2MiCo: A Contrastive Semi-Supervised Method with Double Mixup for Smart Meter Modbus RS-485 Communication Security
2023 IEEE 9th Intl Conference on Big Data Security on Cloud (BigDataSecurity), IEEE Intl Conference on High Performance and Smart Computing, (HPSC) and IEEE Intl Conference on Intelligent Data and Security (IDS)(2023)
摘要
Industrial control systems (ICSs) are getting integrated into cyber-physical systems (CPSs) for a smarter and more energy-efficient society. As they organize the infrastructure of our modern society, cyber-attacks on ICSs may lead to catastrophic disasters if there are no protection, detection, and mitigation schemes. Modbus / RS-485 networks work as the backbone at the edges of ICSs, which security monitoring data on the communication signals comes with tabular forms and only with a few ground truth labels in the practical scenario, which makes the detection of attacks challenging with legacy machine learning. This paper presents a new approach called 2MiCo (Double Mixup and Contrastive) for semi-supervised learning in tabular security data sets. These data sets pose unique challenges for machine learning due to the loss of contextual information and imbalanced data. We address these challenges with a triplet mixup data augmentation approach in the input layer and a common mixup in the hidden layer. Our approach achieves state-of-the-art performance on both binary and multi-class data sets in the Modbus Attack DataSet for AMI (MAMI) compared to other methods. While semi-supervised methods are well-studied in image and language domains, they have been underutilized in security domains, particularly in tabular data sets. 2MiCo demonstrates promise in addressing these challenges and improving the performance of machine learning in security domains.
更多查看译文
关键词
contrastive learning, semi -supervised method, attack detection, multi-class classification, industrial control system, cybersecurity
AI 理解论文
溯源树
样例
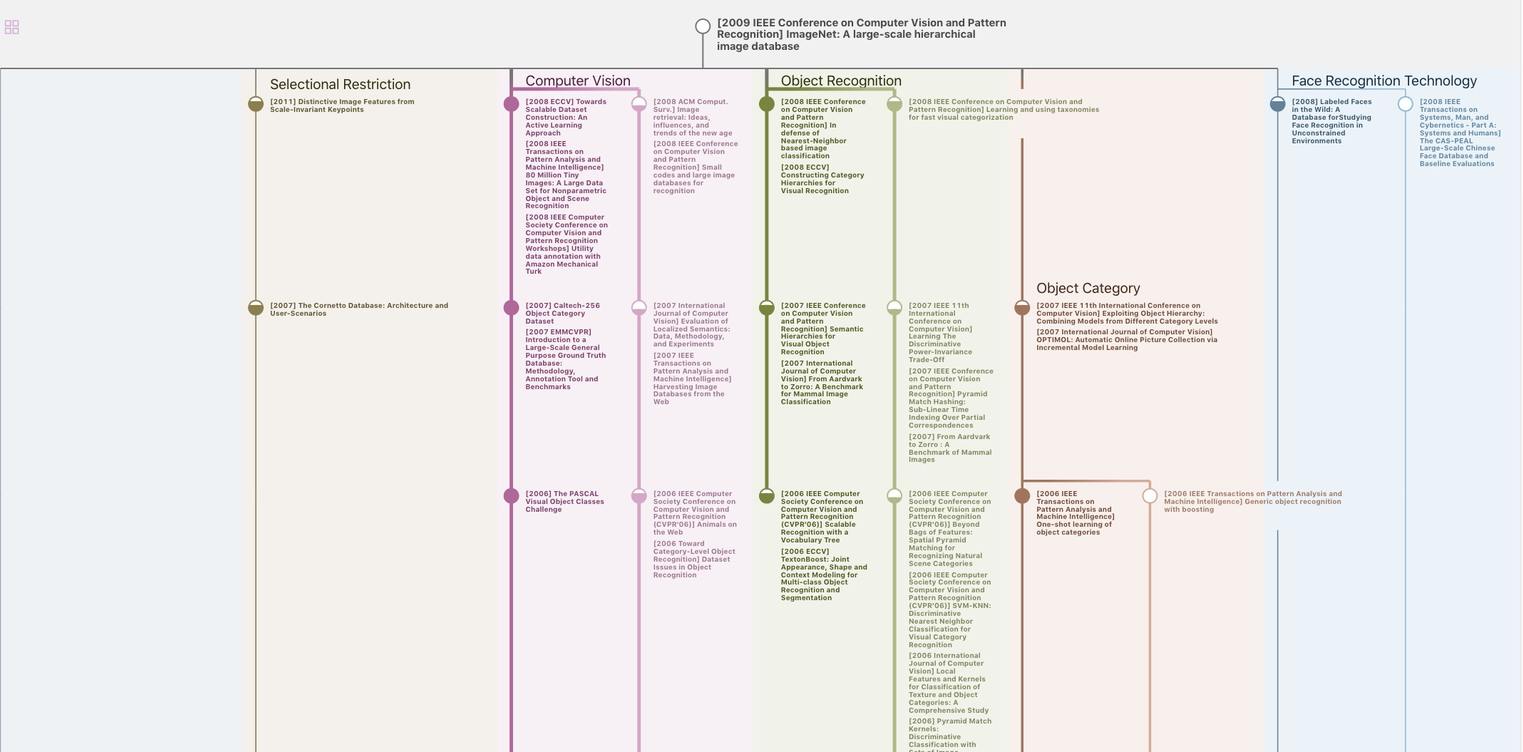
生成溯源树,研究论文发展脉络
Chat Paper
正在生成论文摘要