INTapt: Information-Theoretic Adversarial Prompt Tuning for Enhanced Non-Native Speech Recognition
conf_acl(2023)
摘要
Automatic Speech Recognition (ASR) systems have attained unprecedented performance with large speech models pre-trained based on self-supervised speech representation learning. However, these pre-trained speech models suffer from representational bias as they tend to better represent those prominent accents (i.e., native (L1) English accent) in the pre-training speech corpus than less represented accents, resulting in a deteriorated performance for non-native (L2) English accents. Although there have been some approaches to mitigate this issue, all of these methods require updating the pre-trained model weights. In this paper, we propose Information Theoretic Adversarial Prompt Tuning (INTapt), which introduces prompts concatenated to the original input that can re-modulate the attention of the pre-trained model such that the corresponding input resembles a native (L1) English speech without updating the backbone weights. INTapt is trained simultaneously in the following two manners: (1) adversarial training to reduce accent feature dependence between the original input and the prompt-concatenated input and (2) training to minimize CTC loss for improving ASR performance to a prompt-concatenated input. Experimental results show that INTapt improves the performance of L2 English and increases feature similarity between L2 and L1 accents.
更多查看译文
关键词
speech,recognition,information-theoretic,non-native
AI 理解论文
溯源树
样例
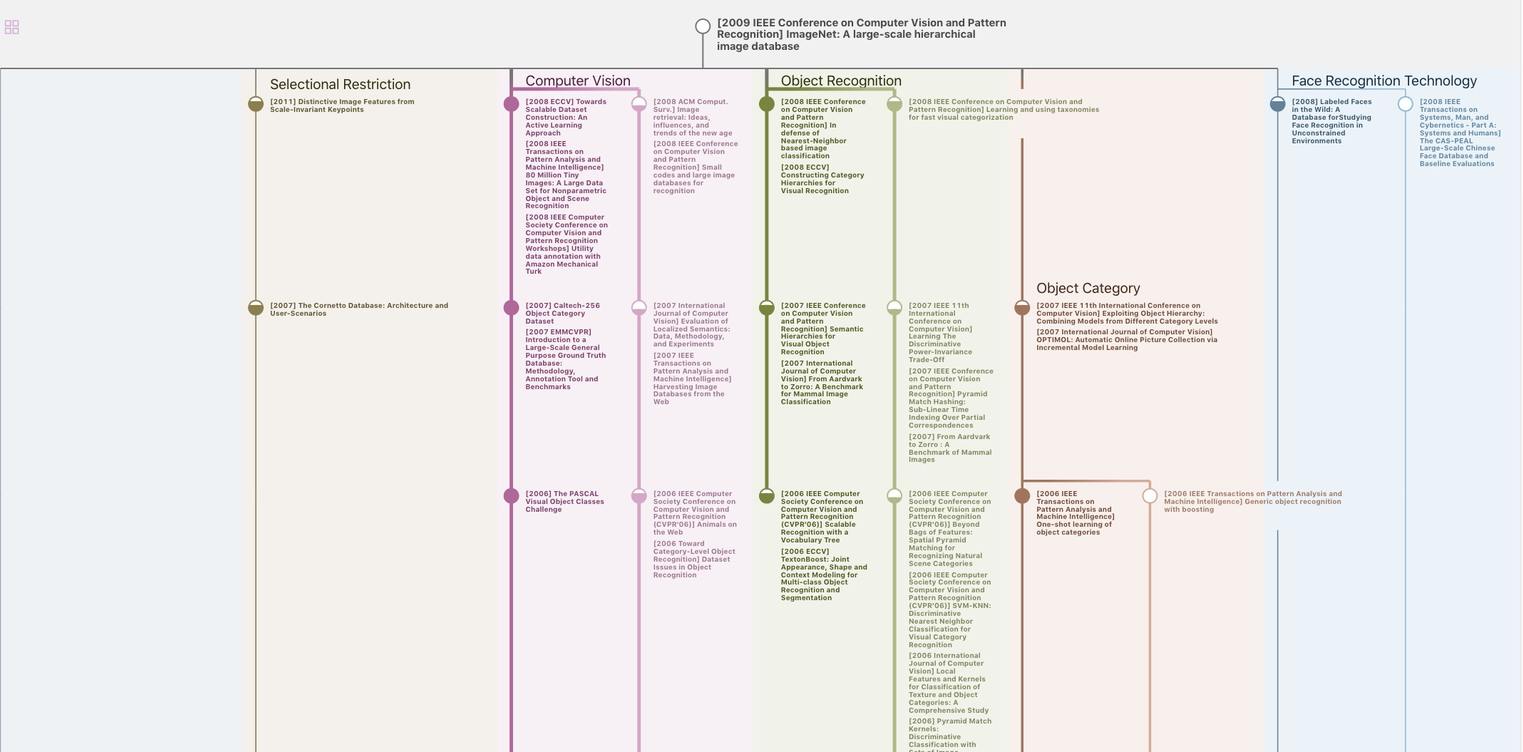
生成溯源树,研究论文发展脉络
Chat Paper
正在生成论文摘要