A Unified Approach for Maximizing Continuous DR-submodular Functions
NeurIPS(2023)
摘要
This paper presents a unified approach for maximizing continuous DR-submodular functions that encompasses a range of settings and oracle access types. Our approach includes a Frank-Wolfe type offline algorithm for both monotone and non-monotone functions, with different restrictions on the general convex set. We consider settings where the oracle provides access to either the gradient of the function or only the function value, and where the oracle access is either deterministic or stochastic. We determine the number of required oracle accesses in all cases. Our approach gives new/improved results for nine out of the sixteen considered cases, avoids computationally expensive projections in two cases, with the proposed framework matching performance of state-of-the-art approaches in the remaining five cases. Notably, our approach for the stochastic function value-based oracle enables the first regret bounds with bandit feedback for stochastic DR-submodular functions.
更多查看译文
关键词
functions,unified approach,dr-submodular
AI 理解论文
溯源树
样例
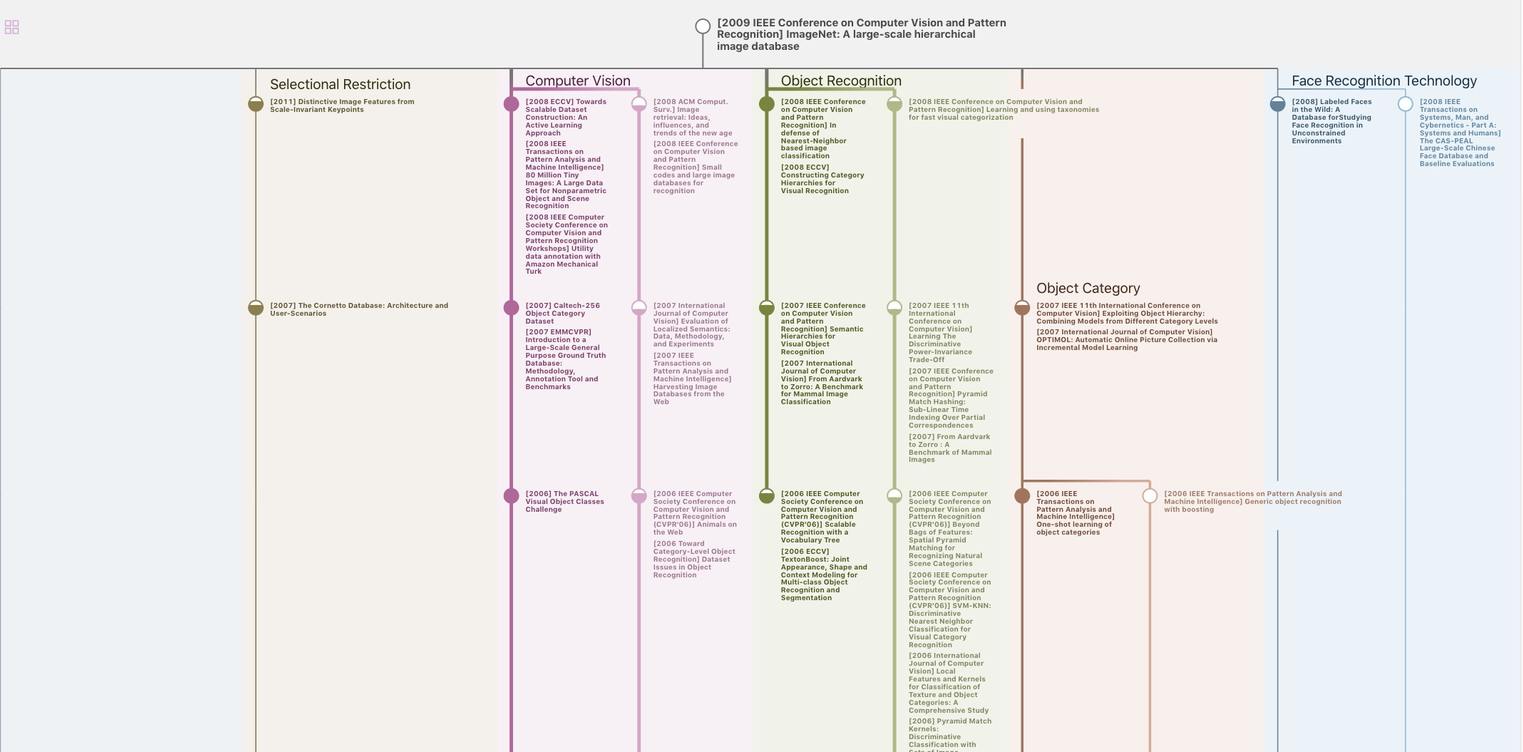
生成溯源树,研究论文发展脉络
Chat Paper
正在生成论文摘要