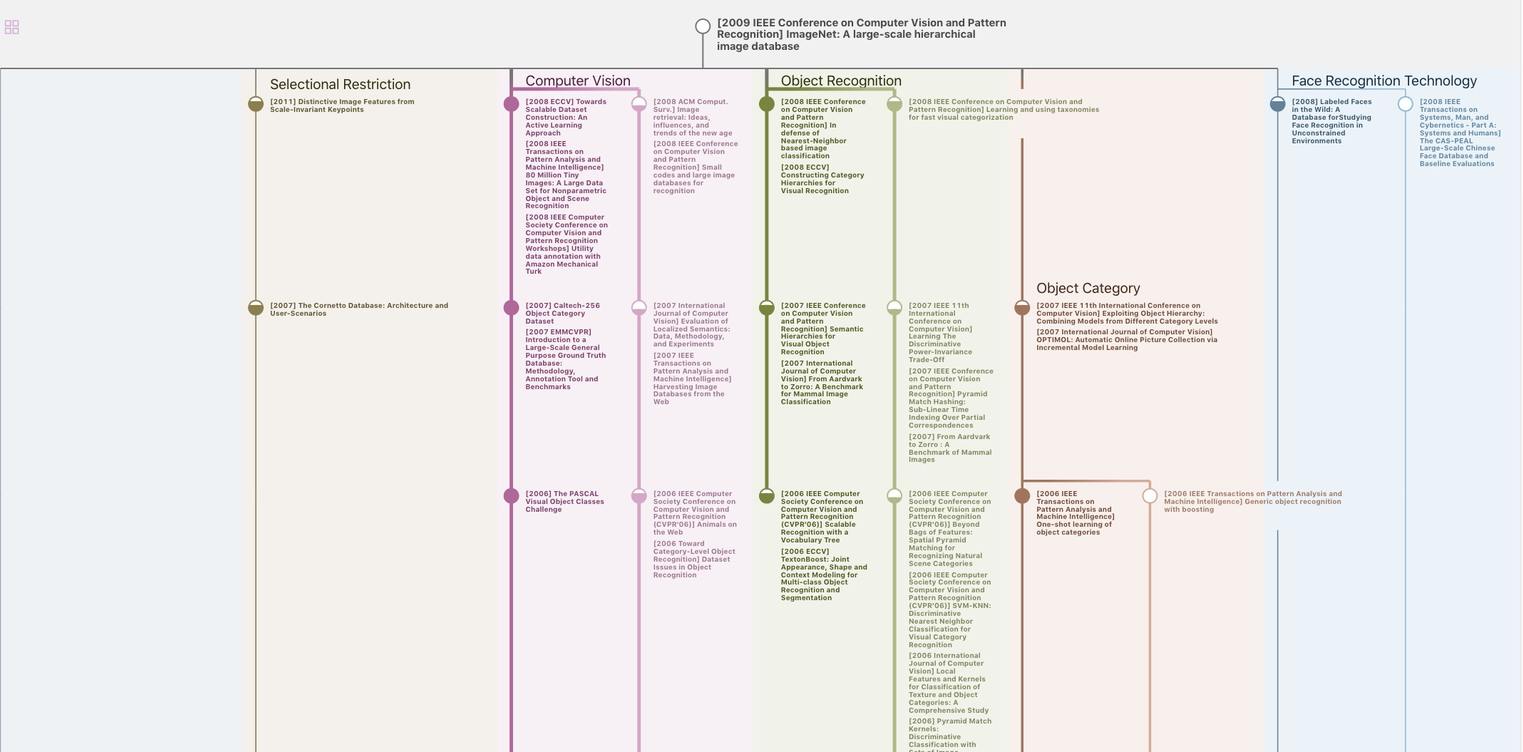
生成溯源树,研究论文发展脉络
Chat Paper
正在生成论文摘要