Think Before You Act: Decision Transformers with Internal Working Memory
CoRR(2023)
摘要
Large language model (LLM)-based decision-making agents have shown the ability to generalize across multiple tasks. However, their performance relies on massive data and compute. We argue that this inefficiency stems from the forgetting phenomenon, in which a model memorizes its behaviors in parameters throughout training. As a result, training on a new task may deteriorate the model's performance on previous tasks. In contrast to LLMs' implicit memory mechanism, the human brain utilizes distributed memory storage, which helps manage and organize multiple skills efficiently, mitigating the forgetting phenomenon. Thus inspired, we propose an internal working memory module to store, blend, and retrieve information for different downstream tasks. Evaluation results show that the proposed method improves training efficiency and generalization in both Atari games and meta-world object manipulation tasks. Moreover, we demonstrate that memory fine-tuning further enhances the adaptability of the proposed architecture.
更多查看译文
关键词
working memory,decision transformers,act,think
AI 理解论文
溯源树
样例
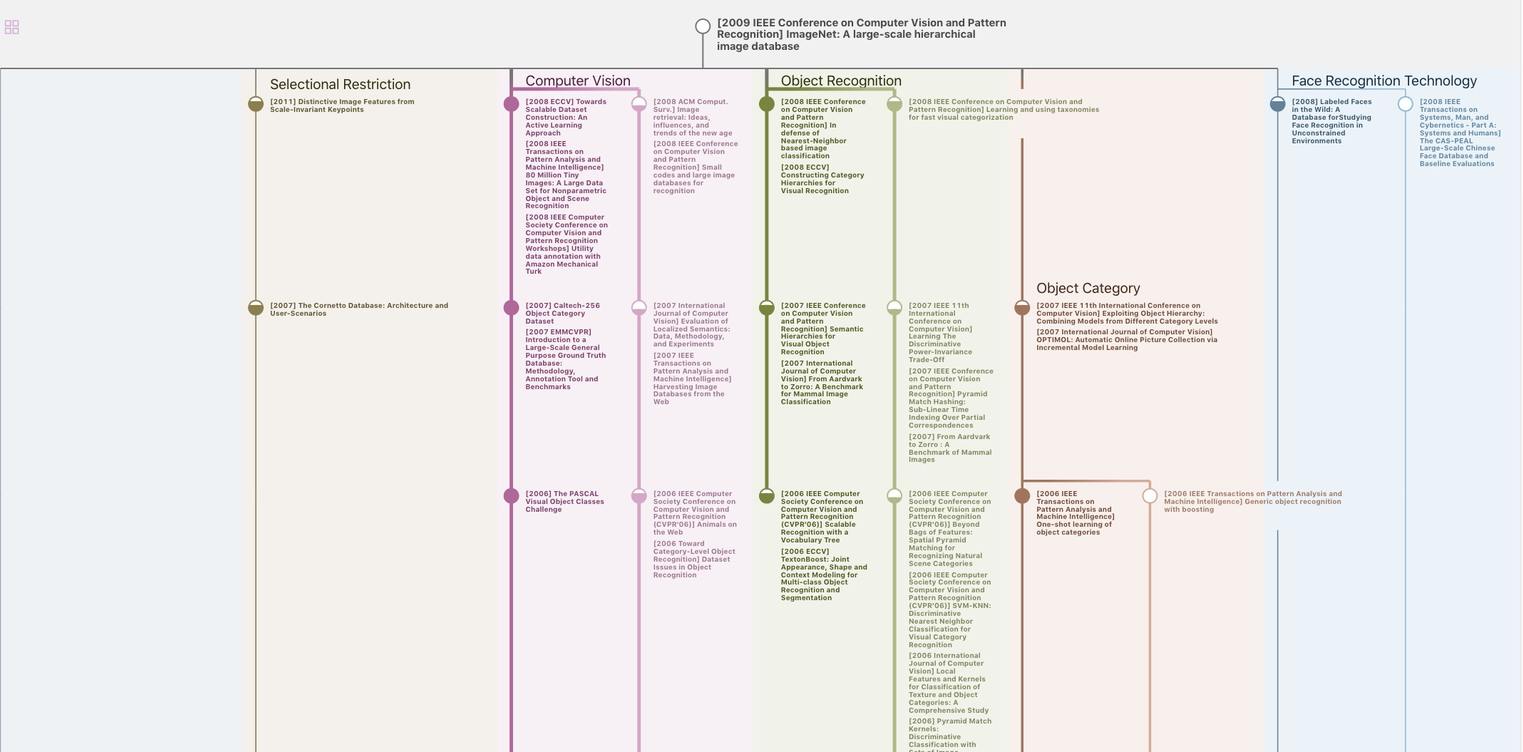
生成溯源树,研究论文发展脉络
Chat Paper
正在生成论文摘要