Physical Deep Reinforcement Learning: Safety and Unknown Unknowns
CoRR(2023)
摘要
In this paper, we propose the Phy-DRL: a physics-model-regulated deep reinforcement learning framework for safety-critical autonomous systems. The Phy-DRL is unique in three innovations: i) proactive unknown-unknowns training, ii) conjunctive residual control (i.e., integration of data-driven control and physics-model-based control) and safety- \& stability-sensitive reward, and iii) physics-model-based neural network editing, including link editing and activation editing. Thanks to the concurrent designs, the Phy-DRL is able to 1) tolerate unknown-unknowns disturbances, 2) guarantee mathematically provable safety and stability, and 3) strictly comply with physical knowledge pertaining to Bellman equation and reward. The effectiveness of the Phy-DRL is finally validated by an inverted pendulum and a quadruped robot. The experimental results demonstrate that compared with purely data-driven DRL, Phy-DRL features remarkably fewer learning parameters, accelerated training and enlarged reward, while offering enhanced model robustness and safety assurance.
更多查看译文
关键词
deep reinforcement learning,safety,physical
AI 理解论文
溯源树
样例
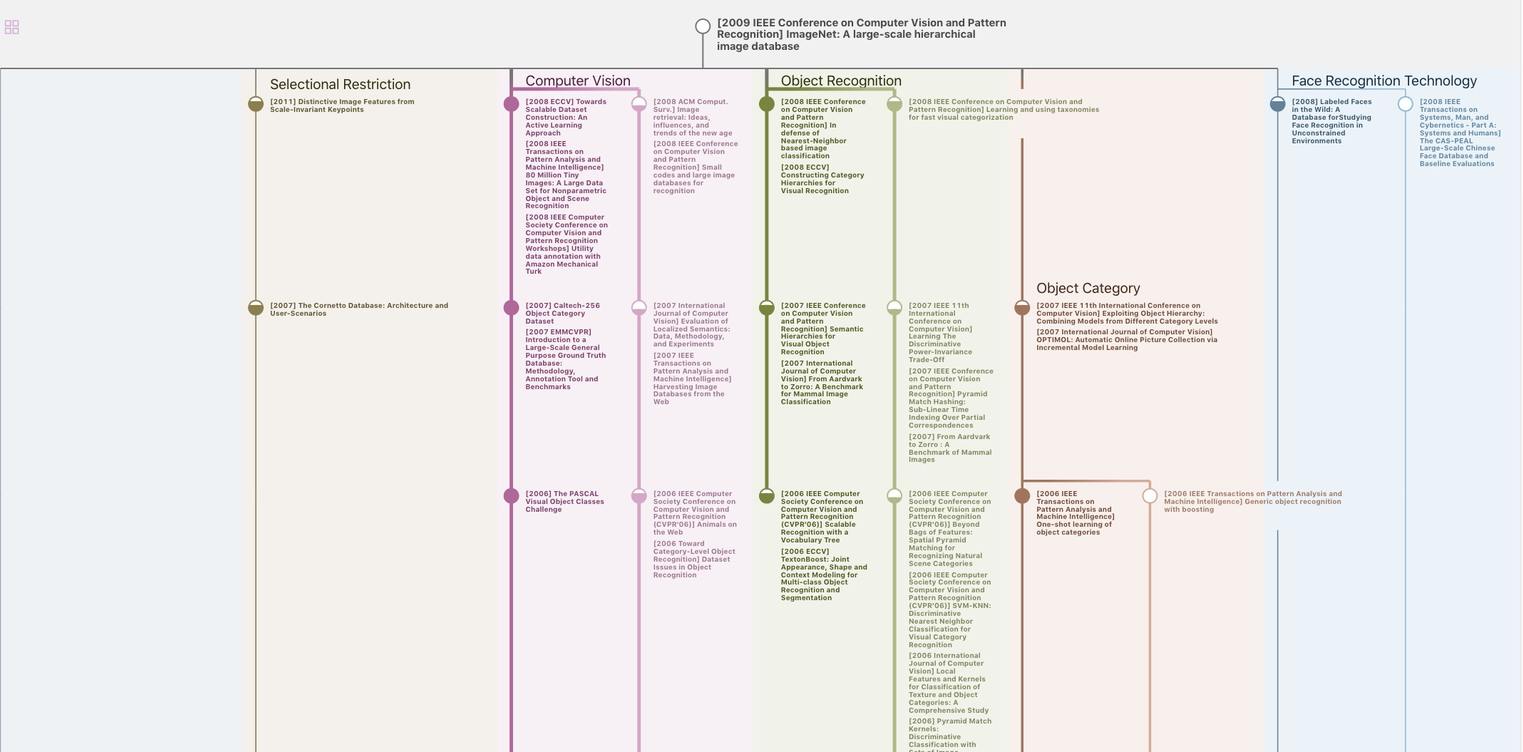
生成溯源树,研究论文发展脉络
Chat Paper
正在生成论文摘要