Neural Architecture Search for Parameter-Efficient Fine-tuning of Large Pre-trained Language Models
conf_acl(2023)
Abstract
Parameter-efficient tuning (PET) methods fit pre-trained language models (PLMs) to downstream tasks by either computing a small compressed update for a subset of model parameters, or appending and fine-tuning a small number of new model parameters to the pre-trained network. Hand-designed PET architectures from the literature perform well in practice, but have the potential to be improved via automated neural architecture search (NAS). We propose an efficient NAS method for learning PET architectures via structured and unstructured pruning. We present experiments on GLUE demonstrating the effectiveness of our algorithm and discuss how PET architectural design choices affect performance in practice.
MoreTranslated text
Key words
models,language,search,parameter-efficient,fine-tuning,pre-trained
AI Read Science
Must-Reading Tree
Example
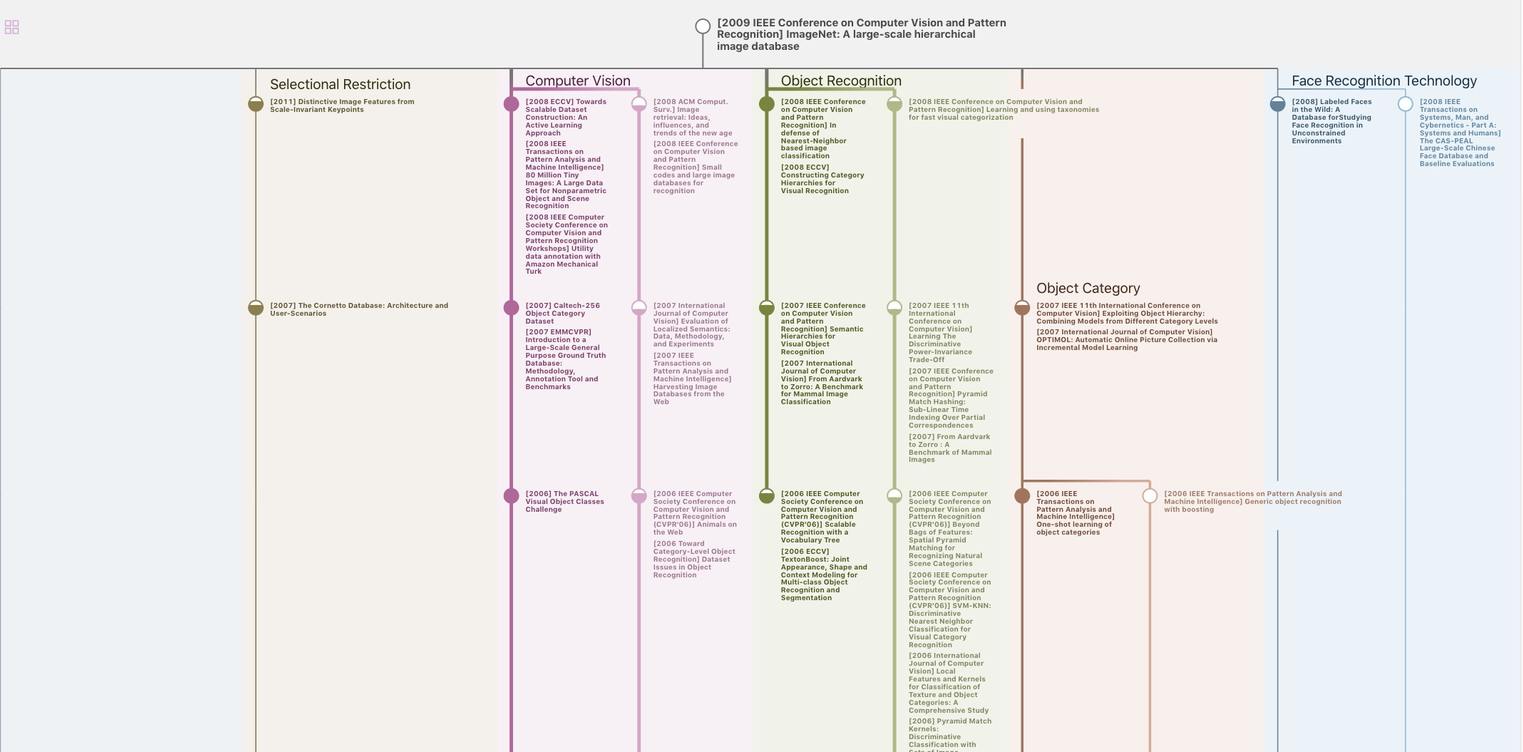
Generate MRT to find the research sequence of this paper
Chat Paper
Summary is being generated by the instructions you defined