Chromatic fusion: Generative multimodal neuroimaging data fusion provides multi-informed insights into schizophrenia
HUMAN BRAIN MAPPING(2023)
摘要
This work proposes a novel generative multimodal approach to jointly analyze multimodal data while linking the multimodal information to colors. We apply our proposed framework, which disentangles multimodal data into private and shared sets of features from pairs of structural (sMRI), functional (sFNC and ICA), and diffusion MRI data (FA maps). With our approach, we find that heterogeneity in schizophrenia is potentially a function of modality pairs. Results show (1) schizophrenia is highly multimodal and includes changes in specific networks, (2) non-linear relationships with schizophrenia are observed when interpolating among shared latent dimensions, and (3) we observe a decrease in the modularity of functional connectivity and decreased visual-sensorimotor connectivity for schizophrenia patients for the FA-sFNC and sMRI-sFNC modality pairs, respectively. Additionally, our results generally indicate decreased fractional corpus callosum anisotropy, and decreased spatial ICA map and voxel-based morphometry strength in the superior frontal lobe as found in the FA-sFNC, sMRI-FA, and sMRI-ICA modality pair clusters. In sum, we introduce a powerful new multimodal neuroimaging framework designed to provide a rich and intuitive understanding of the data which we hope challenges the reader to think differently about how modalities interact.
更多查看译文
关键词
deep learning,multimodal fusion,static functional connectivity,structural/functional/diffusion magnetic resonance imaging,variational autoencoders,visualization
AI 理解论文
溯源树
样例
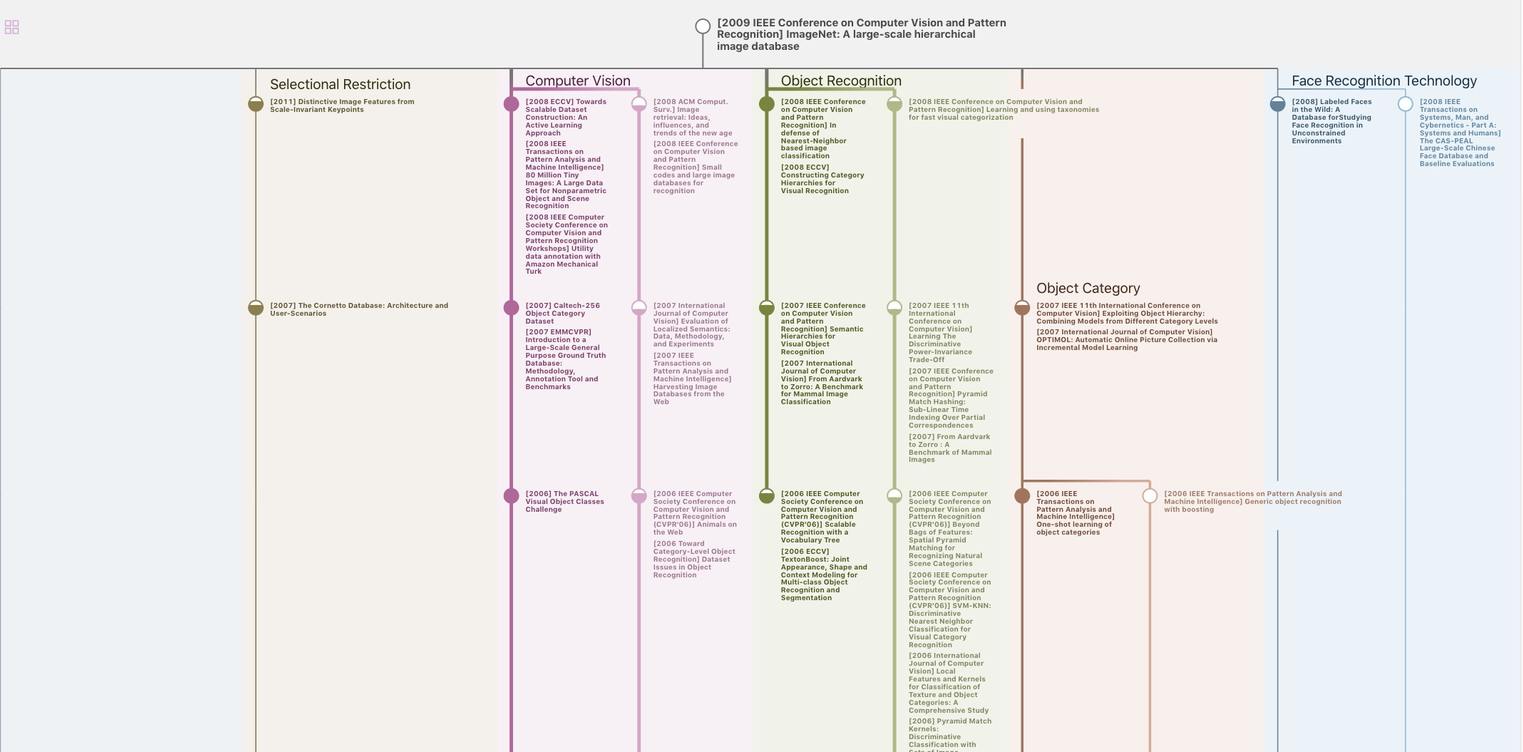
生成溯源树,研究论文发展脉络
Chat Paper
正在生成论文摘要