ImageFed: Practical Privacy Preserving Intrusion Detection System for In-Vehicle CAN Bus Protocol
2023 IEEE 9th Intl Conference on Big Data Security on Cloud (BigDataSecurity), IEEE Intl Conference on High Performance and Smart Computing, (HPSC) and IEEE Intl Conference on Intelligent Data and Security (IDS)(2023)
摘要
In-vehicle networks handle the communication among Electronic Control Units (ECUs) and sensors with a serial protocol called Controller Area Network (CAN), which takes advantage of a broadcast mechanism for transferring data. However, the lack of authentication and encryption in CAN bus might lead to the vehicle being compromised by an untrusted agent. Meanwhile, AI-based Network Intrusion Detection Systems (NIDS) introduced in the literature require a centralized cloud server to undertake the resource-consuming task of processing network data, which also occupies the bandwidth while transferring data from vehicles to the remote server. Moreover, many car manufacturers avoid sharing information with the remote server to prevent further exposure of vehicles’ private data. We utilize Federated Learning (FL) as a suitable solution to previous challenges, where data is trained locally on the vehicle side, and partially trained models are sent to the server. Nevertheless, private training in-side the vehicle requires low memory utilization while achieving low latency and high performance compared to Centralized Learning (CL) approaches. In this study, we propose a practical privacy-preserving IDS (ImageFed) approach by employing federated Convolutional Neural Network (CNN). To evaluate the robustness of ImageFed in real-world applications, we investigate two scenarios that may cause performance degradation in FL, such as non-independent and identically distributed (non-iid) clients and scarcity of training data during the process. The resulting analysis proves the robustness of ImageFed while delivering an average 99.54% f1-score, 99.87% accuracy, and low detection latency.
更多查看译文
关键词
Terms Internet of Vehicles (IoV), Security, Intrusion Detection Systems (IDS), Federated Learning (FL), I)eep Learning (DL), Convolutional Neural Networks (CNN)
AI 理解论文
溯源树
样例
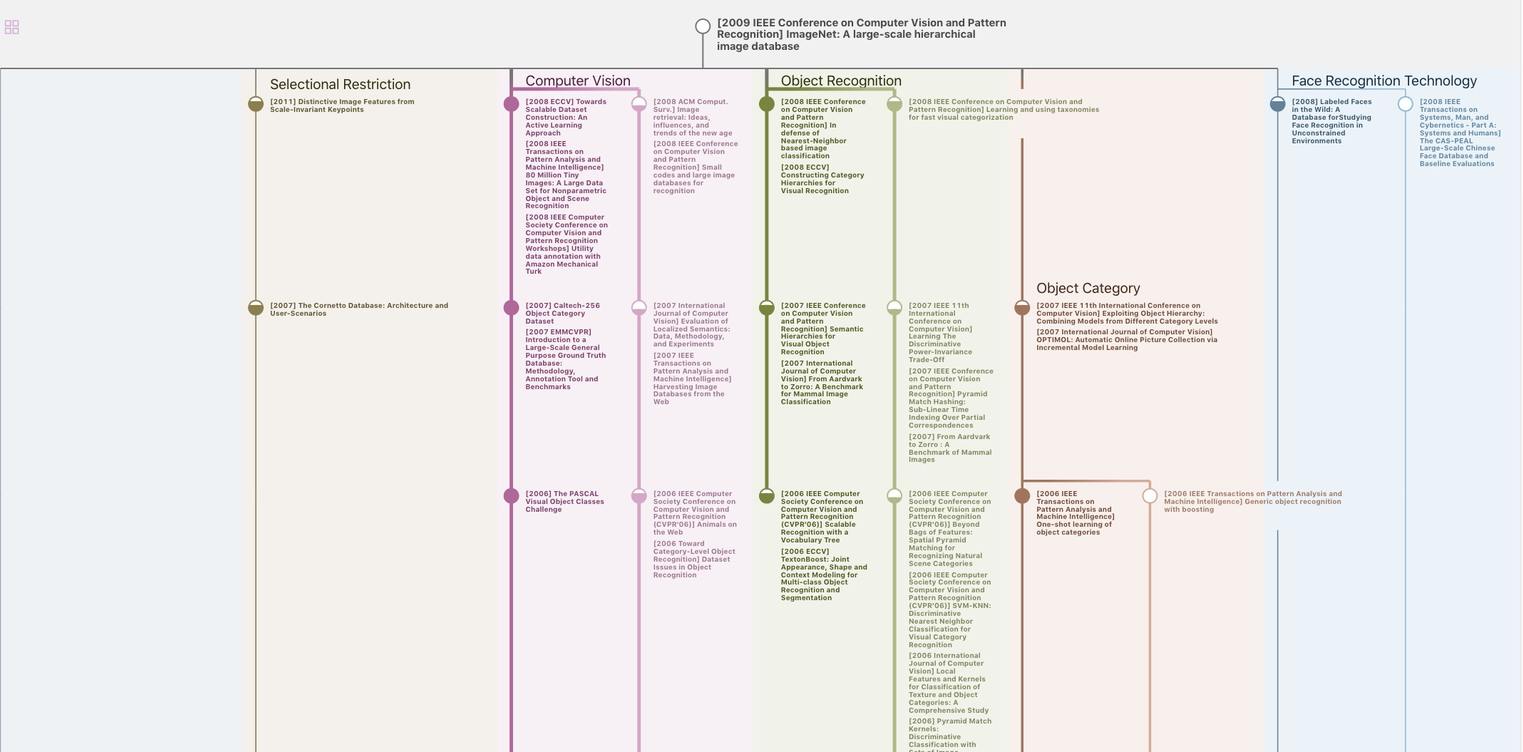
生成溯源树,研究论文发展脉络
Chat Paper
正在生成论文摘要